The COVID-19 Pandemic from the Eye of the Virus
semanticscholar(2021)
摘要
The all-pervasive lens that humans ordinarily use to watch and analyze the pandemic is time. This article considers an alternative. Instead of tracking incidence as a function of time, new cases are counted as a function of cumulative cases. This resource-centric perspective, which is more natural and physically justified, is the perspective of the virus. In this article, we demonstrate the relevance of this approach by characterizing an outbreak as an independent increments Gaussian process that fluctuates about a deterministic curve, called the incidence-cumulative cases (ICC) curve. We illustrate these concepts on Influenza A and COVID-19 outbreaks in the US. The novel perspective presented here reveals universal properties of disease spread that would otherwise remain hidden. As evidenced by the COVID-19 pandemic, societies throughout the world are highly vulnerable to disease outbreaks [1]. Understanding disease transmission is therefore crucial to timely and effective public health policies. To this end, scientists have developed numerous mathematical, statistical, and computational models of infectious disease dynamics [2]. But because disease spread is inherently complex, such descriptions often rely on large numbers of parameters that may be difficult to estimate. Any effort that reduces model complexity while maintaining core features is therefore invaluable. In most instances, the independent variable underlying the course of an epidemic is time: health authorities report numbers of new cases and deaths per day or week, forming what is commonly called an epidemiological (EPI) curve (see examples in the top left panel of Figure 1); and modelers fit their models to this same EPI curve. However, time – as 1 ar X iv :2 10 3. 12 84 8v 1 [ qbi o. PE ] 2 3 M ar 2 02 1 we measure it – is not intrinsic to virus spread dynamics. As such, we argue that when focusing on temporal aspects of virus spread, many modeling and inference efforts obscure relevant properties of the dynamics, thereby making it more difficult to fit models to data. Dynamical systems theory has long promoted a “phase portrait” perspective that can provide both intuitive insights and analytical approaches not easily identified under the normal time domain description. Expanding on the viewpoint fostered in [3, 4] to apply such a perspective to outbreak analysis, this article reveals how simple universal structures emerge from microscopic stochastic interactions. It also offers a more refined and streamlined