Monitoring the Damage of Armyworm in Summer Corn by Unmanned Aerial Vehicle Imaging
Research Square (Research Square)(2020)
摘要
Background: Monitoring armyworm (Mythimna separata Walker) damage in crops requires timely, rapid and accurate observations to avoid severe yield losses. Results: The Random Forest (RF) classifier was more effective at automatically and accurately monitoring armyworm damage compared with Support Vector Machine (SVM), Multilayer Perceptron Classifier (MLPC) and Naive Bayes Classifier (NB) classifiers. Furthermore, the incorporation of an Unmanned Aerial Vehicle (UAV) image-generated digital surface model improved the performance of the RF classifier, increasing the F-score from 0.985 and 0.970 to 0.997 and 0.994, and increasing the Kappa coefficient from 0.955 to 0.990. In addition, we found that Band 3 (735 nm) of the UAV image and Band 6 (740 nm) of a coincident Sentinel-2 image were not sensitive to an armyworm infestation in this study. Conclusions: We developed an accurate algorithm for the automated identification of armyworm-damaged corn plants using UAV images at the field scale. The study also indicated the feasibility of the developed method for monitoring corn armyworm damage at regional scale when combined with Sentinel-2 images.
更多查看译文
关键词
unmanned aerial vehicle imaging,armyworm,unmanned aerial vehicle,summer corn
AI 理解论文
溯源树
样例
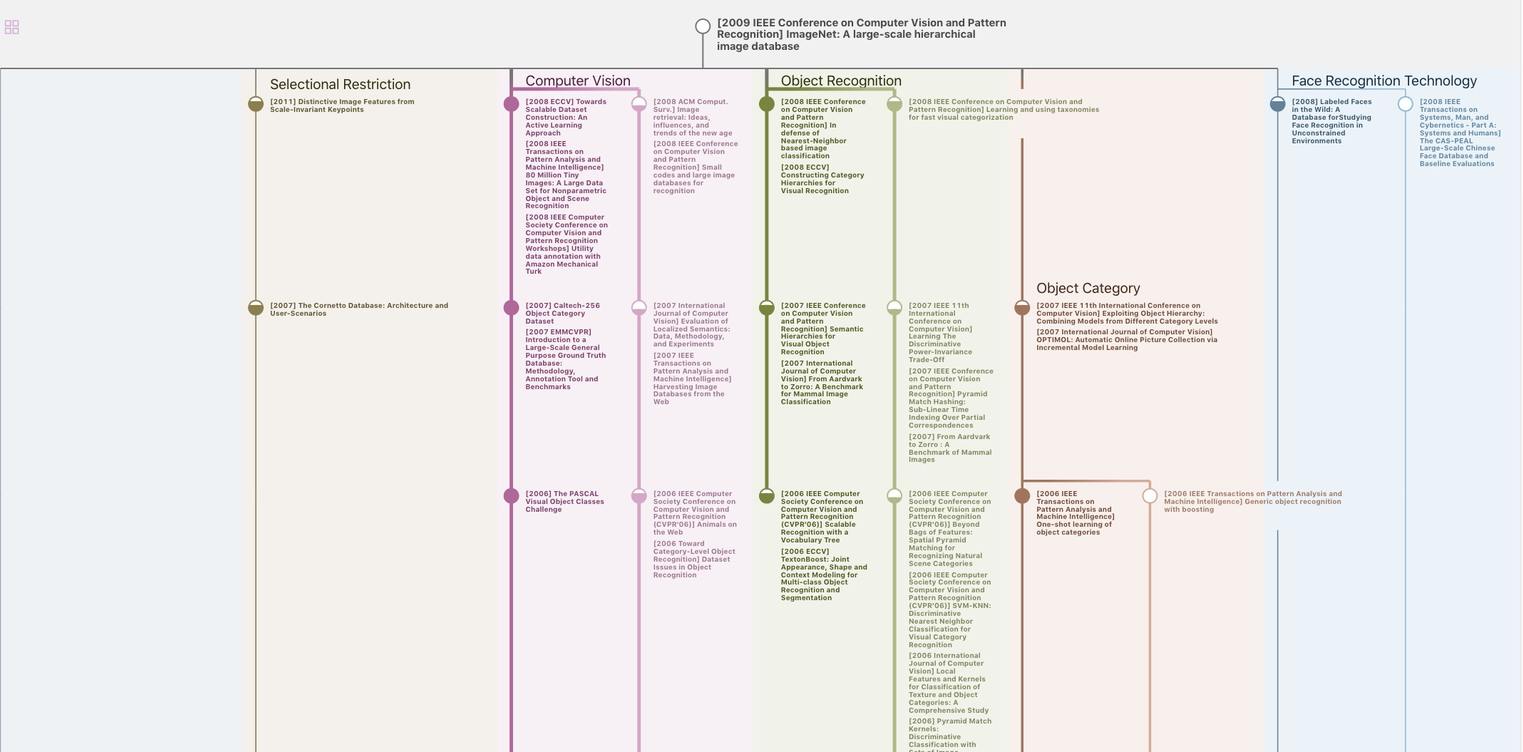
生成溯源树,研究论文发展脉络
Chat Paper
正在生成论文摘要