C-SemiSeg: Contrastive Semi-supervised Segmentation via Cross-set Learning and Dynamic Class-balancing
semanticscholar(2021)
摘要
Region-level data mixing. As stated in our main text, we perform region-level data mixing among labeled and unlabeled data using CutMix [3] operation. Specifically, we fix the rectangle region’s size to the half of the inputs and vary the aspect ratio and the position for each mixing pair. Although the mixing image does not exist in the real world, it is reasonable to consider it as a kind of augmentation technique for training, which is similar to the random crop operation. Besides, experiments show that conducting cross-set region-level data mixing enriches not only the diversity of unlabeled samples but also deduces the feature misalignment between labeled and unlabeled set. Overall augmentation strategy. In a semi-supervised learning setting, we apply the asymmetric data augmentation strategy for the mean teacher network. Specifically, weak augmentation is conducted on the labeled data, as well as the unlabeled data for the teacher’s input. The strong augmentation with Auto Augmentation1 is applied to the unlabeled data to generate the student’s input. We summarize the operations in Table 1.
更多查看译文
AI 理解论文
溯源树
样例
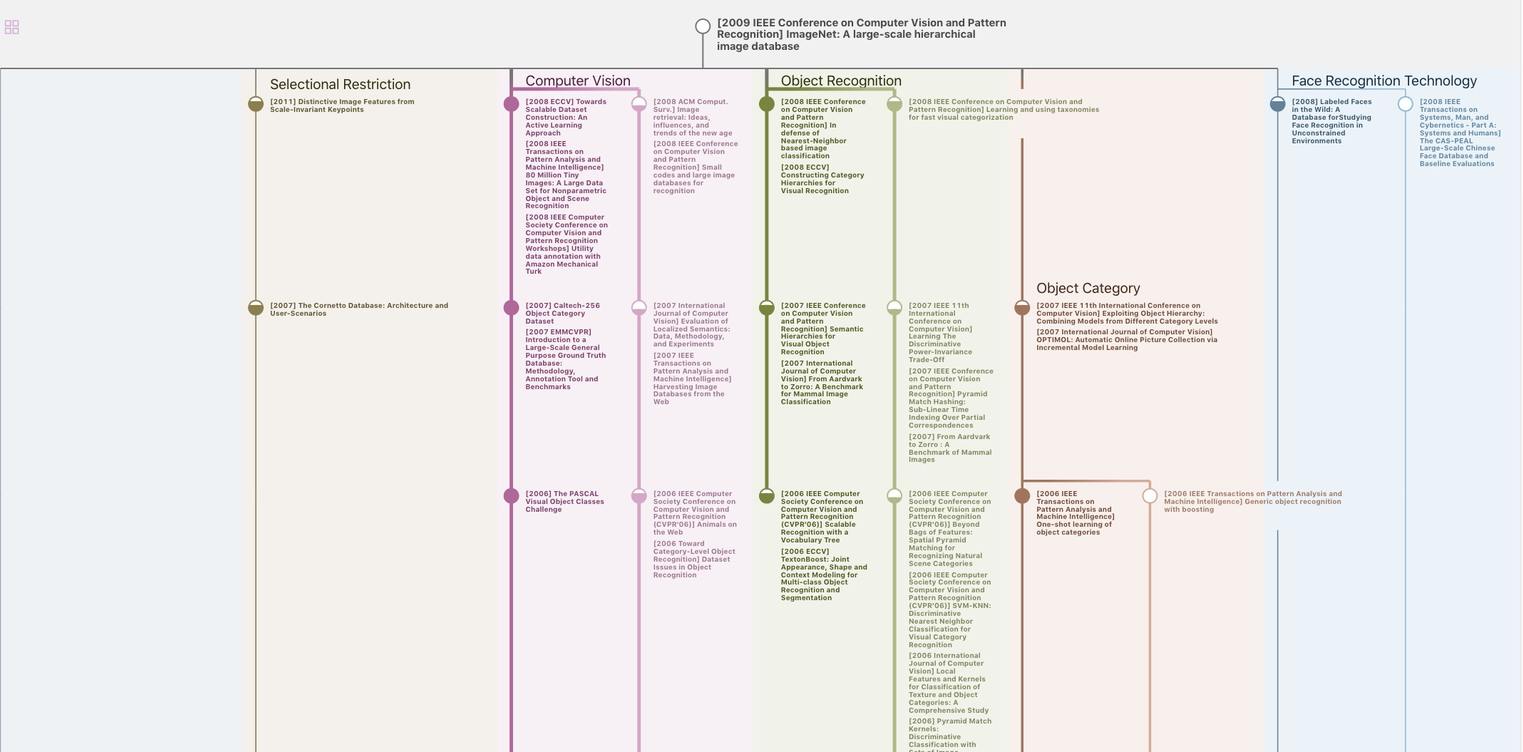
生成溯源树,研究论文发展脉络
Chat Paper
正在生成论文摘要