Application-Driven Learning via Joint Prediction and Optimization of Demand and Reserves Requirement
arXiv (Cornell University)(2021)
摘要
Forecasting and decision-making are generally modeled as two sequential steps with no feedback, following an open-loop approach. In power systems, operators first forecast loads trying to minimize errors with respect to historical data. They also size reserve requirements based on error estimates. Next, energy and reserves are scheduled and the system is operated following the dispatch schedule, deploying reserves as needed to accommodate forecast errors. However, co-optimizing these processes may lead to better decisions and result in lower operating costs than when they are considered sequentially. In this paper, we present a new closed-loop learning framework in which the processes of forecasting and decision-making are merged and co-optimized through a bilevel optimization problem. We prove asymptotic convergence of the method and propose two solution approaches: an exact method based on the KKT conditions of the second level problem, and a scalable heuristic approach suitable for decomposition methods. We benchmark our methodology with the standard sequential least-squares forecast and dispatch planning process. We apply the proposed methodology to an illustrative system and to a wide range of systems ranging from dozens to thousands of buses. Our results show that the proposed approach yields consistently better performance than the standard open-loop approach.
更多查看译文
关键词
joint prediction,demand,learning,optimization,application-driven
AI 理解论文
溯源树
样例
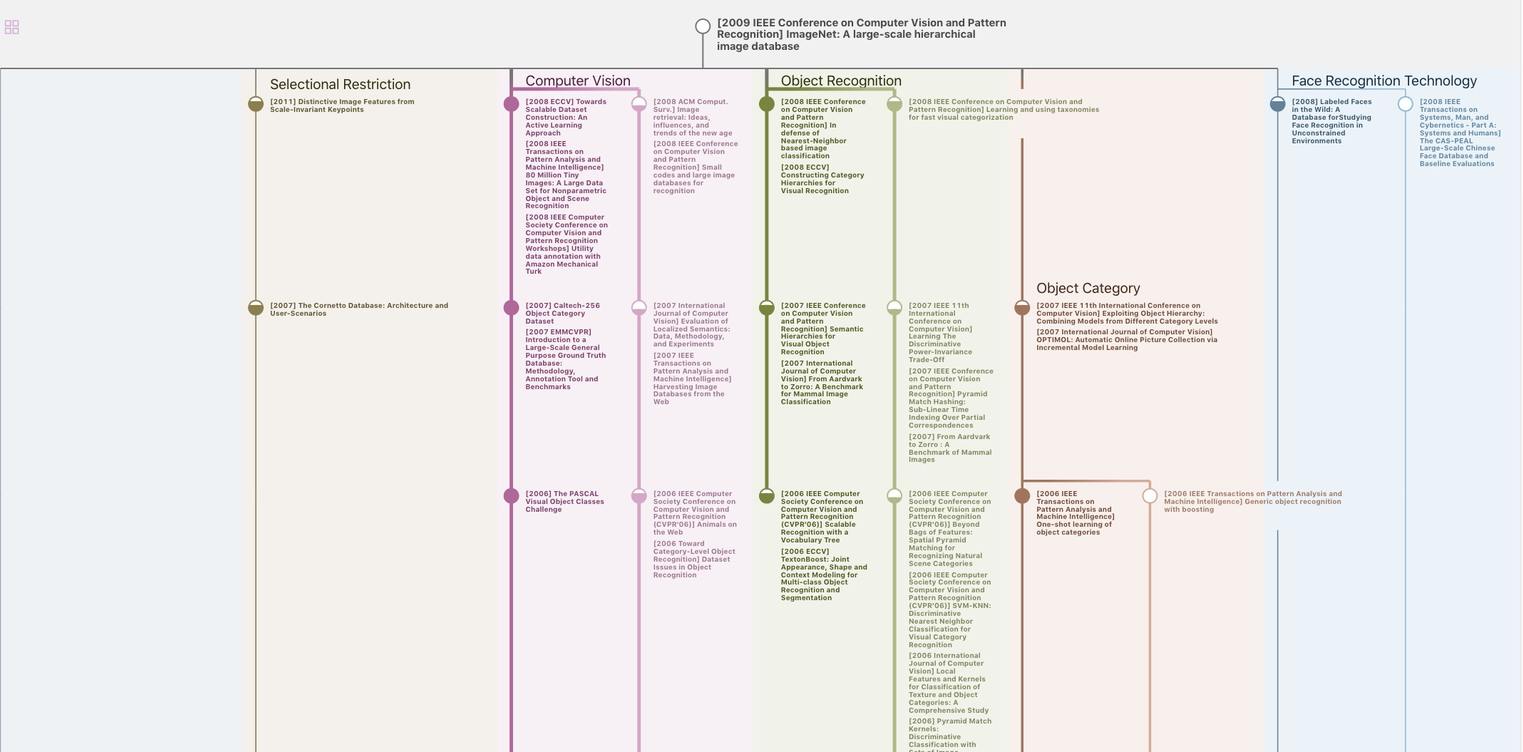
生成溯源树,研究论文发展脉络
Chat Paper
正在生成论文摘要