Accelerated NMR Spectroscopy: Merge Optimization with Deep Learning
ArXiv(2020)
摘要
Multi-dimensional NMR spectroscopy is an invaluable biophysical tool in studies of structure, interactions, and dynamics of large molecules like proteins and nuclear acids. Non-uniform sampling is a powerful approach for shortening measurement time and increasing spectra resolution. Several methods have been established for spectra reconstruction from the undersampled data, typical approaches include model-based optimization and datadriven deep learning. The former is well theoretically grounded and provides high-quality spectra, while the latter has a huge advantage in reconstruction time potential and push further limits of spectra quality and analysis. Combining the merits of the two, we propose a model-inspired deep learning, for reliable, high-quality, and ultrafast spectra reconstruction, exemplified by multi-dimensional spectra of several representative proteins. We demonstrate that the proposed network needs very few parameters, and shows very high robustness in respect to dissimilarity of the training and target data in the spectra size, type, and sampling level. This work can be considered as a proof-of-concept of merging optimization with deep learning in NMR spectroscopy. NMR spectroscopy serves as an indispensable biophysical tool in modern chemistry, biology, and life science1-4. Since the duration of an NMR experiment is proportional to the number of measured data points and increases rapidly with the dimensionality and spectral resolution, accelerating the measurement time by using the non-uniform sampling (NUS) approach5,6 becomes widely accepted practice. Over the past two decades, a number of methods have been successfully used in the NMR field to reconstruct high-quality spectra from NUS data. The two distinct strategies are modelor prior-based optimization7-19 and data-driven deep learning20-24. The former has the explicit modeling and specific priors with insights from NMR spectroscopy, while the latter relies on low-complexity algorithms to greatly reduce the reconstruction time. For model-based optimization methods, similarly, they rely on the prior knowledge or assumptions, to compensate for the lack of information introduced by the NUS scheme. A range of modern approaches include the maximum entropy6, spectral lineshape estimation7, tensor structures5, compressed sensing (CS)8-13, and low-rank Hankel matrices and tensors14-19. Among them, CS introduces the reasonable assumption of NMR spectral sparsity, and utilizes the explicit structure and simple iterative algorithms to reconstruct NMR spectra. It can be regarded as an effective self-learning method by iterating on a single input. Nevertheless, the sparseness prior of the CS has limitations on broad peaks14 and the computations are lengthy. Moreover, with the decrease of the NUS densities and the increase of the spectra size, the computational time for reconstructing high-quality spectra increases significantly, which drives development of new techniques. Data-driven deep learning (DL) is a representative artificial intelligence technique utilizing neural networks25. Due to the low-complexity non-iterative algorithm and massive parallelization with graphic processing units (GPUs), a trained network can reconstruct a high-quality spectrum20-24 very fast. However, the