Addressing Unique Fairness Obstacles within Federated Learning
semanticscholar(2021)
摘要
Efforts to reduce social bias in machine learning has increased in the past several years. As data privacy concerns grow, finding techniques to train private, debiased machine learning models becomes increasingly important. Federated Learning (FL) has emerged as a popular privacy-preserving machine learning strategy. FL, however, by not providing complete access to training data, brings with it a unique set of difficulties in bias mitigation that have yet to be explored. In this paper, we delve into these difficulties, and how they can affect bias measured in federated learning models.
更多查看译文
AI 理解论文
溯源树
样例
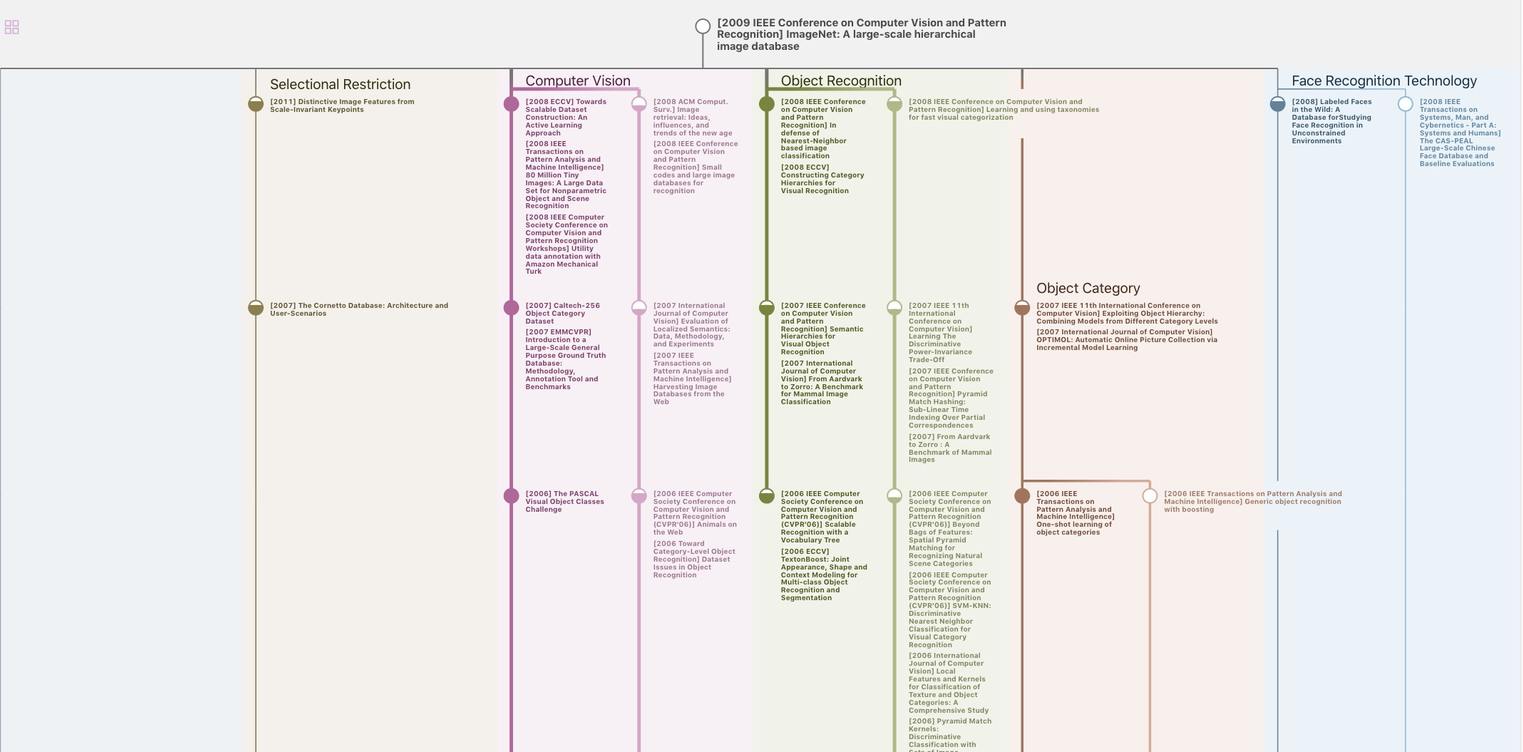
生成溯源树,研究论文发展脉络
Chat Paper
正在生成论文摘要