Spectral-designed Depthwise Separable Graph Neural Networks
semanticscholar(2020)
摘要
This paper aims at revisiting Convolutional Graph Neural Networks (ConvGNNs) by designing new graph convolutions in spectral domain with a custom frequency profile while applying them in the spatial domain. Within the proposed framework, we propose two ConvGNNs methods: one using a simple single-convolution kernel that operates as a low-pass filter, and one operating multiple convolution kernels called Depthwise Separable Graph Convolution Network (DSGCN). The latter is a generalization of the depthwise separable convolution framework for graph convolutional networks, which allows to decrease the total number of trainable parameters while keeping the capacity of the model unchanged. Our proposals are evaluated on both transductive and inductive graph learning problems, demonstrating that DSGCN outperforms the state-of-the-art methods.
更多查看译文
关键词
separable graph,networks,depthwise,spectral-designed
AI 理解论文
溯源树
样例
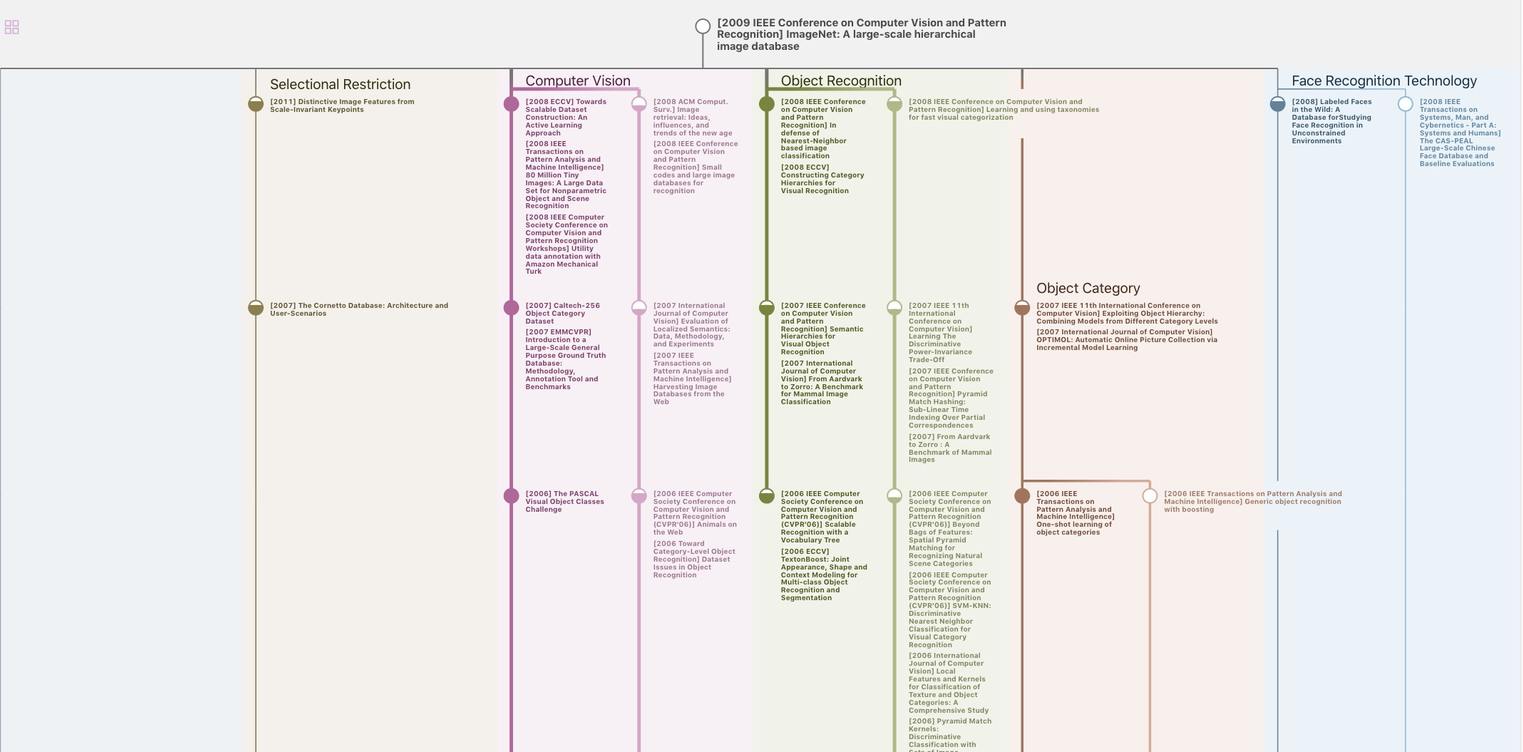
生成溯源树,研究论文发展脉络
Chat Paper
正在生成论文摘要