Leveraging fine-mapping and non-European training data to improve trans-ethnic polygenic risk scores
medRxiv(2021)
摘要
Polygenic risk scores (PRS) based on European training data suffer reduced accuracy in non-European target populations, exacerbating health disparities. This loss of accuracy predominantly stems from LD differences, MAF differences (including population-specific SNPs), and/or causal effect size differences. Here, we propose PolyPred, a method that improves trans-ethnic polygenic prediction by combining two complementary predictors: a new predictor that leverages functionally informed fine-mapping to estimate causal effects (instead of tagging effects), addressing LD differences; and BOLT-LMM, a published predictor. In the special case where a large training sample is available in the non-European target population (or a closely related population), we propose PolyPred+, which further incorporates the non-European training data, addressing MAF differences and causal effect size differences. We applied PolyPred to 49 diseases and complex traits in 4 UK Biobank populations using UK Biobank British training data (average N=325K), and observed statistically significant average relative improvements in prediction accuracy vs. BOLT-LMM ranging from +7% in South Asians to +32% in Africans (and vs. LD-pruning + P-value thresholding (P+T) ranging from +77% to +164%), consistent with simulations. We applied PolyPred+ to 23 diseases and complex traits in UK Biobank East Asians using both UK Biobank British (average N=325K) and Biobank Japan (average N=124K) training data, and observed statistically significant average relative improvements in prediction accuracy of +24% vs. BOLT-LMM and +12% vs. PolyPred. In conclusion, PolyPred and PolyPred+ improve trans-ethnic polygenic prediction accuracy, ameliorating health disparities.
更多查看译文
AI 理解论文
溯源树
样例
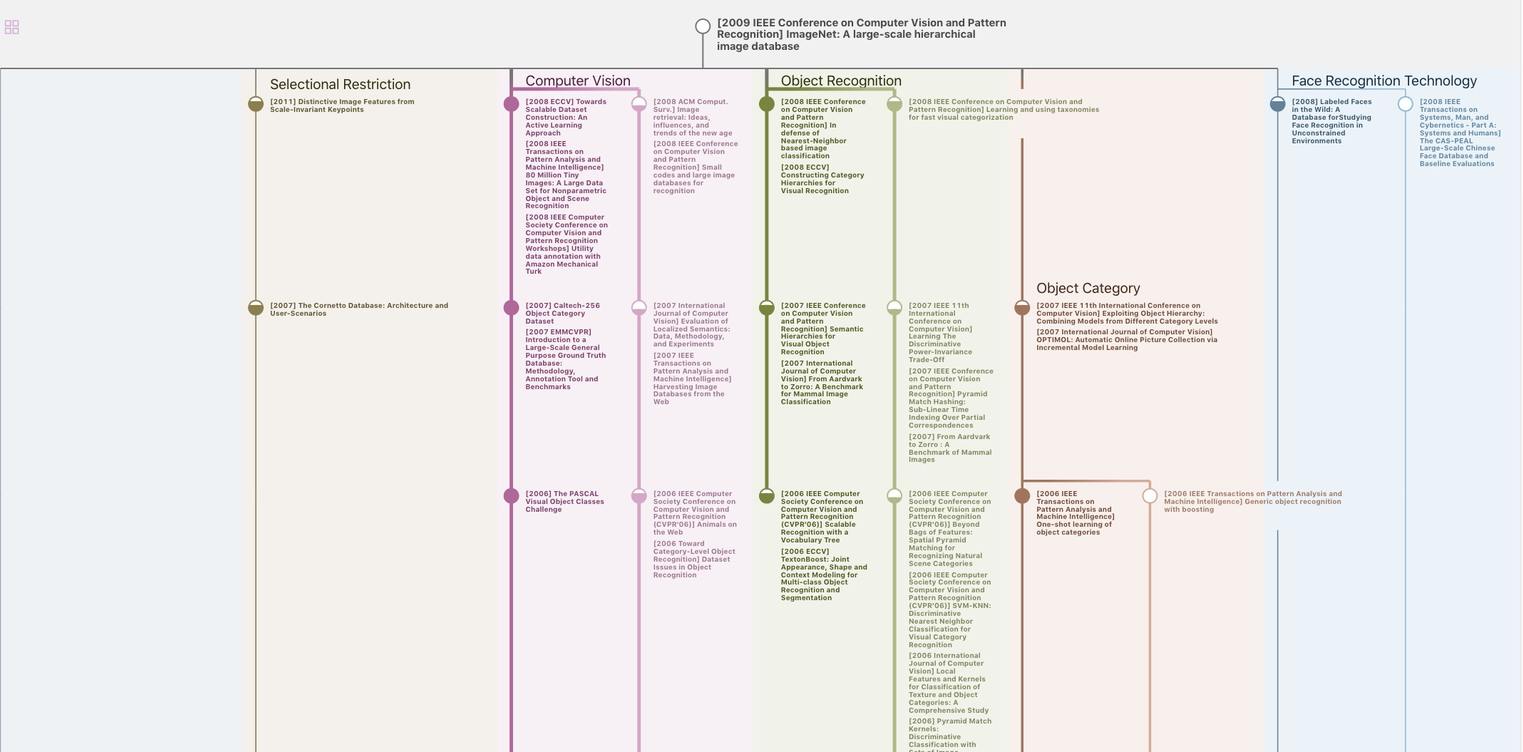
生成溯源树,研究论文发展脉络
Chat Paper
正在生成论文摘要