Bayesian inference for zero-and/or-one augmented beta rectangular regression models
BRAZILIAN JOURNAL OF PROBABILITY AND STATISTICS(2021)
摘要
Abstract. In this paper we developed a full set of Bayesian inference tools, for zero-and/or-one augmented beta rectangular regression models to analyze limited-augmented data, under a new parameterization. This parameterization: facilitates the development of both regression models and inferential tools as well as make simplifies the respective computational implementations. The proposed Bayesian tools were parameter estimation, model fit assessment, model comparison (information criteria), residual analysis and case influence diagnostics, developed through MCMC algorithms. In addition, we adapted available methods of posterior predictive checking, using appropriate discrepancy measures. We conducted several simulation studies, considering some situations of practical interest, aiming to evaluate: prior sensitivity choice, parameter recovery of the proposed model and estimation method, the impact of transforming the observed zeros and ones, along with the use of non-augmented models, and the behavior of the proposed model fit assessment and model comparison tools. A psychometric real data set was analyzed to illustrate the performance of the developed tools, illustrating the advantages of the developed analysis framework.
更多查看译文
关键词
 , Augmented beta rectangular distribution, Bayesian inference, Diagnostic analysis, MCMC algorithms, Generalized linear models
AI 理解论文
溯源树
样例
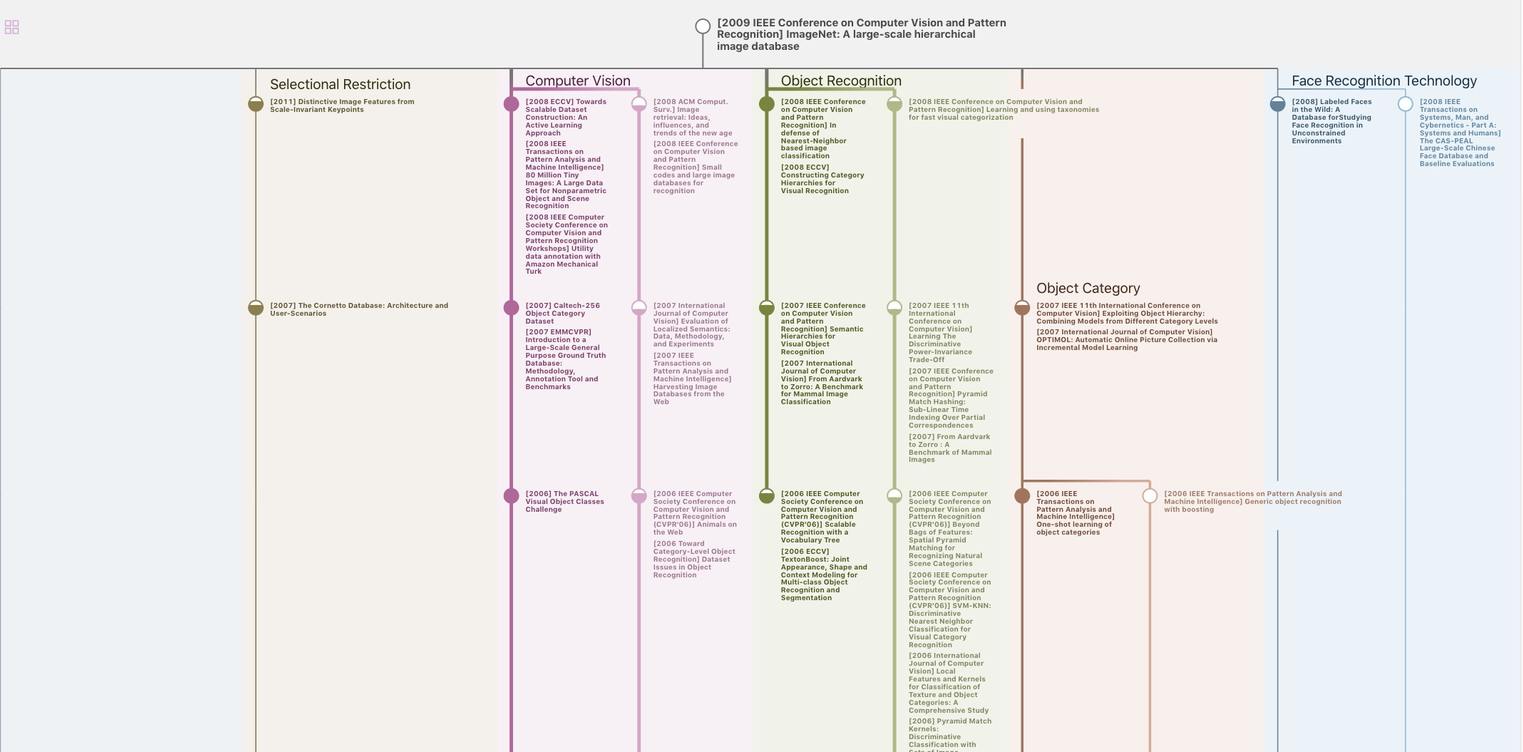
生成溯源树,研究论文发展脉络
Chat Paper
正在生成论文摘要