existing NMR DL networks contain too many adjustable parameters and are over-parametrized. Consequently, they require very large pool of date for training, an excessively large operative memory, and suffer from lack of robustness and explainability23. Hence, the question remains, can a deep network achieve even better performance in spectra quality and computational efficiency while having an understandable architecture for human NMR experts? Now, we feel strongly that, so far independent development of optimization and deep learning methods approached to the merging stage, and it is a trend of the future technology development. Effectiveness of fusion between the optimization and deep learning has been recently successfully demonstrated in signal processing and other areas26-30, which encourages us to provide a clear guidance for merging the optimization and deep learning in NMR. Herein, we introduce a Model-inspired Deep learning framework, called MoDern. Our design of architecture starts from the sparse prior knowledge and adopts the main idea from the classic CS algorithm: iterative soft-thresholding (IST)10,13. Let r be the complete NMR time-domain signal, called free induction decay (FID), and the forward Fourier transform F converts it into a NMR spectrum x Fr . In NMR spectroscopy, CS model states that the sparsest solution can be always found by the 1 l norm optimization10,11,31. This task can be efficiently solved by the IST algorithm, and its ( 1, , ) th k k K iteration process can be written as follows10,13: (Data Consistency) 1 1 T H k k k d x FU y UF x , (1a) (Soft-thresholding) , k k S x d , (1b) where y is the FID signal undersampled by operator U , H F is the inverse Fourier transform, d is the spectrum after data consistency, the superscript T is the transpose operator, is the threshold, and , max ,0 S x x x x is the softthresholding operator. Initialized with 0 T x FU y , CS reconstructs the spectrum by alternating the data consistency and the soft-thresholding. Once the overall number of iterations is fixed, the data flow can be viewed as an unfolded deep learning network, as shown in Figure 1. Same to Eq. (1a), the spectrum is forced to maintain the data consistency to the sampled signal. Since the proper choice of thresholds is still of great demand and challenging, thus, instead of Eq. (1b), we use a learnable network LS which can change thresholds with the characteristics of the input data, for adaptive soft-thresholding. The single network LS is composed of convolutional layers, fully-connected layers, and a soft-thresholding (See in the Supplement S1.2 for details). Our experiments demonstrate the effectiveness of this scheme (See in the Supplement S1.4 for details). With the increase of iterations, artifacts are gradually removed, and finally a highquality reconstructed spectrum can be obtained. Given the success of training neural networks using solely synthetic data with the exponential functions20,22, we also employ this scheme to train our network to learn the best internal parameters Θ̂ and an optimal mapping ˆ , f y Θ by minimizing the mean square error between outputs of the learnable adaptive soft-thresholding and fully sampled spectra. For a well-trained network, the spectrum x can be reconstructed reliably and fast from an undersampled signal y via ˆ , f y Θ . Notably, the self-learning CS approach