Functional Priors for Bayesian Neural Networks through Wasserstein Distance Minimization to Gaussian Processes
semanticscholar(2021)
摘要
The Bayesian treatment of neural networks dictates that a prior distribution is considered over the weight and bias parameters of the network. The non-linear nature of the model implies that any distribution of the parameters has an unpredictable effect on the distribution of the function output. Gaussian processes offer a rigorous framework to define prior distributions over the space of functions. Our proposal is to impose such functional priors on well-established architectures of neural networks by means of minimising the Wasserstein distance between samples of stochastic processes. Early experimental results demonstrate the potential of functional priors for Bayesian neural networks.
更多查看译文
AI 理解论文
溯源树
样例
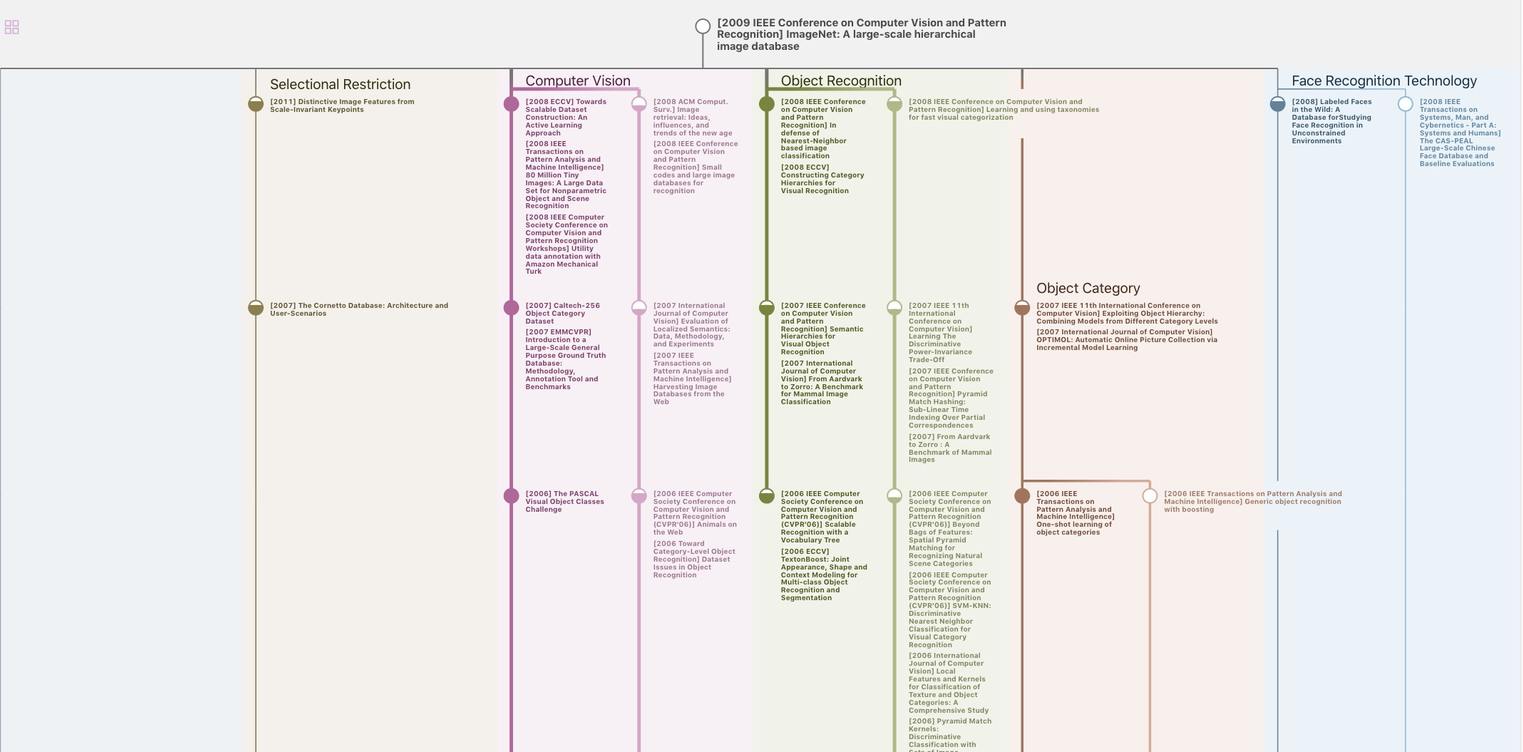
生成溯源树,研究论文发展脉络
Chat Paper
正在生成论文摘要