Proofs of Conservative Confidence Bounds on PFD, Using Claims of Improved Reliability
semanticscholar(2021)
摘要
We derive infima for posterior confidence in bounds on system pfd, subject to various constraints – called “prior knowledge” – on joint prior distributions. This concerns confidence bounds on the pfd for a system B, upon observing two systems, A and B, operate without failure. In particular, the results hold when evidence supports a claim of B being as reliable, or better, than A. The propositions proved in this technical report are motivated, explained and discussed in the paper “Conservative Confidence Bounds in Safety, from Generalised Claims of Improvement & Statistical Evidence”, reported at the 51st IEEE/IFIP DSN conference. INTRODUCTION This report derives conservative confidence bounds on the probability of failure on demand (pfd) for a system. Let XA and XB be the unknown pfds of systems A and B. Consider the following 4 forms of “prior knowledge” that each constrain the joint prior distribution of 〈XA, XB〉. We refer to these constraints as PK 1, 2, 3 and 4 respectively. Prior Knowledge 1. certainty that the system pfd X is no better than some pl > 0. That is, P (X > pl) = 1 . Prior Knowledge 2. θ×100% confidence that the system pfd X meets, or surpasses, a pfd ε. That is, P (X 6 ε) = θ. Prior Knowledge 3. confidence in version A’s pfd being α or better, and in the B version being an improvement: P (XB 6 XA, XA 6 α) = φ (1) where ε 6 α 6 1 and 0 < φ < 1. In particular, φ is defined as the value of φ when α = 1. Prior Knowledge 4. confidence in version A’s pfd falling within some range of values, and version B being an improvement: for some sub-interval I of [0, 1], with φ as just defined, P (XB 6 XA, XA ∈ I) = φ 1− φ (XA < XB , XA ∈ I) (2) In particular, we consider the case when such a requirement holds for the two intervals [pl 6 XA 6 ε], [α 6 XA 6 1] and, thus (as probabilities must add up to 1), also holds for [ε < XA < α]. Let two independent Bernoulli processes characterise the occurrence of failures for systems A and B. If systems A and B experience no failures, respectively, on a sequence of nA and nB independent demands, then the posterior probability that [XB 6 p] for some p is P (XB6p | nA, nB) = E[L(XA, XB)1XB6p ] E[L(XA, XB) ] (3) where L(x, y) = (1−x)A(1−y)B is the likelihood function, and 1S is an indicator function – it equals 1 when predicate S is true, and 0 otherwise. Let D be the set of all probability distributions over the unit square. The following two propositions are constrained optimisation problems that give the infima (i.e. greatest lower bounds) for (3) under different circumstances. Solving these problems entails determining preferred joint prior distributions with P (XB < p | nA, nB) equal to the relevant infimum. Each optimisation problem is solved subject to PK constraints and certain parameter ranges of θ, φ and φ. The solutions illustrate how to solve analogous optimisation problems for other parameter ranges, using similar solution steps. Proposition 1. Consider the optimisation problem inf D P (XB 6 p | nA, nB) where ε 6 p, subject to systems A, B satisfying PK 1, 2, 3. Fig. 1 shows a prior distribution that solves this problem when φ > θ > 1− θ, ε < α 6 p. The infimum is Num Num+ L(pl, p)(1− φ) (4) where Num := L(α, α)(φ − θ) + L(ε, ε)(φ + θ − 1) + L(α, ε)(1 − φ). This is the value of P (XB < p | nA, nB) computed using this prior distribution. Proposition 2. Consider the optimisation problem inf D P (XB 6 p | nA, nB) where ε 6 p, subject to systems A, B satisfying PK 1, 2, 4. Fig. 2 shows a prior distribution that solves this problem when φ > θ, p < α < 1 . The infimum is L(ε, ε)φθ L(ε, ε)φ2θ +Den (5)
更多查看译文
AI 理解论文
溯源树
样例
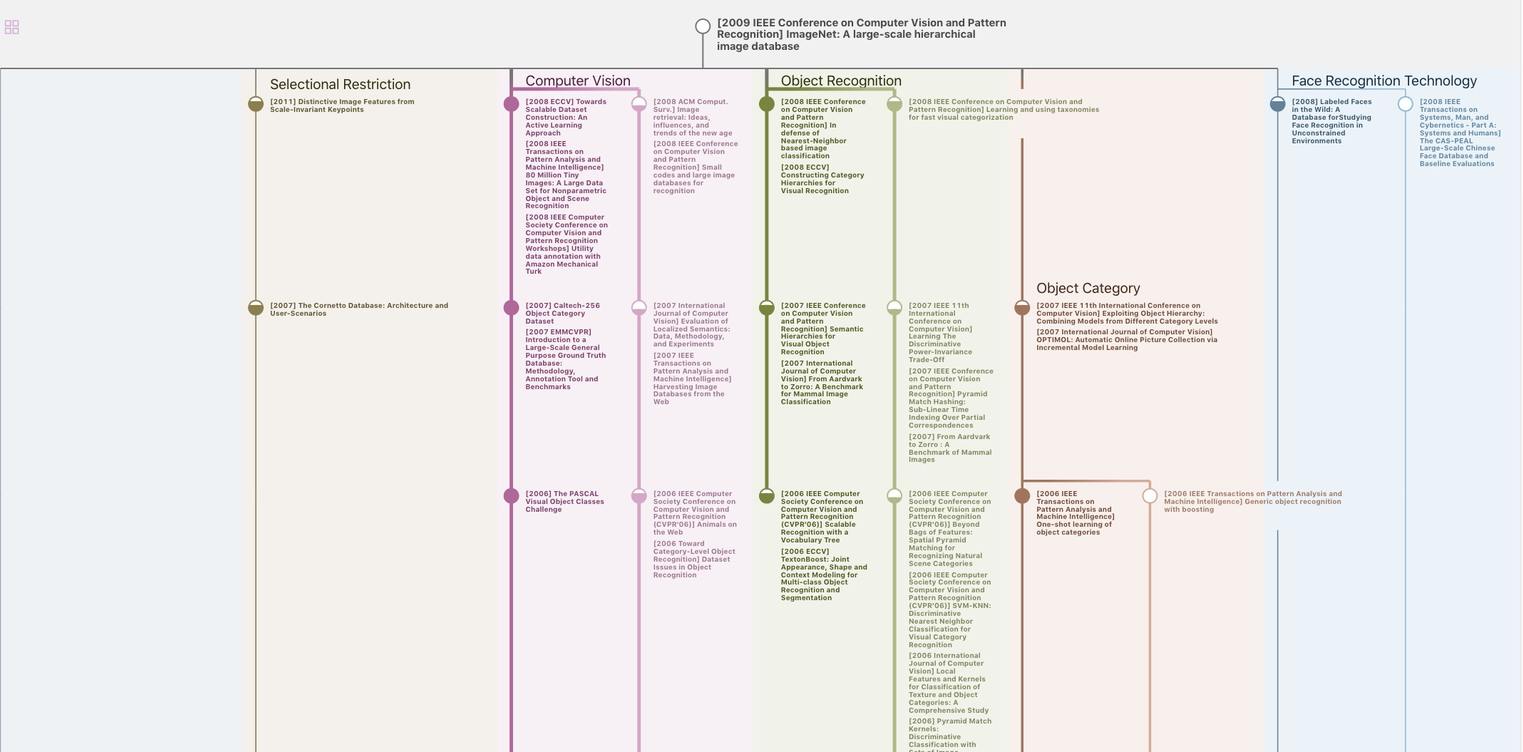
生成溯源树,研究论文发展脉络
Chat Paper
正在生成论文摘要