Measuring the u.s. employment situation using online panels: the yale labor survey
semanticscholar(2021)
Abstract
This study presents the design and results of a rapid-fire survey that collects labor market data for individuals in the United States. The purpose is to test online panels for their application to social, economic, and demographic information as well as to apply this approach to the U.S. labor market. The Yale Labor Survey (YLS) used an online panel from YouGov to replicate statistics from the Current Population Survey (CPS), the government’s official source of household labor market statistics. The YLS’s advantages included its timeliness, low cost, and ability to develop new questions quickly to study unusual labor market patterns during the COVID-19 pandemic. Results from the YLS track employment data closely from the CPS during the pandemic. Although YLS estimates of unemployment and participation rates mirrored the broad trends in CPS data, YLS estimates of those two rates were less accurate than for employment. The study demonstrates the power of carefully crafted online surveys to replicate expensive traditional methods quickly and inexpensively. 1 The authors of this report are Christopher Foote, senior economist and policy adviser, Federal Reserve Bank of Boston; Tyler Hounshell, Tobin Predoctoral Program, Yale University; William Nordhaus, Sterling Professor of Economics, Yale University; Douglas Rivers, Professor of Political Science, Stanford University, and Chief Scientist at YouGov; and Pamela Torola, Tobin Predoctoral Program, Yale University. We thank William Bannick of YouGov for outstanding assistance with this project. Alan Gerber, Matthew Shapiro, and Jason Faberman provided helpful suggestions in the design and execution of the project. (File is YLS-Report041521a-text.docx.) 2 Corresponding author is William Nordhaus (william.nordhaus@yale.edu). 3 The survey received initial Yale IRB approval on April 10, 2020 and has received further approvals as it has been revised. Foote, Hounshell, Nordhaus, and Torola declare no financial conflicts of interest with the research. Rivers has a conflict of interest as an employee and shareholder in YouGov. The views expressed in this study are those of the authors and do not indicate concurrence by the Federal Reserve Bank of Boston, the principals of the Board of Governors, the Federal Reserve System, or of any of the organizations with which the authors are affiliated. The initial surveys were conducted by YouGov for their own research purposes, and the ones after April 15 were financed by the Tobin Center at Yale University, the Cowles Foundation at Yale University, the MacMillan Center at Yale University, the Federal Reserve Bank of Boston, the Lounsbery Foundation, and the Sloan Foundation. The present paper draws upon a preliminary report in Foote et al. (2020).
MoreTranslated text
求助PDF
上传PDF
AI Read Science
AI Summary
AI Summary is the key point extracted automatically understanding the full text of the paper, including the background, methods, results, conclusions, icons and other key content, so that you can get the outline of the paper at a glance.
Example
Background
Key content
Introduction
Methods
Results
Related work
Fund
Key content
- Pretraining has recently greatly promoted the development of natural language processing (NLP)
- We show that M6 outperforms the baselines in multimodal downstream tasks, and the large M6 with 10 parameters can reach a better performance
- We propose a method called M6 that is able to process information of multiple modalities and perform both single-modal and cross-modal understanding and generation
- The model is scaled to large model with 10 billion parameters with sophisticated deployment, and the 10 -parameter M6-large is the largest pretrained model in Chinese
- Experimental results show that our proposed M6 outperforms the baseline in a number of downstream tasks concerning both single modality and multiple modalities We will continue the pretraining of extremely large models by increasing data to explore the limit of its performance
Upload PDF to Generate Summary
Must-Reading Tree
Example
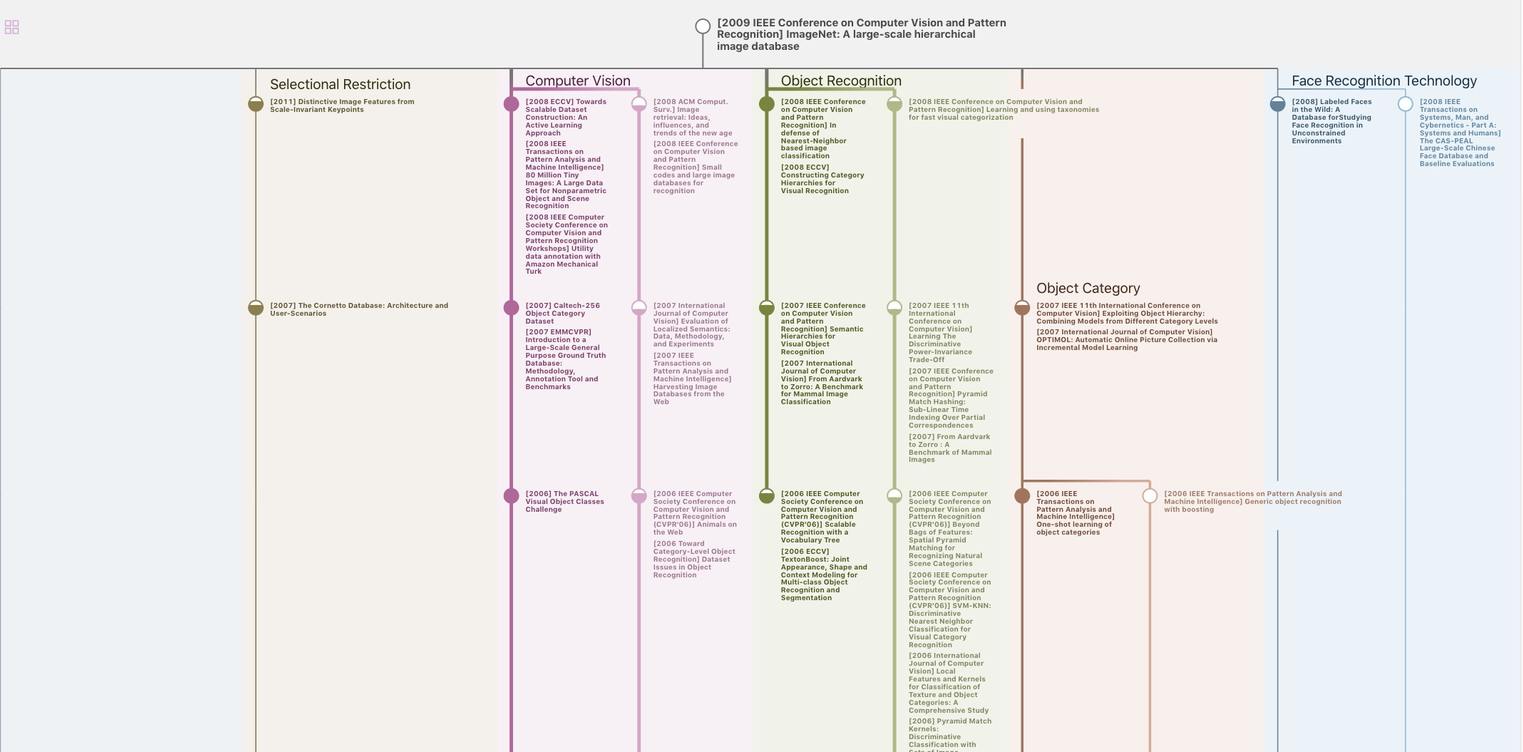
Generate MRT to find the research sequence of this paper
Related Papers
Data Disclaimer
The page data are from open Internet sources, cooperative publishers and automatic analysis results through AI technology. We do not make any commitments and guarantees for the validity, accuracy, correctness, reliability, completeness and timeliness of the page data. If you have any questions, please contact us by email: report@aminer.cn
Chat Paper