Deep Learning Techniques of Fatty Liver Using Multi-view Ultrasound Images scanned by Different Scanners (Preprint)
semanticscholar
摘要
BACKGROUND
Fat fraction values obtained from magnetic resonance images (MRI) can be used to obtain an accurate diagnosis of fatty liver diseases. However, MRI is expensive and cannot be performed for everyone.
OBJECTIVE
In this study, we aim to develop multi-view ultrasound image-based convolutional deep learning models to detect fatty liver disease and yield fat fraction values.
METHODS
We extracted 90 (the right intercostal view) and 90 (the right intercostal view containing the right renal cortex) ultrasound images from 39 fatty liver subjects (MRI-PDFF ≥ 5%) and 51 normal subjects (MRI-PDFF < 5%) containing MRI-PDFF values from Good Gang-An Hospital. We combined liver and kidney-liver (CLKL) images to train the deep learning models, and developed classification and regression models based on VGG19 to classify fatty liver disease and yield fat fraction values. We employed the data augmentation techniques such as flip and rotation to prevent the deep learning model from overfitting. We determined the deep learning model with performance metrics such as accuracy, sensitivity, specificity, and coefficient of determination (R2).
RESULTS
In demographic information, all metrics such as age and sex were similar between the two groups, i.e., fatty liver disease and normal subjects. In classification, model trained on CLKL images achieved 80.1% accuracy, 86.2% precision, and 80.5% specificity to detect fatty liver disease. In regression, the predicted fat fraction values of the regression model trained on CLKL images correlated with MRI-proton density fat fraction (MRI-PDFF) values (R2, 0.633), indicating that the predicted fat fraction values were moderately estimated.
CONCLUSIONS
With deep learning techniques and multi-view ultrasound images, it is potentially possible to replace MRI-PDFF values with deep learning predictions for detecting fatty liver disease and estimating fat fraction values.
更多查看译文
AI 理解论文
溯源树
样例
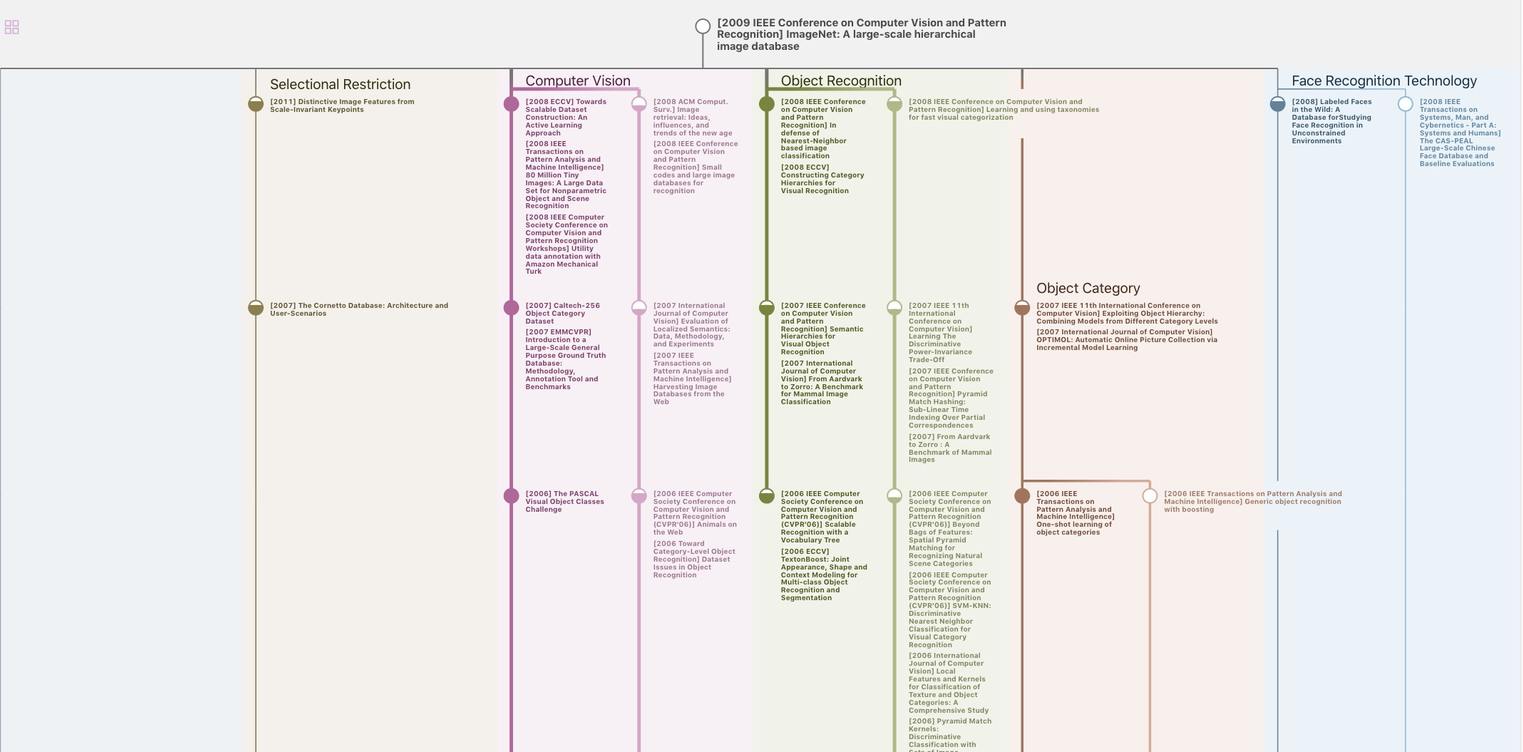
生成溯源树,研究论文发展脉络
Chat Paper
正在生成论文摘要