Implementation of ai/deep learning disruption predictor into a plasma control system
CONTRIBUTIONS TO PLASMA PHYSICS(2021)
摘要
State-of-the-art deep-learning disruption prediction models based on the Fusion Recurrent Neural Network (FRNN) introduced in the NATURE (2019) publication have been further improved. The paper reports on new capability of the AI/DL software to output not only the “disruption score,” as an indicator of the probability of an imminent disruption, but also a “sensitivity score” in real-time to indicate the underlying reasons for the imminent disruption. This provides valuable physics-interpretability for the deep-learning model results and associated guidance for control actuators when implemented into a modern Plasma Control System (PCS). The advance is a significant step forward in moving from modern DL disruption prediction to real-time control and brings novel AI-enabled capabilities relevant for application to the future burning plasma ITER system. Results presented used large amounts of data from JET and DIII-D vetted in the earlier NATURE publication. In addition to “when” a shot predicted to disrupt, this paper addresses reasons “why” by carrying out sensitivity studies. FRNN is accordingly extended to use many more channels of information, including measured DIII-D signals such as (i) “n1rms” that is correlated with the n =1 modes with finite frequency, including neoclassical tearing mode and sawtooth dynamics; (ii) the bolometer data indicative of plasma impurity content; and (iii) “q-min” – the minimum value of the safety factor relevant to the key physics of kink modes. When integrated into the deep learning FRNN software, clearer identification of physics responsible for the disruption events was enabled with associated relevant guidance for control actuators. In providing a “disruption score” together with a “sensitivity score” for each physics-connected channel, the present investigations of disruption subcategories for relevant physics channels can provide more precise and direct information for the control actuators in a plasma control system.
更多查看译文
关键词
artificial intelligence plus, Fusion Energy Science plus, machine learning plus, tokamak disruption prediction & control
AI 理解论文
溯源树
样例
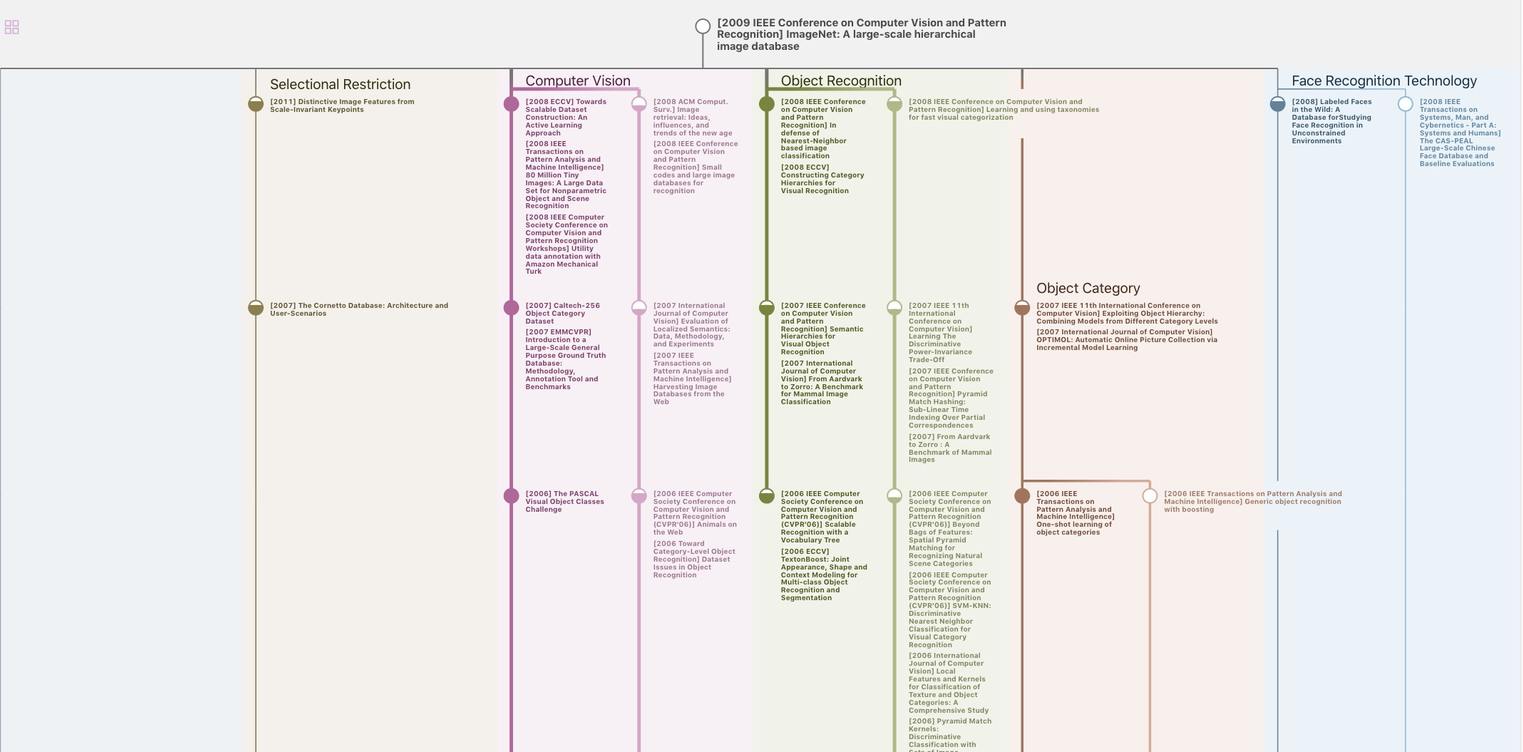
生成溯源树,研究论文发展脉络
Chat Paper
正在生成论文摘要