Frequentist and Bayesian Perspectives on Logistic Regression and Neural Networks
semanticscholar(2019)
摘要
Binary classification is a central problem in statistical practice. A plethora of statistical learning techniques have been developed to address this problem, each with their own benefits and limitations. In this paper, we set out to unify two such techniques, Logistic Regression and Neural Networks, and to analyze a larger model class that utilizes the binomial likelihood to connect covariate information with observed class assignments. We consider training and subsequent inference of these models under both a Frequentist and Bayesian paradigm which allows for a complete analysis of the similarity and differences that these models posit. Finally, we apply these methods to a resume dataset that contains binary features to predict whether an applicant receives an interview.
更多查看译文
AI 理解论文
溯源树
样例
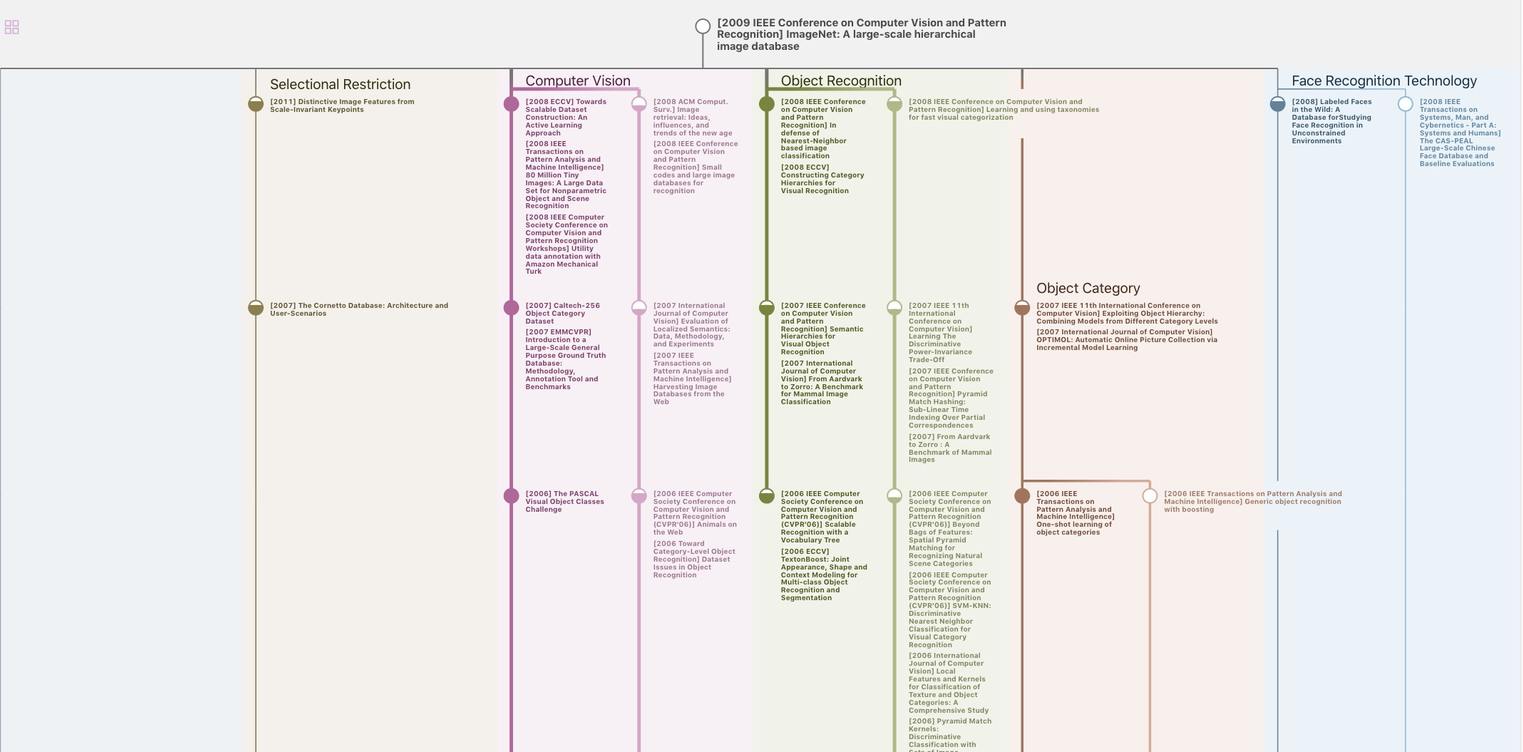
生成溯源树,研究论文发展脉络
Chat Paper
正在生成论文摘要