A framework for data-driven analysis of materials under uncertainty: breaking the curse of dimensionality
semanticscholar(2016)
摘要
A computational framework is developed to assist in the discovery of new material systems and models. The framework integrates three general steps: 1) design of experiments, where the input variables describing material geometry (microstructure), phase properties and external conditions are sampled; 2) efficient computational analyses of each design sample, leading to the creation of a material response database; and 3) machine learning applied to this database to obtain a new design or response model. The choice of methods for the design of experiments, computational analyses, and machine learning steps dictates if the problem of interest is solved within a reasonable time frame. A particular challenge arises when the construction of large databases is needed but the computational analysis of each design sample is too time consuming given reasonable accuracy requirements. This is especially true when nonlinear irreversible processes are modeled (e.g. plasticity). A recently developed reduced order model called “self-consistent clustering analysis” offers a solution to accelerate the material behavior predictions for such cases, enabling the application of the computational framework to a wide range of problems, effectively addressing the curse of dimensionality. The future application of this computational framework to experimentally-validated predictions will lead to the discovery of innovative materials and structures with new capabilities in an era of high-throughput computing (“big-data”).
更多查看译文
AI 理解论文
溯源树
样例
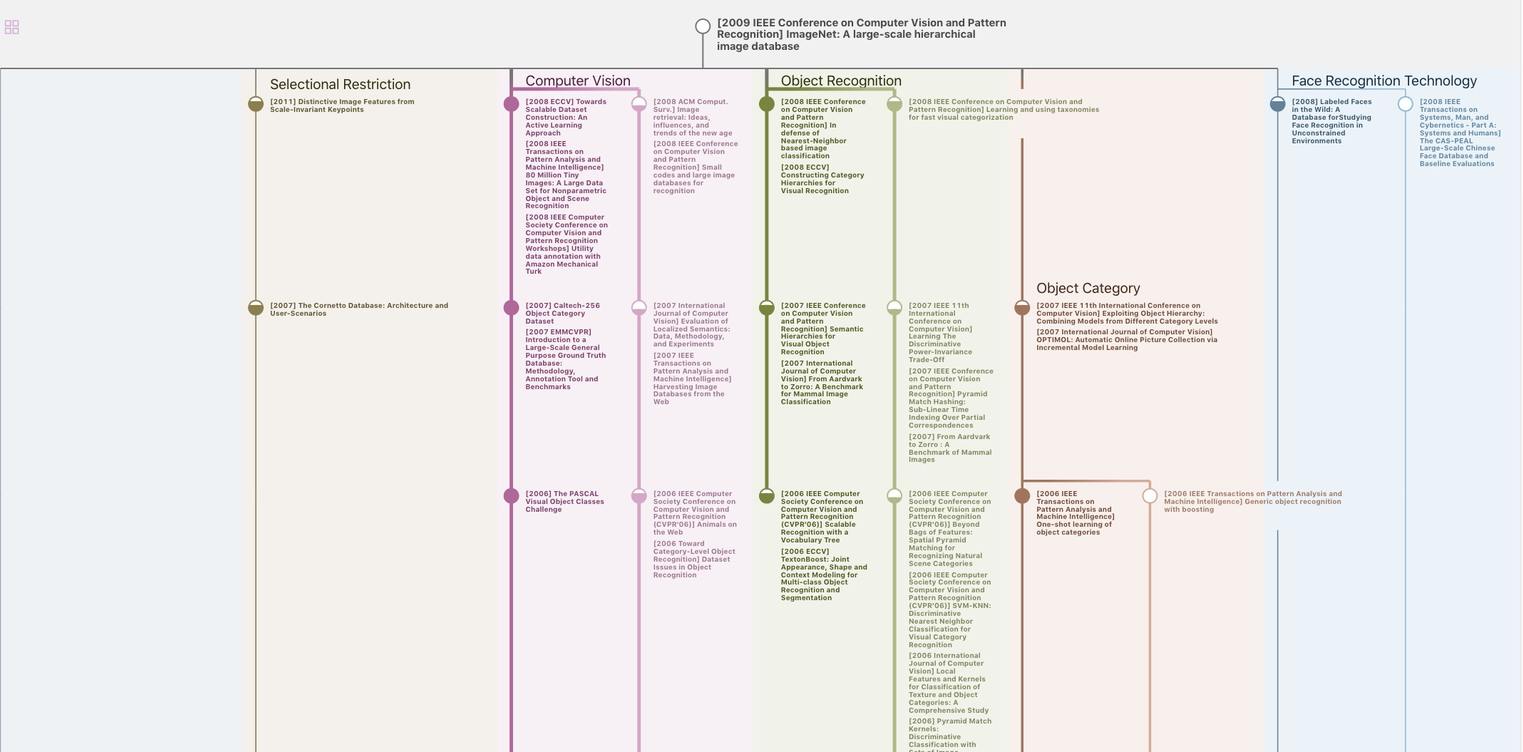
生成溯源树,研究论文发展脉络
Chat Paper
正在生成论文摘要