often needs lots of iterations, however, the trained deep learning network with the fixed number of iterations is a simple forward model, which greatly shorten the reconstruction time. Figure 1. MoDern: The proposed Model-inspired Deep Learning framework for NMR spectra reconstruction. The recursive MoDern framework that alternates between the data consistency (DC), which is same to Eq. (1a), and the learnable adaptive soft-thresholding (LS) inspired by Eq. (1b). With the increase of the iteration, artifacts are gradually removed, the spectrum tends to be “sparse”, and finally a high-quality reconstructed spectrum can be obtained. Note: “FT” is the Fourier transform. A data consistency followed by a learnable adaptive soft-thresholding constitutes an iteration. MoDern is inspired by the CS, but go beyond it due to the learning ability of the deep neural network. For example, we solve the limitation of CS on broad peaks. Figure 2 shows a comparison between a synthetic fully sampled reference spectrum and its NUS reconstructions obtained using CS10 and MoDern. The spectrum contains five peaks with different line widths. It can be observed that while the narrowest Peak 5 is successfully recovered, the other four peaks have visible distortions exacerbated as the peaks get broader (Figure 2b). Meanwhile, MoDern reconstructs all five peaks faithfully (Figure 2c). Figure 2d and 2e show that, for the NUS density in the range 10%~20%, MoDern has higher peak intensity correlations to the reference spectrum than CS in recovering three broadest peaks, and results are comparable for the remaining peaks. Similar results have been observed on a 2D 1H-15N HSQC spectrum of the cytosolic domain of CD79b in Figure 3 (See in the Supplement S2 for details). Thus, we can conclude that, MoDern produces at least as good spectra reconstruction as CS and often outperforms it for broadest and weakest peaks, which indicate high effective sensitivity32 of MoDern. By abandoning a large number of redundant convolution layers, which is often used in the data-driven deep learning20,22, number of the network parameters of MoDern is ca 9% of that needed for the state-of-the-art DLNMR20 (Figure 4a), resulting in a significant reduction in both training and reconstruction time (Figure 4b) without loss of spectral reconstruction quality (See in the Supplement S4 for details). Since the integration of optimization alleviates the network's dependence on the training dataset, MoDern is very flexible and can overcome the mismatch between training and real datasets, e.g. difference in spectra size, type, and NUS density, to work effectively in a wide range of scenarios without re-training. Thus, Figure 4c and 4d show that MoDern maintains the high-quality and significantly better than DLNMR reconstruction performance when the NUS density of spectra significantly deviates from the level used in the training (See in the Supplement S3 for details). Figure 2. Reconstruction of the synthetic spectrum with five peaks. (a) The fully sampled spectrum. (b) and (c) are the reconstructed spectra using CS and MoDern from 20% data, respectively. (d) and (e) are peak intensity correlations between fully sampled spectrum and reconstructed
更多查看译文
关键词
nmr spectroscopy,deep learning,merge optimization
AI 理解论文
溯源树
样例
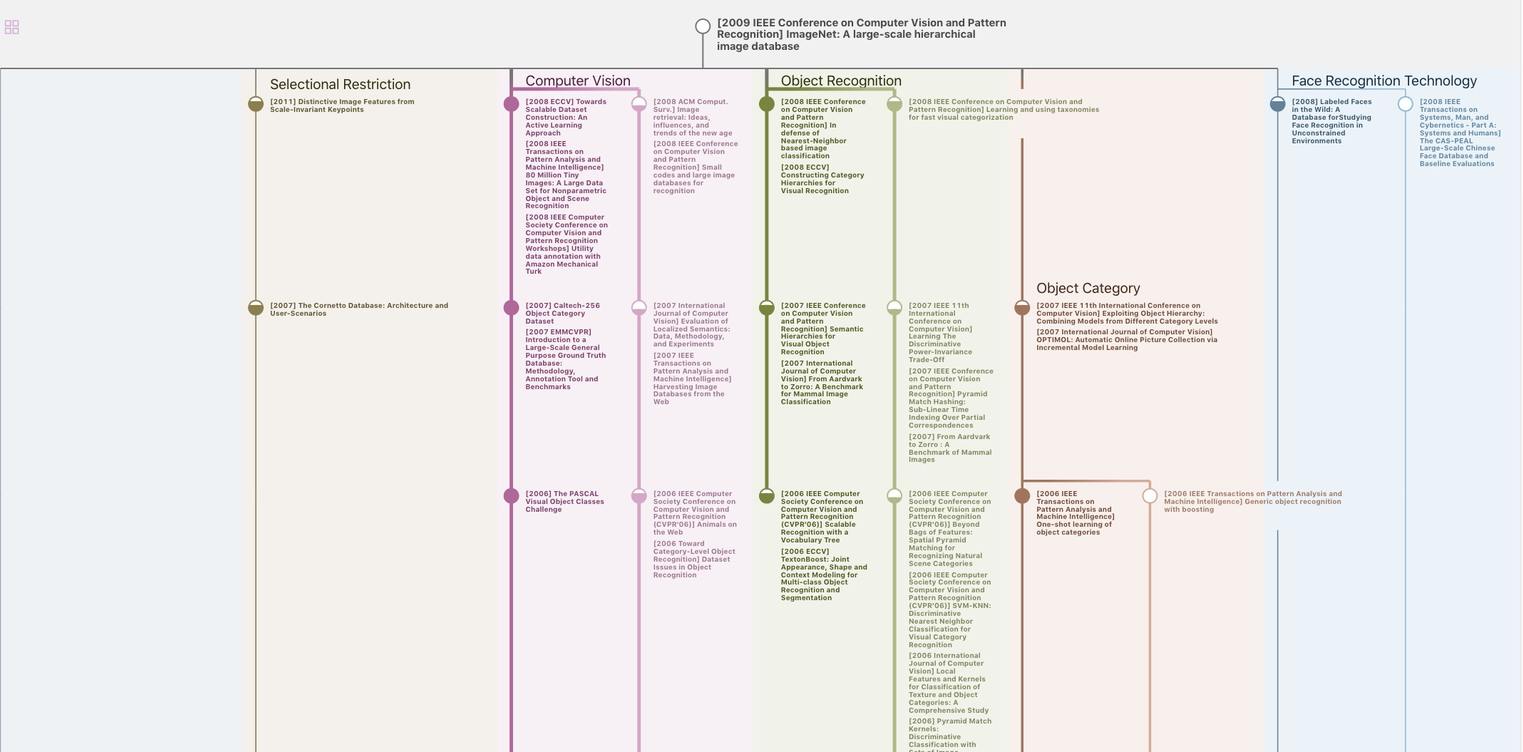
生成溯源树,研究论文发展脉络
Chat Paper
正在生成论文摘要