A Computational Dual-Process Model of Fixation-Duration Control in Natural Scene Viewing
Computational Brain & Behavior(2021)
摘要
Research on eye-movement control during natural scene viewing has investigated the degree to which the duration of individual fixations can be immediately adjusted to ongoing visual-cognitive processing demands. Results from several studies using the fixation-contingent scene quality paradigm suggest that the timing of fixations adapts to stimulus changes that occur on a fixation-to-fixation basis. Analysis of fixation-duration distributions has revealed that saccade-contingent degradations and enhancements of the scene stimulus have two qualitatively distinct types of influence. The surprise effect begins early in a fixation and is tied to surprising visual events such as unexpected stimulus changes. The encoding effect is tied to difficulties in visual-cognitive processing and occurs relatively late within a fixation. Here, we formalize an existing descriptive account of these two effects (referred to as the dual-process account) by using stochastic simulations. In the computational model, surprise and encoding related influences are implemented as time-dependent changes in the rate at which saccade timing and programming are completed during critical fixations. The model was tested on data from two experiments in which the luminance of the scene image was either decreased or increased during selected critical fixations (Walshe & Nuthmann, Vision Research , 100, 38–46, 2014 ). A counterfactual method was used to remove model components and to identify their specific influence on the fixation-duration distributions. The results suggest that the computational dual-process model provides a good account for the data from the luminance-change studies. We describe how the simulations can be generalized to explain a diverse set of experimental results.
更多查看译文
关键词
Computational modelling, Random walk, Fixation duration, Saccades, Naturalistic scenes, Double step
AI 理解论文
溯源树
样例
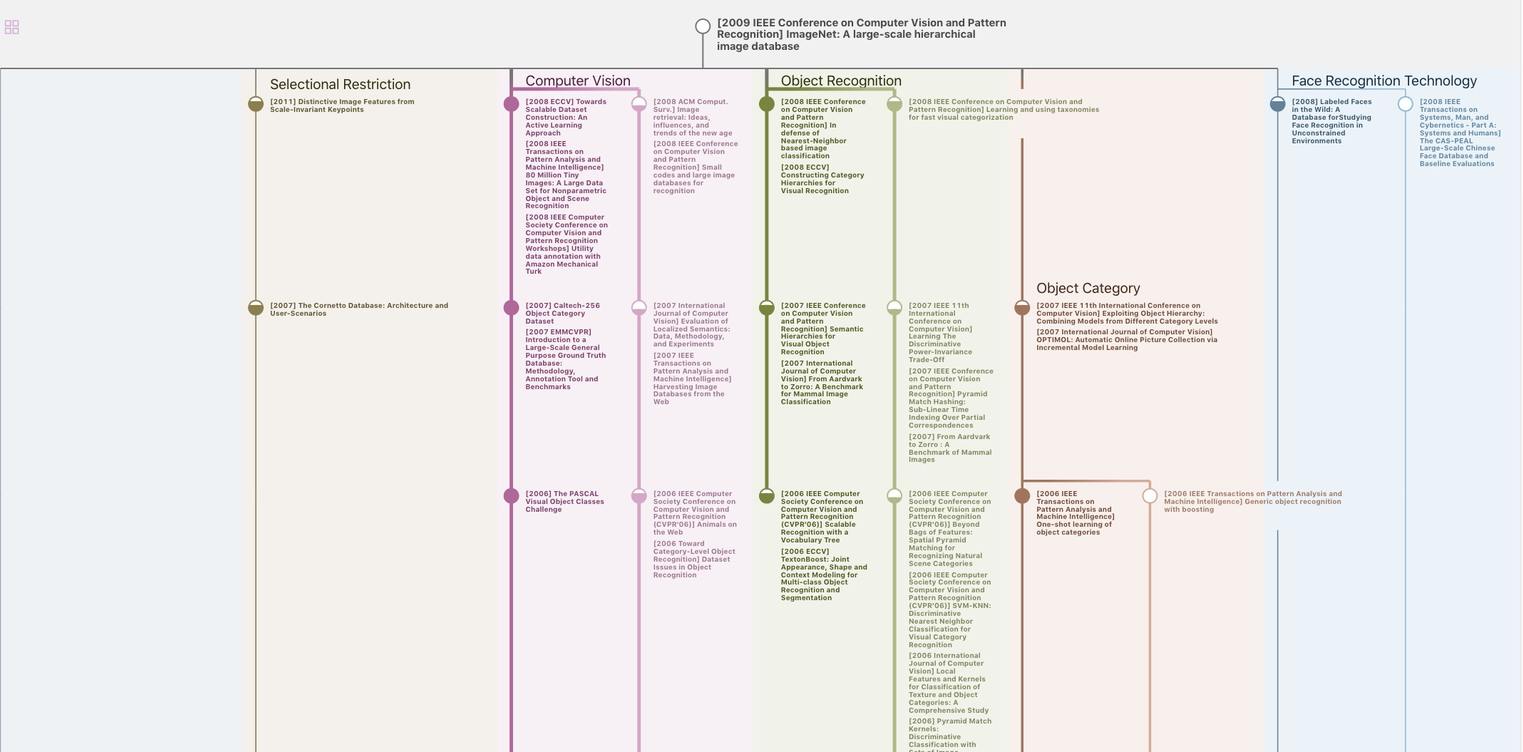
生成溯源树,研究论文发展脉络
Chat Paper
正在生成论文摘要