Global dynamic optimization with Hammerstein–Wiener models embedded
Journal of Global Optimization(2022)
摘要
Hammerstein–Wiener models constitute a significant class of block-structured dynamic models, as they approximate process nonlinearities on the basis of input–output data without requiring identification of a full nonlinear process model. Optimization problems with Hammerstein–Wiener models embedded are nonconvex, and thus local optimization methods may obtain suboptimal solutions. In this work, we develop a deterministic global optimization strategy that exploits the specific structure of Hammerstein–Wiener models to extend existing theory on global optimization of systems with linear dynamics. At first, we discuss alternative formulations of the dynamic optimization problem with Hammerstein–Wiener models embedded, demonstrating that careful selection of the optimization variables of the problem can offer significant numerical advantages to the solution approach. Then, we develop convex relaxations for the proposed optimization problem and discuss implementation aspects to obtain the global solution focusing on a control parametrization technique. Finally, we apply our optimization strategy to case studies comprising both offline and online dynamic optimization problems. The results confirm an improved computational performance of the proposed solution approach over alternative options not exploiting the linear dynamics for all considered examples. They also underline the tractability of deterministic global dynamic optimization when using few control intervals in online applications like nonlinear model predictive control.
更多查看译文
关键词
Global optimization,Dynamic systems,Data-driven modeling,Hammerstein–Wiener,Optimal control,NMPC
AI 理解论文
溯源树
样例
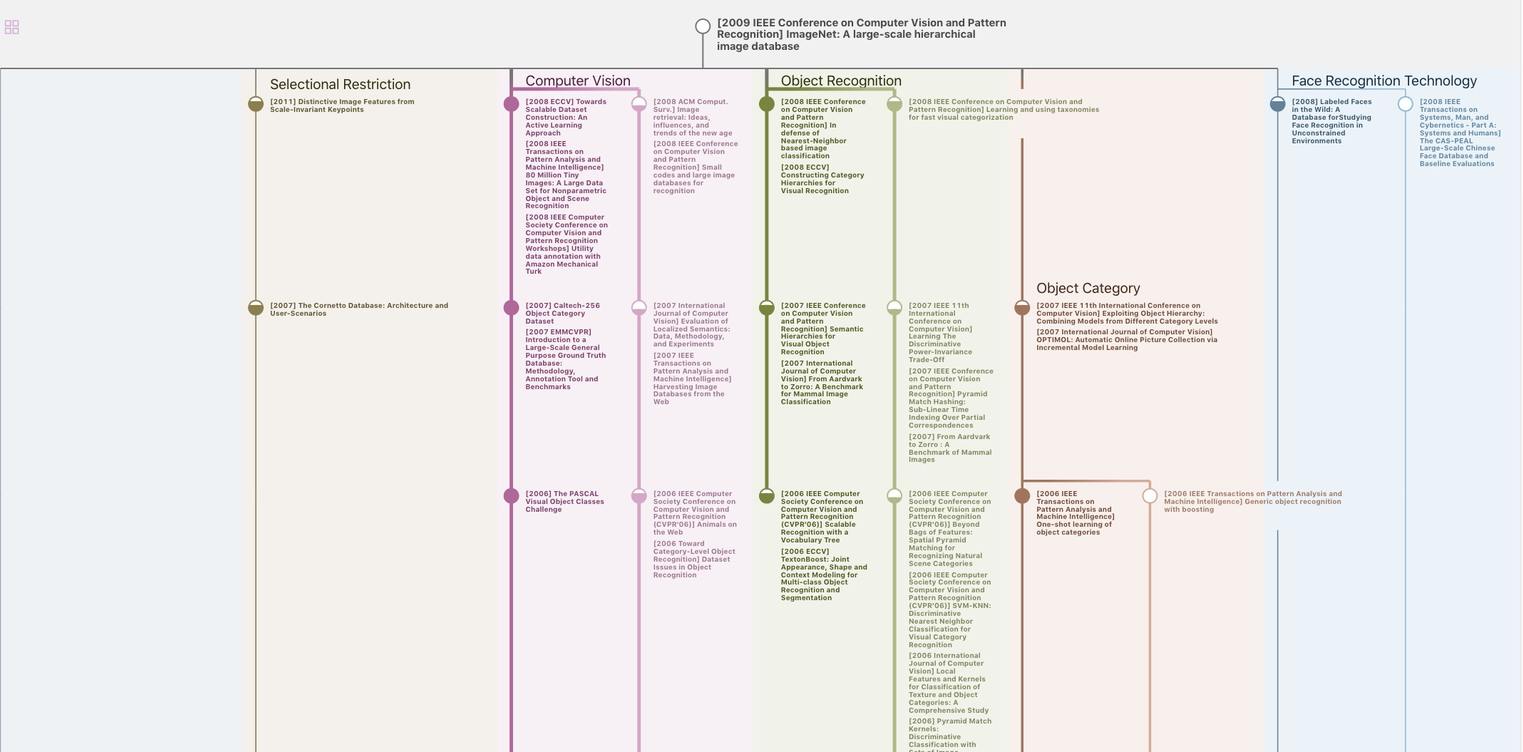
生成溯源树,研究论文发展脉络
Chat Paper
正在生成论文摘要