Identification and prediction of ALS subgroups using machine learning
medRxiv(2021)
摘要
Background The disease entity known as amyotrophic lateral sclerosis (ALS) is now known to represent a collection of overlapping syndromes. A better understanding of this heterogeneity and the ability to distinguish ALS subtypes would improve the clinical care of patients and enhance our understanding of the disease. Subtype profiles could be incorporated into the clinical trial design to improve our ability to detect a therapeutic effect. A variety of classification systems have been proposed over the years based on empirical observations, but it is unclear to what extent they genuinely reflect ALS population substructure. Methods We applied machine learning algorithms to a prospective, population-based cohort consisting of 2,858 Italian patients diagnosed with ALS for whom detailed clinical phenotype data were available. We replicated our findings in an independent population-based cohort of 1,097 Italian ALS patients. Findings We found that semi-supervised machine learning based on UMAP applied to the output of a multi-layered perceptron neural network produced the optimum clustering of the ALS patients in the discovery cohort. These clusters roughly corresponded to the six clinical subtypes defined by the Chio classification system (bulbar ALS, respiratory ALS, flail arm ALS, classical ALS, pyramidal ALS, and flail leg ALS). The same clusters were identified in the replication cohort. A supervised learning approach based on ensemble learning identified twelve clinical parameters that predicted ALS clinical subtype with high accuracy (area under the curve = 0.94). Interpretation Our data-driven study provides insight into the ALS population's substructure and demonstrates that the Chio classification system robustly identifies ALS subtypes. We provide an interactive website (https://share.streamlit.io/anant-dadu/machinelearningforals/main) so that clinical researchers can predict the clinical subtype of an ALS patient based on a small number of clinical parameters. Funding National Institute on Aging and the Italian Ministry of Health.
更多查看译文
关键词
als subgroups,machine learning,prediction
AI 理解论文
溯源树
样例
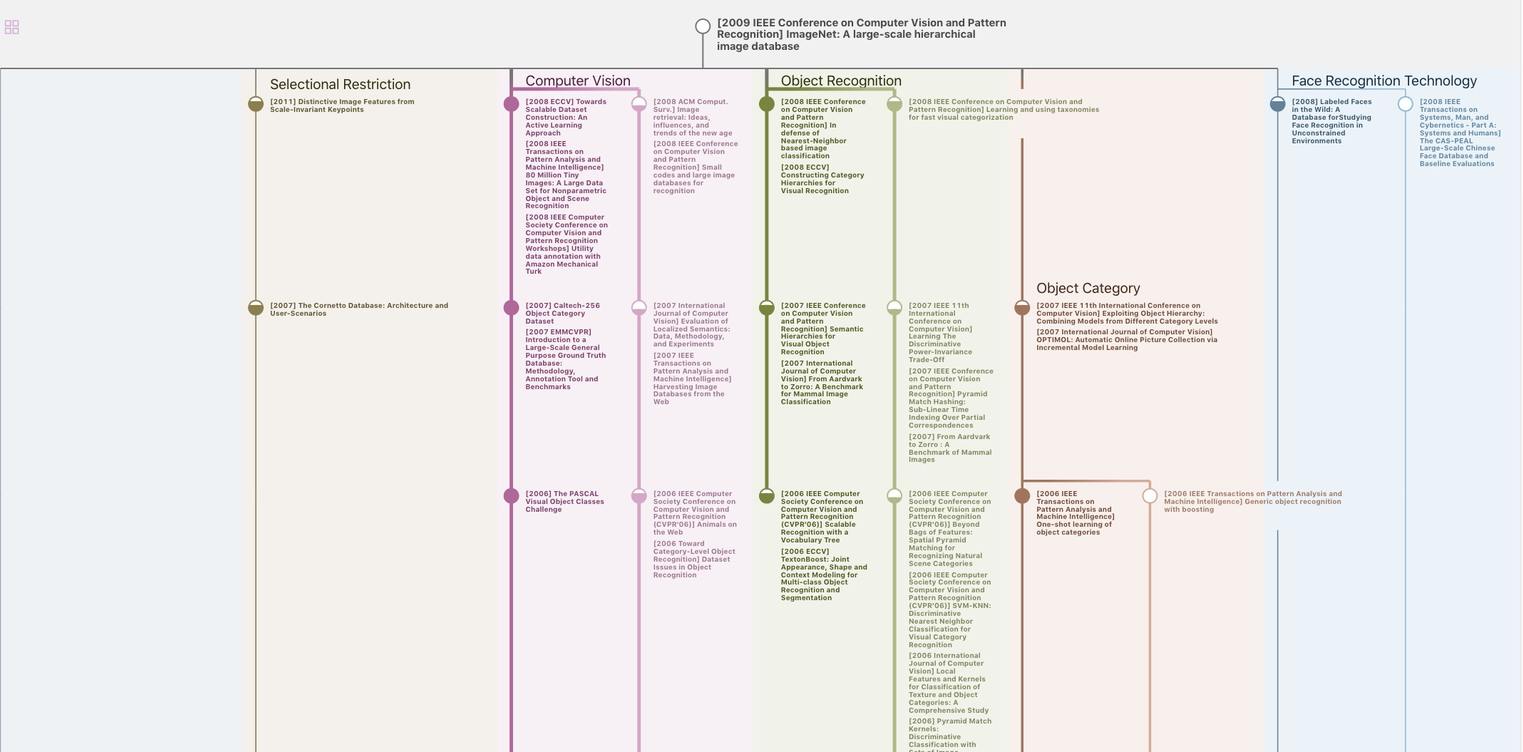
生成溯源树,研究论文发展脉络
Chat Paper
正在生成论文摘要