Unsupervised Hyperspectral Band Selection Based on Hypergraph Spectral Clustering
IEEE GEOSCIENCE AND REMOTE SENSING LETTERS(2022)
摘要
Hyperspectral images can provide spectral characteristics related to the physical properties of different materials, which arouses great interest in many fields. Band selection (BS) could effectively solve the problem of high dimensions and redundant information of HSI data. However, most BS methods utilize a single measurement criterion to evaluate band importance so that the assessment of bands is not comprehensive. To dispose of these issues, we propose the hypergraph spectral clustering band selection (HSCBS) method in this letter. First, a novel hypergraph construction method is proposed to combine bands selected by different priority criteria. Second, based on the hypergraph Laplacian matrix, an unsupervised band selection model named HSCBS is presented to cluster the bands into compact clusters with high within-class similarity and low between-class similarity. The results of comprehensive experimental on two public real datasets demonstrate the effectiveness of HSCBS.
更多查看译文
关键词
Laplace equations,Matrix decomposition,Hyperspectral imaging,Feature extraction,Entropy,Task analysis,Optics,Band selection (BS),hypergraph,hyperspectral imaging,spectral clustering,unsupervised
AI 理解论文
溯源树
样例
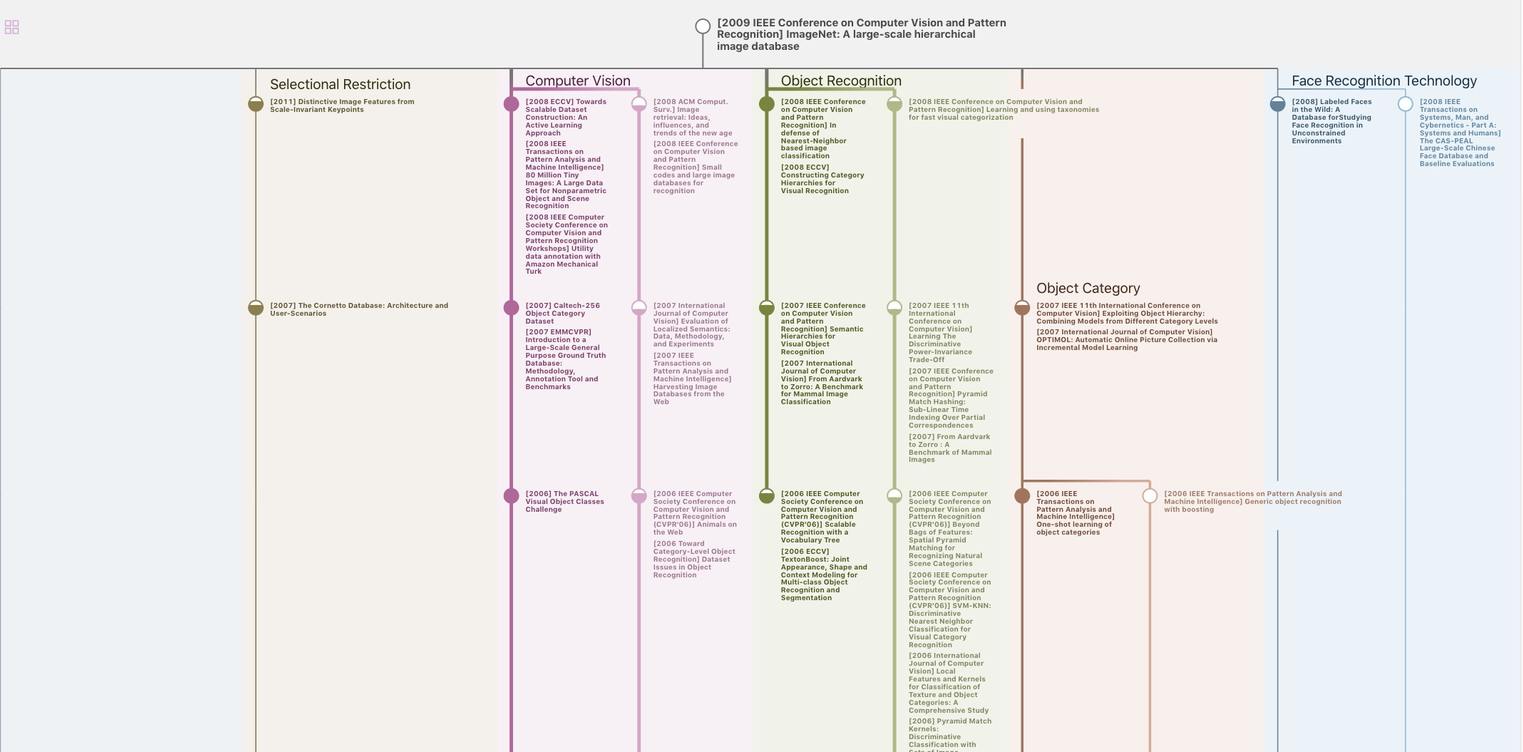
生成溯源树,研究论文发展脉络
Chat Paper
正在生成论文摘要