Alfven eigenmode classification based on ECE diagnostics at DIII-D using deep recurrent neural networks
NUCLEAR FUSION(2022)
摘要
Modern tokamaks have achieved significant fusion production, but further progress towards steady-state operation has been stymied by a host of kinetic and MHD instabilities. Control and identification of these instabilities is often complicated, warranting the application of data-driven methods to complement and improve physical understanding. In particular, Alfven eigenmodes are a class of ubiquitous mixed kinetic and MHD instabilities that are important to identify and control because they can lead to loss of confinement and potential damage to the walls of a plasma device. In the present work, we use reservoir computing networks to classify Alfven eigenmodes in a large labeled database of DIII-D discharges, covering a broad range of operational parameter space. Despite the large parameter space, we show excellent classification and prediction performance, with an average hit rate of 91% and false alarm ratio of 7%, indicating promise for future implementation with additional diagnostic data and consolidation into a real-time control strategy.
更多查看译文
关键词
DIII-D,electron cyclotron emission,Alfven eigenmodes,reservoir computing networks,plasma control
AI 理解论文
溯源树
样例
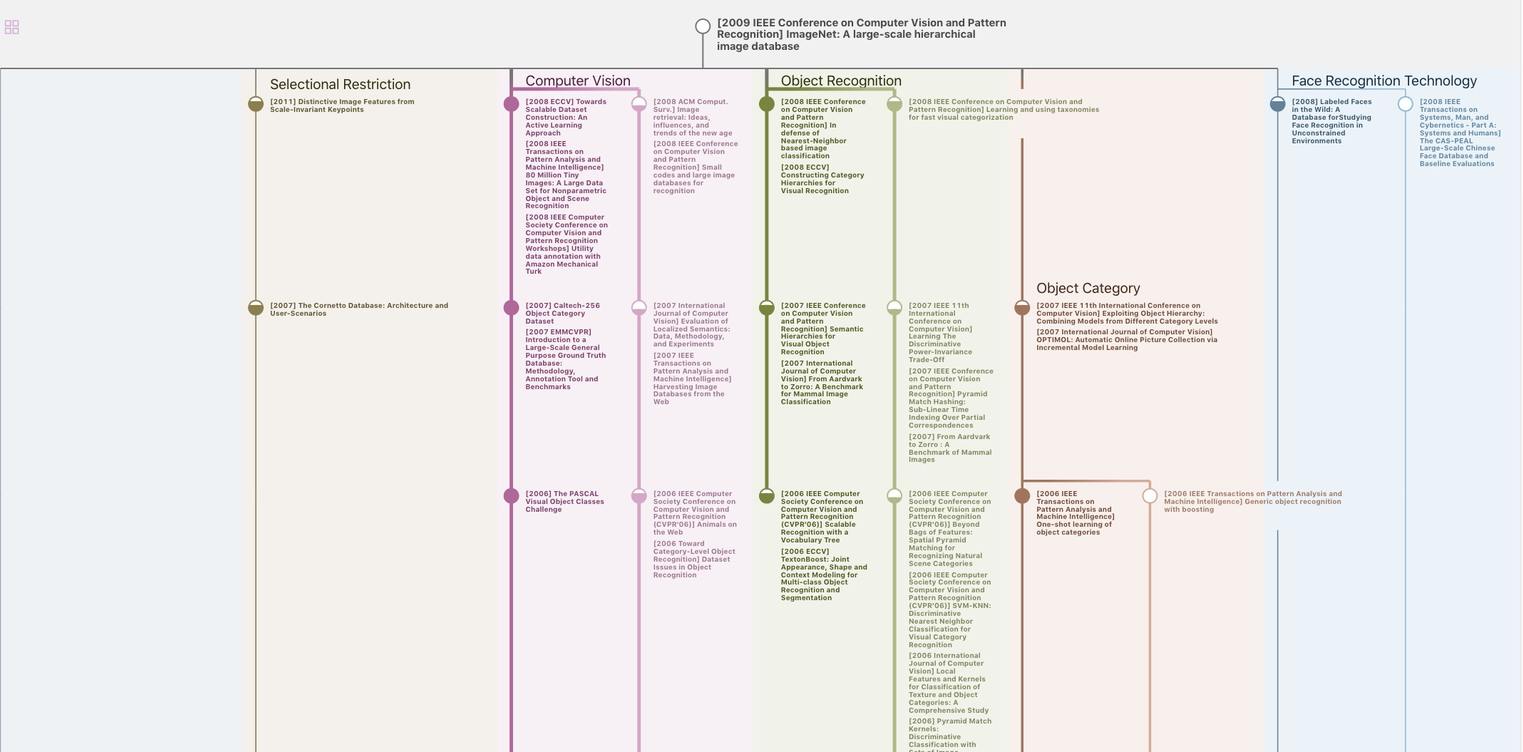
生成溯源树,研究论文发展脉络
Chat Paper
正在生成论文摘要