Scalable cardiovascular risk assessment using artificial intelligence-enabled event adjudication and widely available hematologic predictors.
medRxiv(2021)
摘要
Introduction: Researchers routinely evaluate novel biomarkers for incorporation into clinical risk models. Although of potential benefit, such emerging markers, which are often costly or not yet commercially available, are unlikely to enable the scalable risk assessment needed for population health strategies. In contrast, the ideal inputs for population approaches would be those already widely available for most patients. We hypothesized that simple hematologic markers, available in an outpatient complete blood count without differential, would be useful to develop risk models for cardiovascular events. Methods: Using routine laboratory measurements as predictors and neural network-based automated event adjudication of 1,072,348 discharge summaries, we developed and validated models for prediction of heart attack, ischemic stroke, heart failure hospitalization, revascularization, and all-cause mortality. Results: Models with hematology indices alone showed Harrell's concordance index ranging from 0.60-0.80 on an external validation set. Hematology indices added significantly in terms of discrimination and calibration performance compared to models using only demographic data and diagnostic codes for coronary artery disease, heart failure, and ischemic stroke, with the concordance index of resulting models in the range 0.75-0.85 on an external validation set. Predictive features varied by outcome, and included red blood cell, leukocyte, and platelet indices. Conclusion: We conclude that low-cost ubiquitous inputs, if biologically informative, can provide population-level readouts of risk.
更多查看译文
关键词
scalable cardiovascular risk assessment,available hematologic predictors,risk assessment,event adjudication,intelligence-enabled
AI 理解论文
溯源树
样例
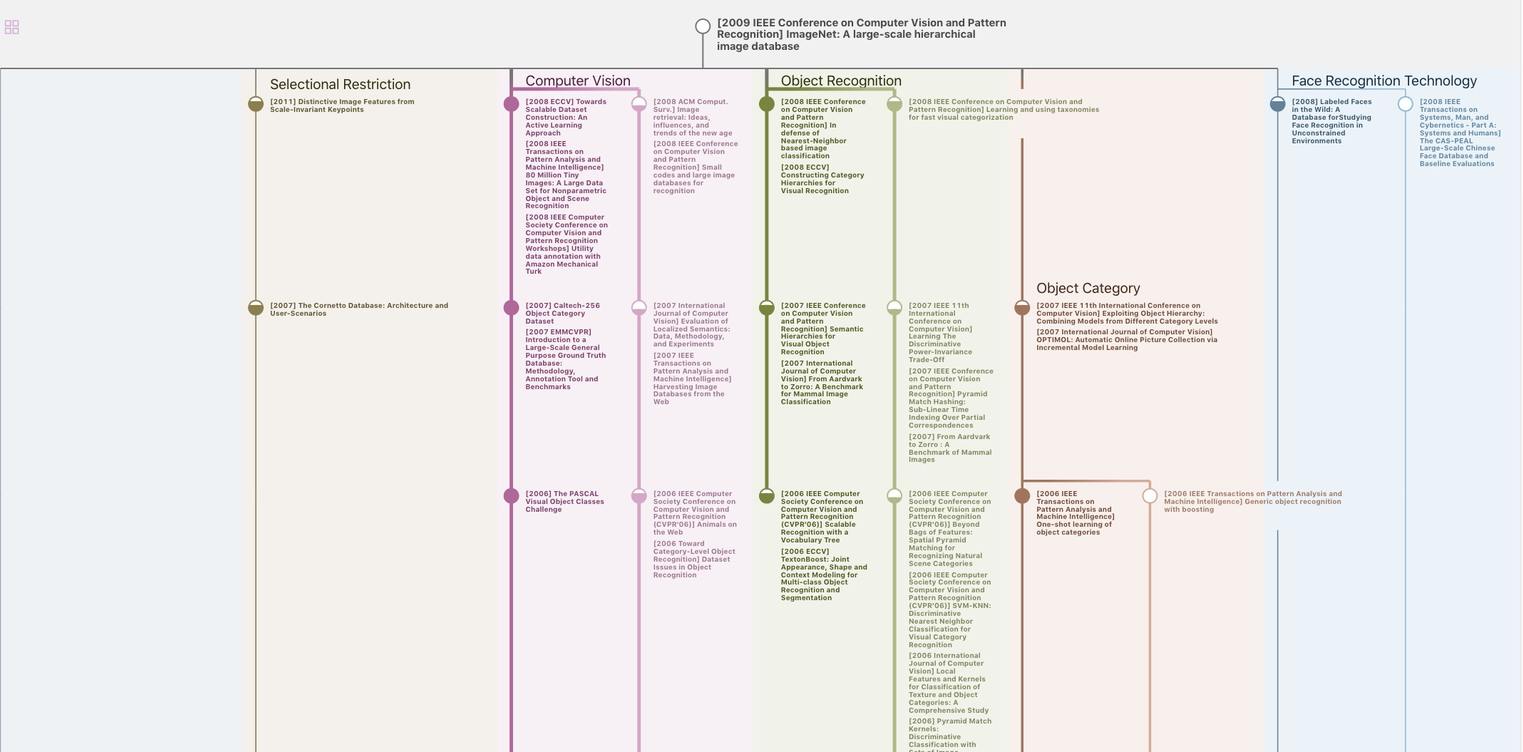
生成溯源树,研究论文发展脉络
Chat Paper
正在生成论文摘要