ScaleHLS: Achieving Scalable High-Level Synthesis through MLIR
semanticscholar(2021)
摘要
High-level Synthesis (HLS) has been widely adopted as it significantly improves the hardware design productivity and enables quick design space exploration (DSE). However, existing HLS tools do not scale well to large designs for two main reasons: (1) The intermediate representations (IR) are not initially designed for HLS, thus are not expressive enough for comprehensive HLS design spaces; (2) The traditional HLS algorithms are based on a singlelevel abstraction, thus cannot easily capture the design hierarchy and are not scalable as the design size grows. To tackle these problems, we present ScaleHLS, a new HLS compilation flow based on a multi-level compiler infrastructure, MLIR. Utilizing MLIR, ScaleHLS introduces a hierarchical representation mechanism for HLS designs, enables scalable optimizations at multi-level abstractions, and directly generates optimized synthesizable HLS code. This approach not only explores the hierarchical design space efficiently but also scales well to large HLS designs. The initial experiments show that comparing to the baseline designs only optimized by the regular LLVM optimizations of Xilinx Vivado HLS, ScaleHLS improves the performance by up to 768.2× on computation kernel level algorithms and 4107.6× on a neural network model MobileNet-v2.
更多查看译文
AI 理解论文
溯源树
样例
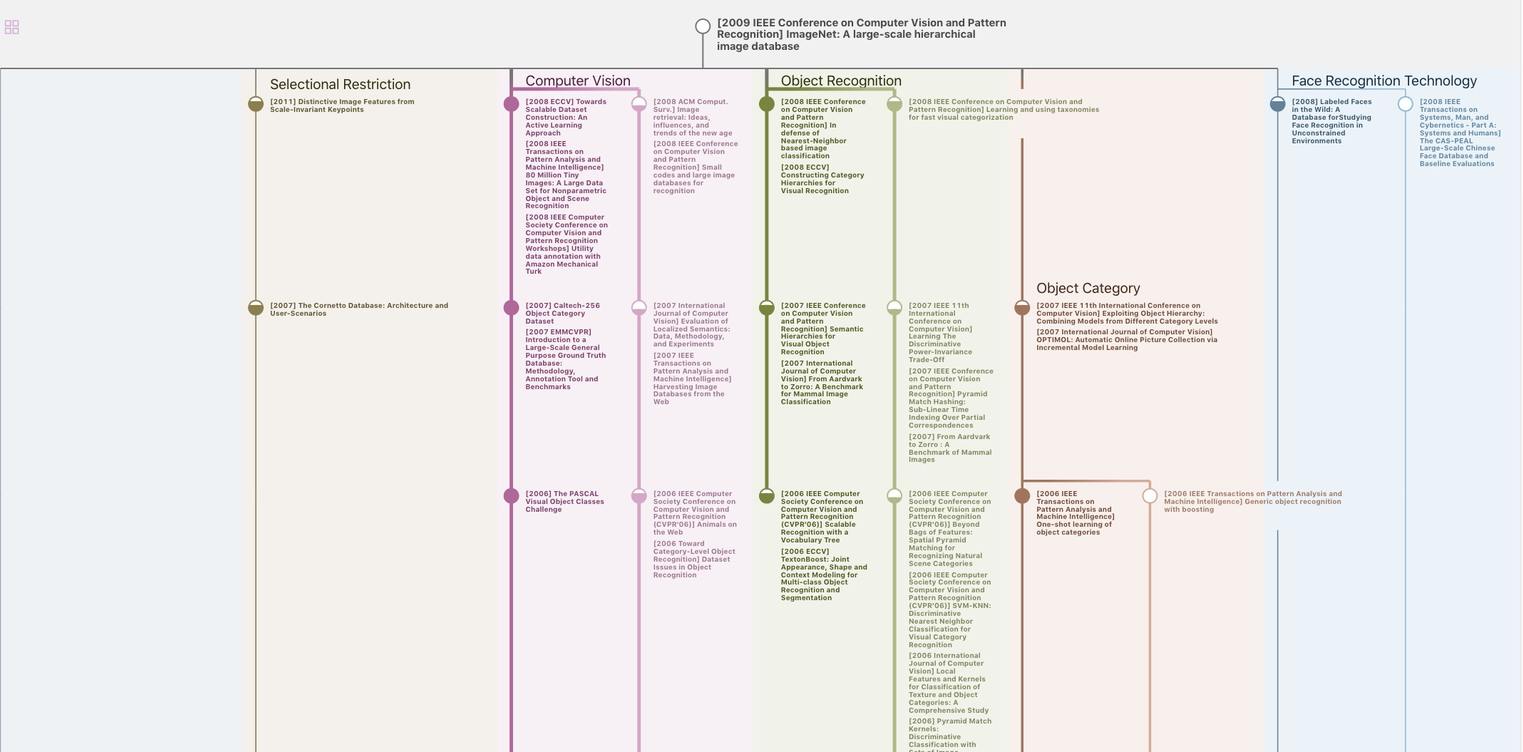
生成溯源树,研究论文发展脉络
Chat Paper
正在生成论文摘要