AdamDGN: Adaptive Memory using Dynamic Graph Networks for Staleness Problem in Recommender System
semanticscholar(2021)
摘要
Graph Neural Networks (GNNs) have proved their effectiveness in various recommendation tasks with their ability to incorporate relational information. However, a staleness problem in a recommendation task has been less explored in the literatures on graph learning, evaluating their performances on datasets with unrealistic distribution of users. In practical applications, ratio of "cool" users, who cool down to a service, dominates that of loyal users yielding an extreme sparsity problem for recommender systems. In this paper, we bring DeepCluster strategy to a memory-based temporal graph model for an online adaptive graph learning method, AdamDGN, that allows all nodes to be adaptively updated as new events are introduced, irrespective of their involveness. We evaluated on Amazon product review datasets, and AdamDGN outperforms all other baselines with significant margins on both two datasets.
更多查看译文
AI 理解论文
溯源树
样例
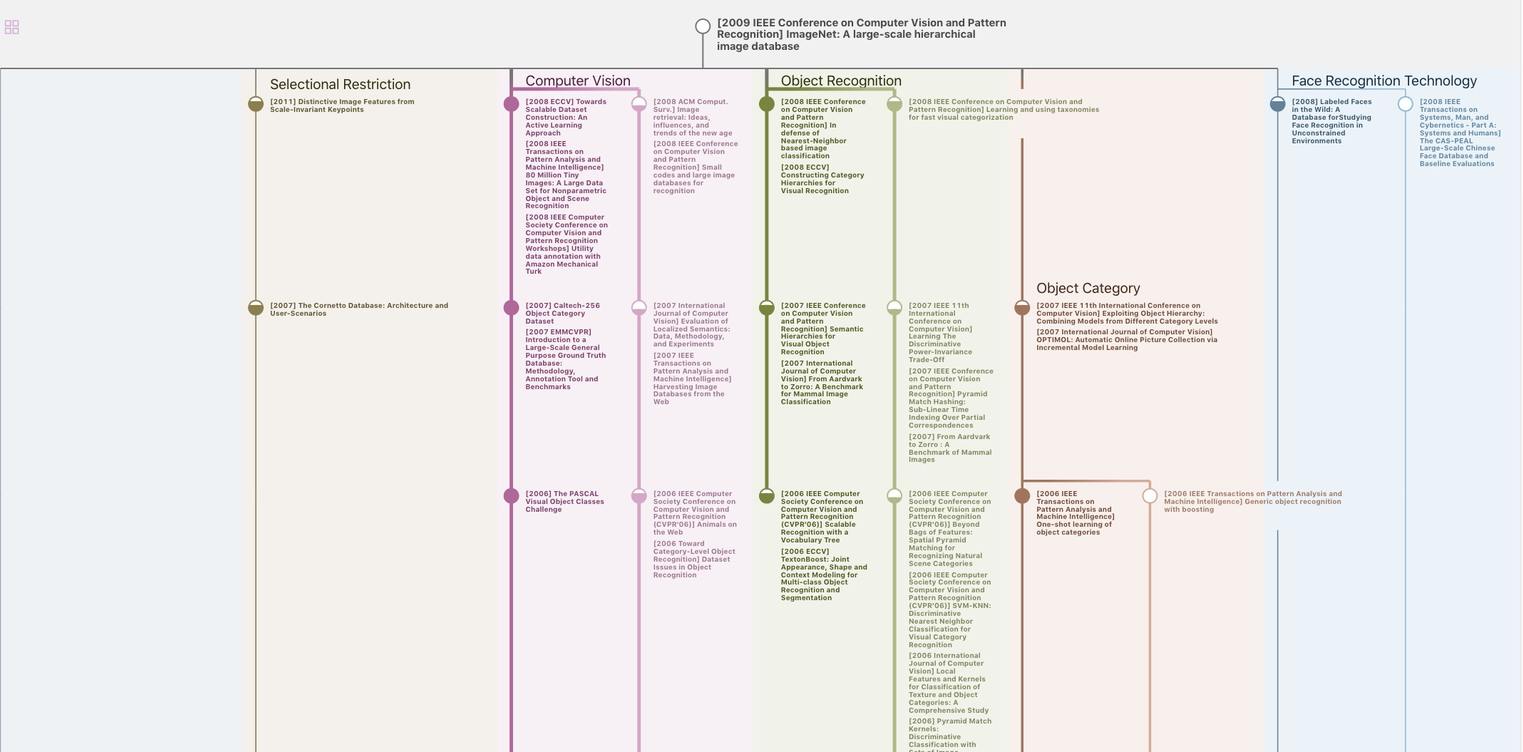
生成溯源树,研究论文发展脉络
Chat Paper
正在生成论文摘要