analysis of important statistical properties of single disease outbreaks. Outbreaks beyond the time domain Finding an alternative domain to analyze epidemics begins by considering the reachable available resources for the pathogen. From this standpoint, susceptible hosts are the resources. At each point in time, all that matters for a pathogen is what resources are available for further invasion or, equivalently if infectivity is high, what resources have been used. As illustrated in Figure 1, this point of view offers valuable insight on important traits shared by different outbreaks associated with the same pathogen. The left plot of the top row shows the EPI curves of the 24 Inluenza A (H3N2) outbreaks that took place in US HHS regions between 1998 and 2019 and led to more than 3000 confirmed cases. No specific properties of these curves are readily observable, because the peak timing and peak height vary between seasons. However, when the same curves are plotted in the incidence vs. cumulative cases (ICC) plane, a structure emerges, revealing similarities between each season that, as we will see, are characteristic of the disease itself. To emphasize that such properties are generic, the bottom row of Figure 1 shows similar results for multiple instances of the spread of a disease on a network, as described by a stochastic SIR (Susceptible, Infected, Recovered) model. Again, the universality normally hidden behind classical EPI curves becomes evident once time is removed from the picture and the independent variable is replaced with cumulative cases. In the deterministic compartmental SIR model, the total number of cases is C = I+R, and the total population n = S−C is fixed. It was shown in [4] that knowledge of C(t) is equivalent to knowledge of S(t), I(t), and R(t), and that the SIR dynamics can be recast as an autonomous differential equation of the form Ī = dC/dt = nG(C/n), where Ī is the deterministic incidence, C is the cumulative number of cases, and G is a function of C that depends on the parameters of the outbreak. The relevance of the SIR model to COVID-19 outbreaks is illustrated in Figure 2, which shows daily incidence in the state of Arizona for the 2020 calendar year, both in the time domain (top row: standard EPI curve) and in the cumulative cases domain (bottom row: ICC curve). The first arrow marks the end of the initial stay at home period (03/19/2020 05/15/2020) ordered by the Governor of Arizona [5, 6, 7]; the second arrow, on August
更多查看译文
AI 理解论文
溯源树
样例
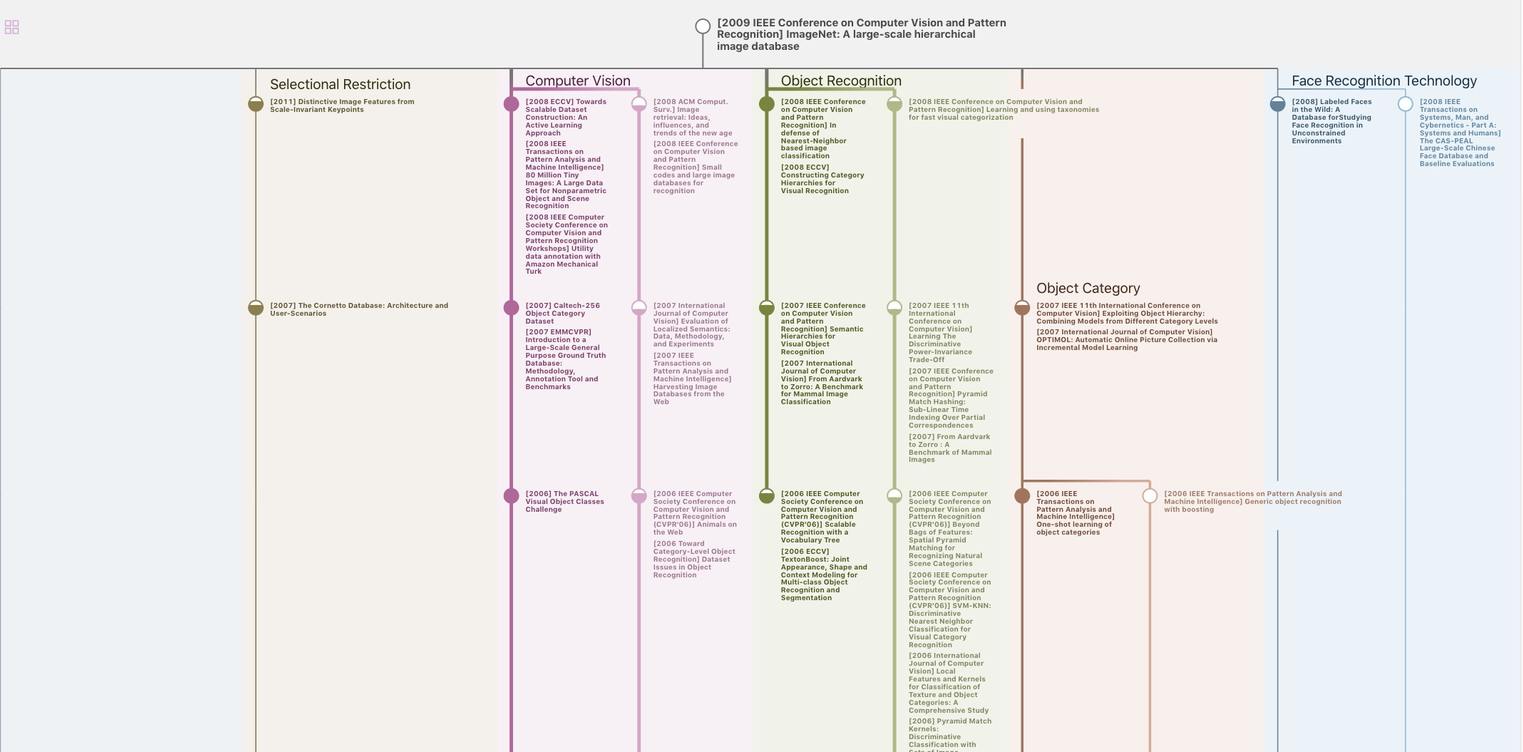
生成溯源树,研究论文发展脉络
Chat Paper
正在生成论文摘要