Learning directed acyclic graphs for ligands and receptors based on spatially resolved transcriptomic analysis of ovarian cancer
bioRxiv(2021)
摘要
A critical bottleneck step towards understanding the immune activation and suppression mechanisms in tumor samples is to identify transcriptional signals underlying the cell-cell communication between tumor cells and immune/stromal cells in tumor microenvironments (TME). Cell-to-cell communication extensively relies on interactions between secreted ligands and cell-surface receptors, which create a highly connected signaling network through many ligand-receptor paths. The latest advance in in situ omics analyses, such as spatial transcriptomic (ST) analysis, provide unique opportunities to directly characterize ligand-receptor signaling networks that powers cell-cell communication, which has not been feasible based on either bulk or single-cell omics data. In this paper, we focus on high grade serous ovarian cancer (HGSC), and propose a novel statistical method, DAGBagST, to characterize the ligand-receptor interaction networks between adjacent tumor and stroma cells in ovarian tumors based on spatial transcriptomic data. DAGBagST utilizes a directed acyclic graph (DAG) model with a novel approach to handle the zero-inflated distribution observed in the ST data. It also leverages existing ligand-receptor regulation databases as prior information, and employs a bootstrap aggregation strategy to achieve robust network estimation. We applied DAGBagST to ST datasets of tumor samples from four HGSC patients, and identified common and distinct ligand-receptor regulations between adjacent tumor and stromal grids across multiple tumors. These results cast light on biological processes relating to the communication between tumor and TME cells in these ovarian tumors. An open-source R package for performing DAGBagST will be shared upon publication.
更多查看译文
关键词
acyclic graphs,transcriptomic analysis,receptors,ligands
AI 理解论文
溯源树
样例
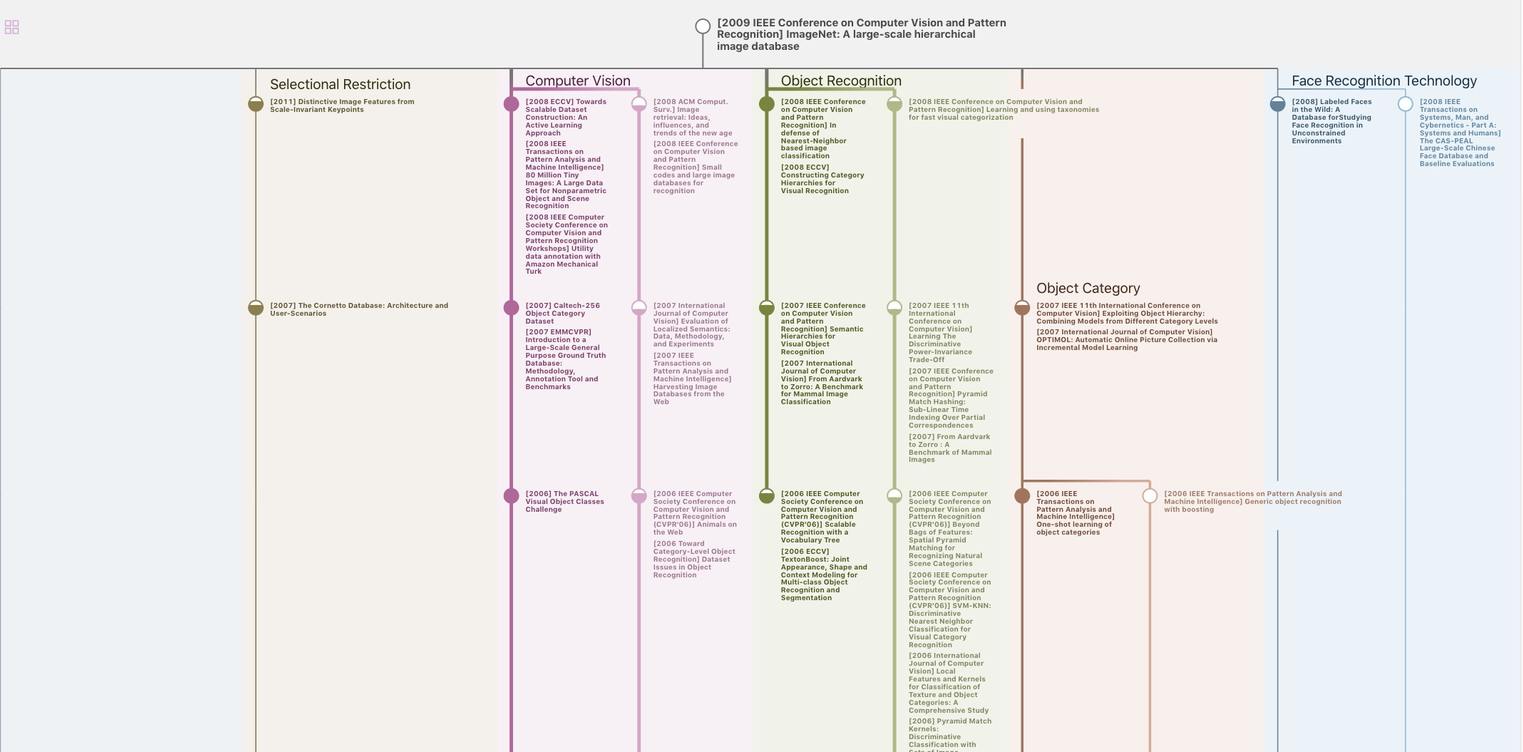
生成溯源树,研究论文发展脉络
Chat Paper
正在生成论文摘要