Quantifying Emotional Similarity in Speech
IEEE Transactions on Affective Computing(2023)
摘要
This study proposes the novel formulation of measuring emotional similarity between speech recordings. This formulation explores the ordinal nature of emotions by comparing emotional similarities instead of predicting an emotional attribute, or recognizing an emotional category. The proposed task determines which of two alternative samples has the most similar emotional content to the emotion of a given anchor. This task raises some interesting questions. Which is the emotional descriptor that provide the most suitable space to assess emotional similarities? Can deep neural networks (DNNs) learn representations to robustly quantify emotional similarities? We address these questions by exploring alternative emotional spaces created with attribute-based descriptors and categorical emotions. We create the representation using a DNN trained with the triplet loss function, which relies on triplets formed with an anchor, a positive example, and a negative example. We select a positive sample that has similar emotion content to the anchor, and a negative sample that has dissimilar emotion to the anchor. The task of our DNN is to identify the positive sample. The experimental evaluations demonstrate that we can learn a meaningful embedding to assess emotional similarities, achieving higher performance than human evaluators asked to complete the same task.
更多查看译文
关键词
Task analysis,Emotion recognition,Speech recognition,Affective computing,Face recognition,Measurement,Reliability,Speech emotion recognition,ordinal affective computing,representation learning of emotion similarity,triplet loss function,speech emotion retrieval
AI 理解论文
溯源树
样例
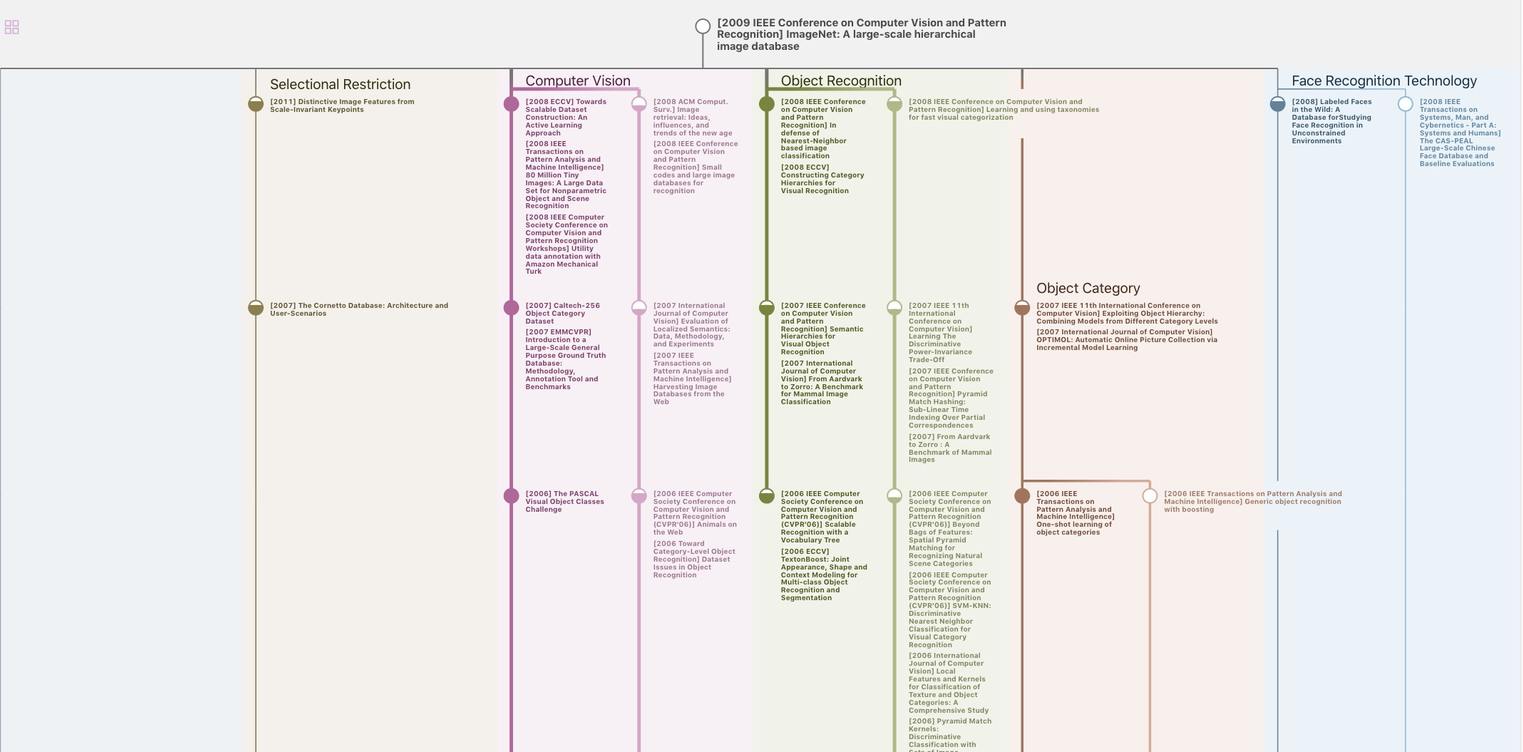
生成溯源树,研究论文发展脉络
Chat Paper
正在生成论文摘要