Simultaneous classification and location of deformation in SAR interferograms using deep learning
semanticscholar(2021)
摘要
This manuscript is a non-peer reviewed EarthArXiv preprint that has been submitted for publication in Remote Sensing of Environment. With the evolution of InSAR into a tool for active hazard monitoring, through its ability to detect ground deformation with low latency, new methods are sought to quickly and automatically interpret the large number of interferograms that are created. In this work, we present a convolutional neural network (CNN) that is able to both classify the type of deformation, and to locate the deformation within an interferogram in a single step. We achieve this through building a “two headed model”, which is able to return both outputs after one forward pass of an interferogram though the network, and so does not require the use of a sliding window approach for localisation. We train our model by first creating a large dataset of synthetic interferograms which feature labels of both the type and location of any deformation, but also find that our model’s performance is improved through the inclusion of just a small amount of real data. When building models Preprint submitted to Remote Sensing of Environment January 11, 2021 of this type, it is common for some of the weights within the model to be transferred from other models designed for different problems. Consequently, we also investigate how to best organise interferograms such that the filters learned in models such as VGG16 are sensitive to the signals of interest in interferograms, but find that using different data in each of the three input channels significantly degrades performance when compared to the simple case of repeating (un)wrapped phase across each channel. This implies that the inclusion of supplementary data, which we expect should improve the ability to distinguish deformation from noise, requires training of a network from scratch.
更多查看译文
AI 理解论文
溯源树
样例
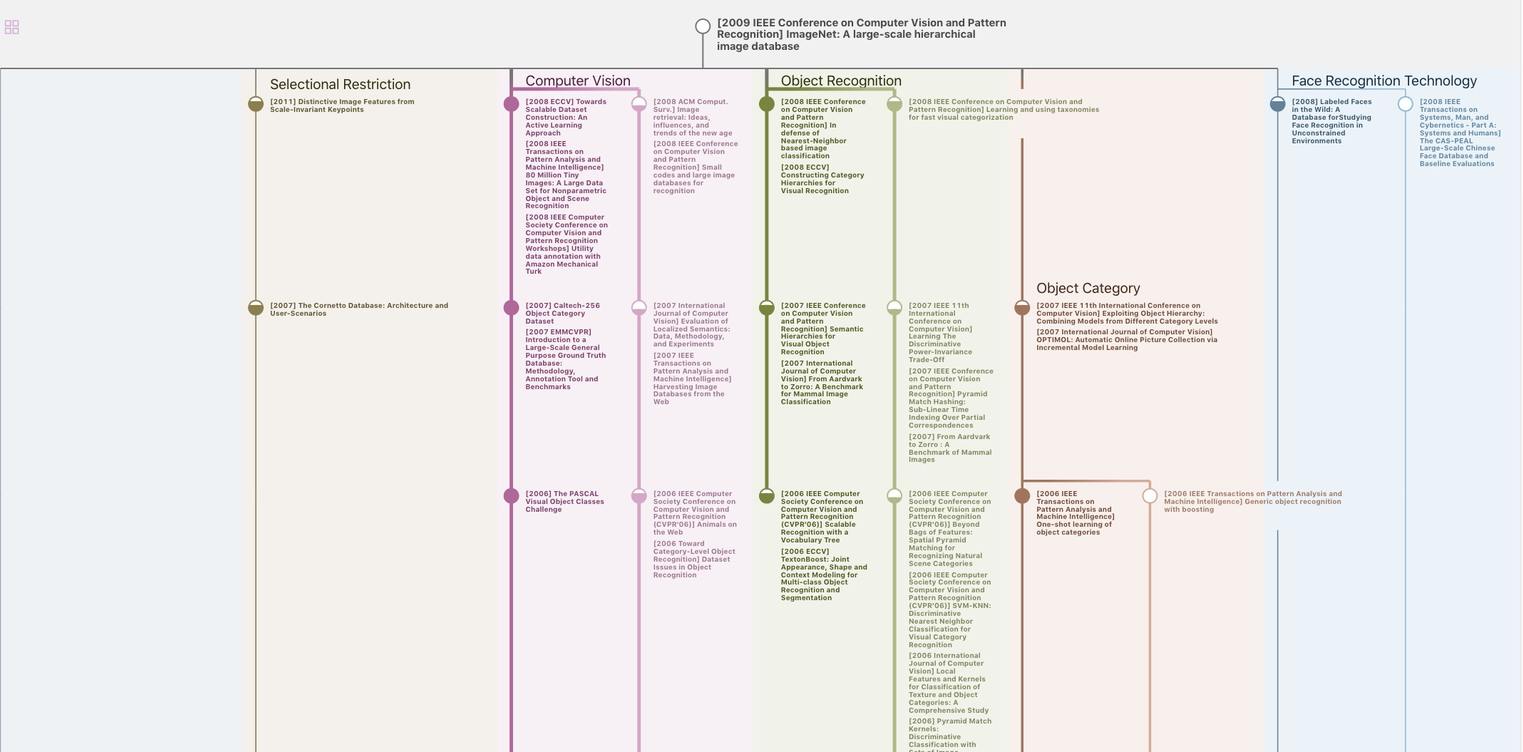
生成溯源树,研究论文发展脉络
Chat Paper
正在生成论文摘要