Prediction of vacancy formation energies at tungsten grain boundaries from local structure via machine learning method
Journal of Nuclear Materials(2022)
摘要
•A framework for predicting vacancy formation energy (EVf) near symmetry tilt grain boundaries in tungsten (W) by machine learning technique was presented.•The geometrical factors, such as spatial size characteristic, density and location, were determined as descriptors for the machine learning analysis.•Three separate cross validation (CV) predictions for high angle, low angle-I and low angle-II STGBs have obvious improvement in accuracies compared to all-data CV.•The present method is robust and general to other materials such as α-Fe, and beneficial to predict and understand the vacancy formation near interfaces.
更多查看译文
关键词
Vacancy formation energy,Machine learning,Tungsten,Symmetry tilt grain boundary,Support vector machine,Cross validation
AI 理解论文
溯源树
样例
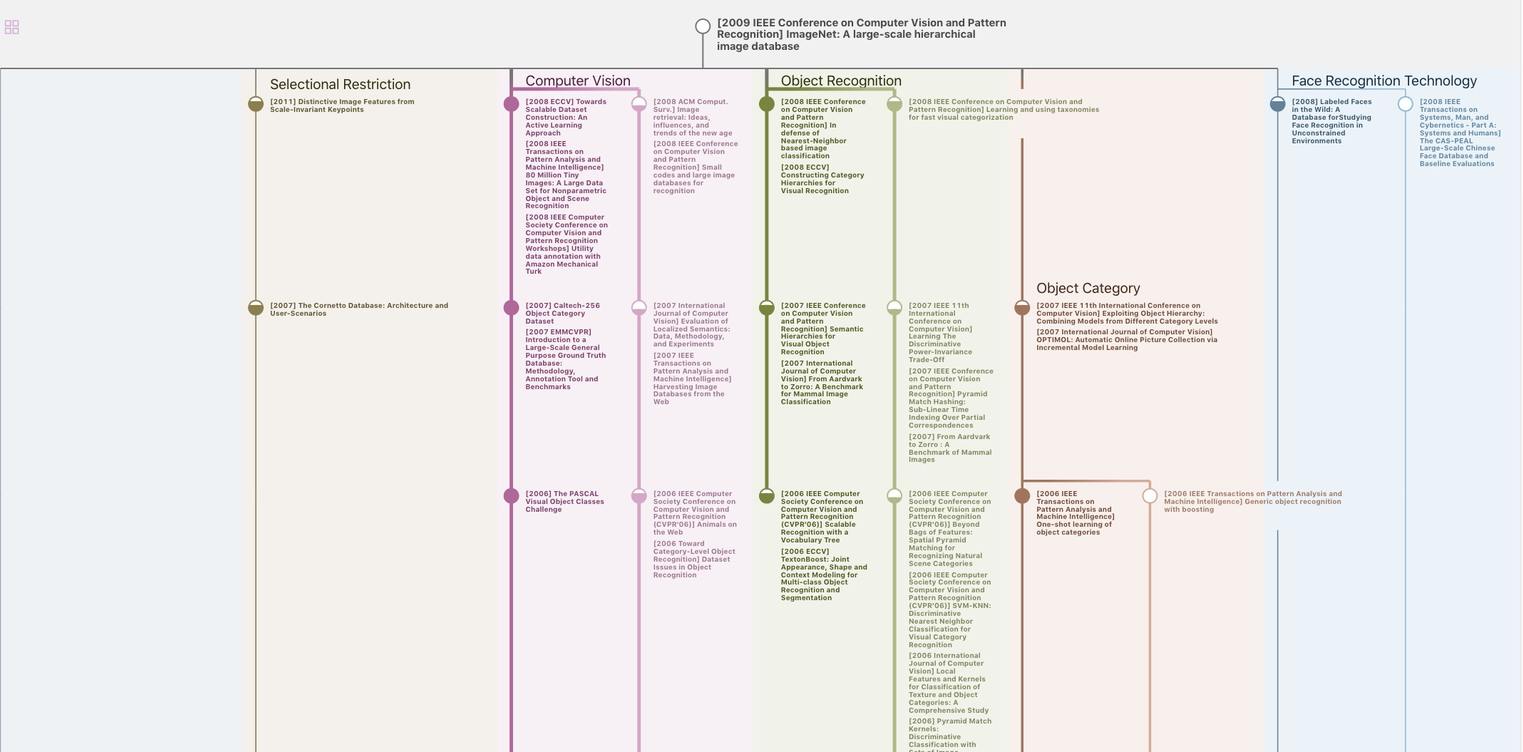
生成溯源树,研究论文发展脉络
Chat Paper
正在生成论文摘要