Face Stereotypes from Insufficient Statistical Learning
semanticscholar(2021)
摘要
Social stereotypes are prevalent and consequential, yet sometimes inaccurate. How do people learn these inaccurate beliefs in the first place and why do these beliefs persist in the face of counter evidence? Building on past research on cognitive limitations and environmental sample biases, we propose an integrative perspective: Insufficient statistical learning (Insta-learn). Instalearn posits that humans are active learners of the environment. Starting from a small sample, people are able to extract statistical patterns within the sample accurately and quickly. However, people do not continue sampling sufficiently. If they decide not to collect more samples once they are (prematurely) satisfied, inaccurate stereotypes can emerge even when more data would show otherwise. We investigated this hypothesis across six online experiments (N = 1565), using novel pairs of computer-generated faces and social behaviors. Fixing the population level statistics of face-behavior associations to zero and varying the initial sample statistics, we found that participants quickly learned the initial sample statistics (from as few as three examples) and persisted in using such spurious associations in their final decisions. Granting the sampling power to participants — samples were endogenously generated by participants and not defined by the experimenters — we found insufficient sampling caused spurious associations to persist. Insta-learn provides a domain-general framework for a mechanistic explanation of the emergence and persistence of social stereotypes.
更多查看译文
AI 理解论文
溯源树
样例
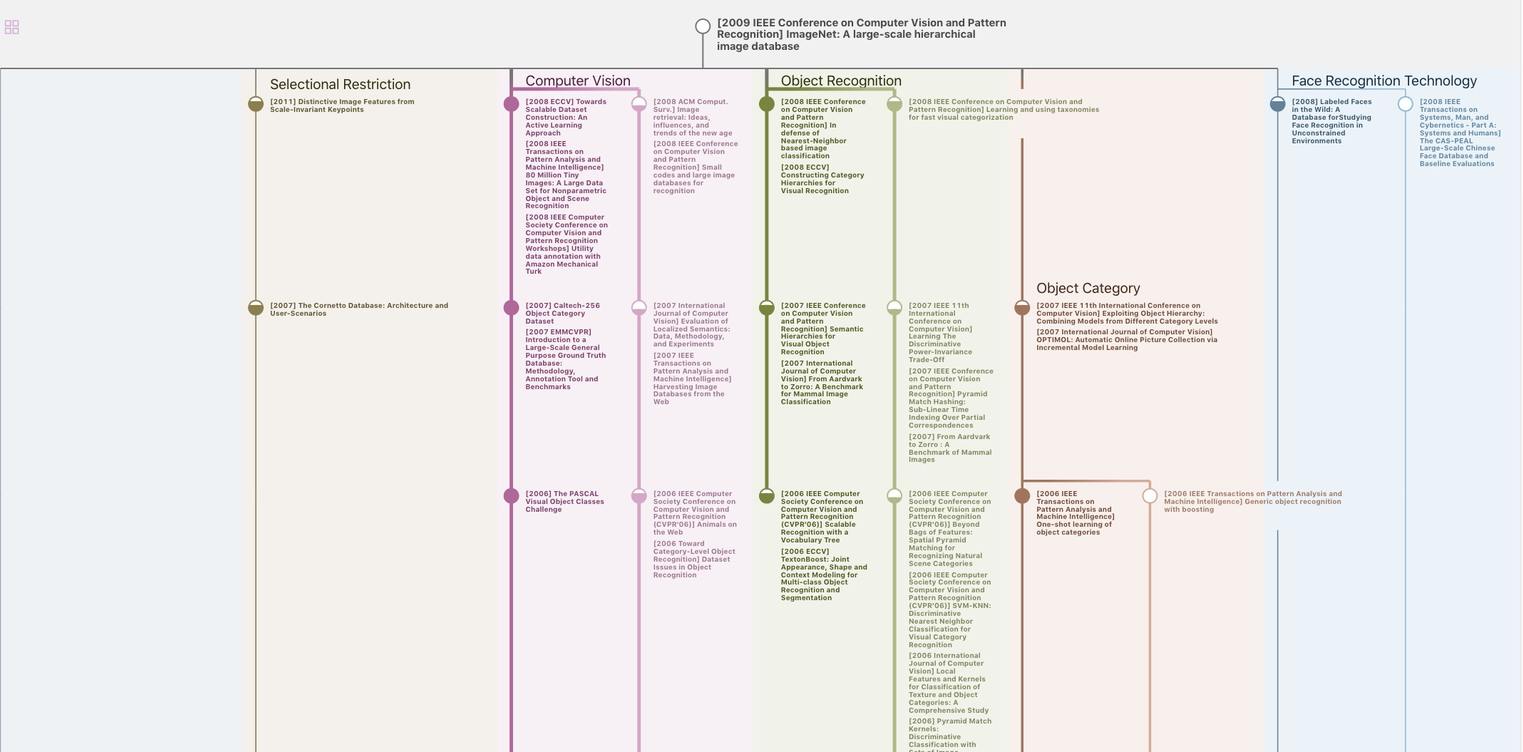
生成溯源树,研究论文发展脉络
Chat Paper
正在生成论文摘要