Automated Evolution of Feature Logging StatementLevels Using Git Histories and Degree of Interest pdfauthor=Yiming Tang, Allan Spektor, Raffi Khatchadourian, and Mehdi Bagherzadeh
semanticscholar(2021)
摘要
Logging—used for system events and security breaches to more informational yet essential aspects of software features—is pervasive. Given the high transactionality of today’s software, logging effectiveness can be reduced by information overload. Log levels help alleviate this problem by correlating a priority to logs that can be later filtered. As software evolves, however, levels of logs documenting surrounding feature implementations may also require modification as features once deemed important may have decreased in urgency and vice-versa. We present an automated approach that assists developers in evolving levels of such (feature) logs. The approach, based on mining Git histories and manipulating a degree of interest (DOI) model, transforms source code to revitalize feature log levels based on the “interestingness” of the surrounding code. Built upon JGit and Mylyn, the approach is implemented as an Eclipse IDE plug-in and evaluated on 18 Java projects with ∼3 million lines of ∗Corresponding author. Email addresses: ytang3@gradcenter.cuny.edu (Yiming Tang), allan.spektor03@myhunter.cuny.edu (Allan Spektor), raffi.khatchadourian@hunter.cuny.edu (Raffi Khatchadourian), mbagherzadeh@oakland.edu (Mehdi Bagherzadeh) URL: http://linkedin.com/in/gracetang1993 (Yiming Tang), https://www.linkedin.com/in/allan-spektor (Allan Spektor), http://cs.hunter.cuny.edu/~Raffi.Khatchadourian99 (Raffi Khatchadourian), http://mbagherz.bitbucket.io (Mehdi Bagherzadeh) 1Degree of interest model (DOI) was proposed by Kersten and Murphy [1] to gauge the degree of developers’ interests in program elements. Preprint submitted to Science of Computer Programming April 19, 2021 ar X iv :2 10 4. 07 73 6v 1 [ cs .S E ] 1 5 A pr 2 02 1 code and ∼4K log statements. Our tool successfully analyzes 99.26% of logging statements, increases log level distributions by ∼20%, identifies logs manually modified with a recall of ∼80% and a level-direction match rate of ∼87%, and increases the focus of logs in bug fix contexts ∼83% of the time. Moreover, pull (patch) requests were integrated into large and popular open-source projects. The results indicate that the approach is promising in assisting developers in evolving feature log levels.
更多查看译文
AI 理解论文
溯源树
样例
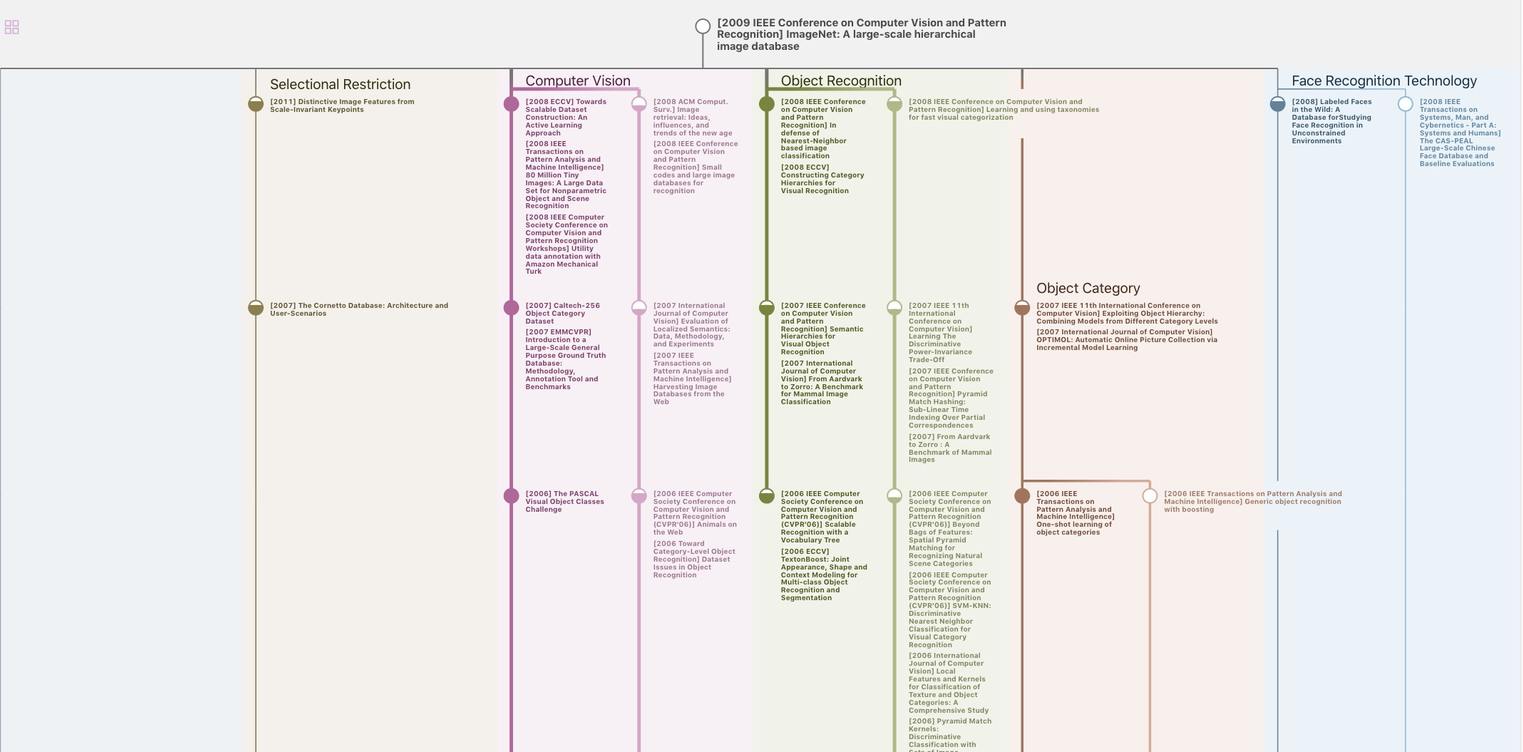
生成溯源树,研究论文发展脉络
Chat Paper
正在生成论文摘要