Cmar_a_326874 7951..7960
semanticscholar(2021)
摘要
1The Department of Radiology, The First Affiliated Hospital of Zhengzhou University, Zhengzhou, Henan Province, People’s Republic of China; 2Department of Radiology, Affiliated Cancer Hospital of Zhengzhou University & Henan Cancer Hospital, Zhengzhou, Henan Province, People’s Republic of China Objective: To explore the feasibility of the whole tumor histogram analysis parameters derived from dynamic contrast-enhanced MRI (DCE-MRI) based on stack-of stars (StarVIBE) to predict T and N staging of resectable gastric cancer (GC). Methods: Eighty-seven patients confirmed as GC by histopathology were enrolled in this prospective study. DCE-MRI were performed before surgery, and quantitative DCE parameters (K, Kep, Ve) and histogram metrics (Skewness, Kurtosis and Entropy) were measured by Omni-Kinetics software. Intraclass correlation coefficient (ICC) testing was used to determine the consistency of K, Kep and Ve values and histogram metrics values between two radiologists using Bland–Altman analysis. The quantitative DCE parameters or histogram metrics values between T stage or N stage were compared using ANOVA or Kruskal–Wallis testing. Receiver operating characteristic (ROC) analyses was performed to find out the best parameters for identifying T and N staging. Results: There was statistical difference in K, Kep, Ve and entropy to identify T staging (P=0.015, 0.033, <0.001, and 0.007, respectively), and in pairwise comparisons of Ve values showed statistically difference between T1+2 and T3 group (P<0.001), T1+2 and T4 group (P<0.001). There were statistical differences in Ve to identify N staging (P=0.041). In ROC analysis, Ve was the best parameter for identifying T staging (AUC: 0.788, the sensitivity and specificity was 0.929 and 0.578, respectively) and N staging (AUC: 0.590, the sensitivity and specificity was 0.714 and 0.899, respectively). Conclusion: The whole tumor histogram analysis parameters derived from StarVIBE DCEMRI may be able to quantitatively evaluate T and N staging of GC, so as to help clinical treatment decision optimization.
更多查看译文
AI 理解论文
溯源树
样例
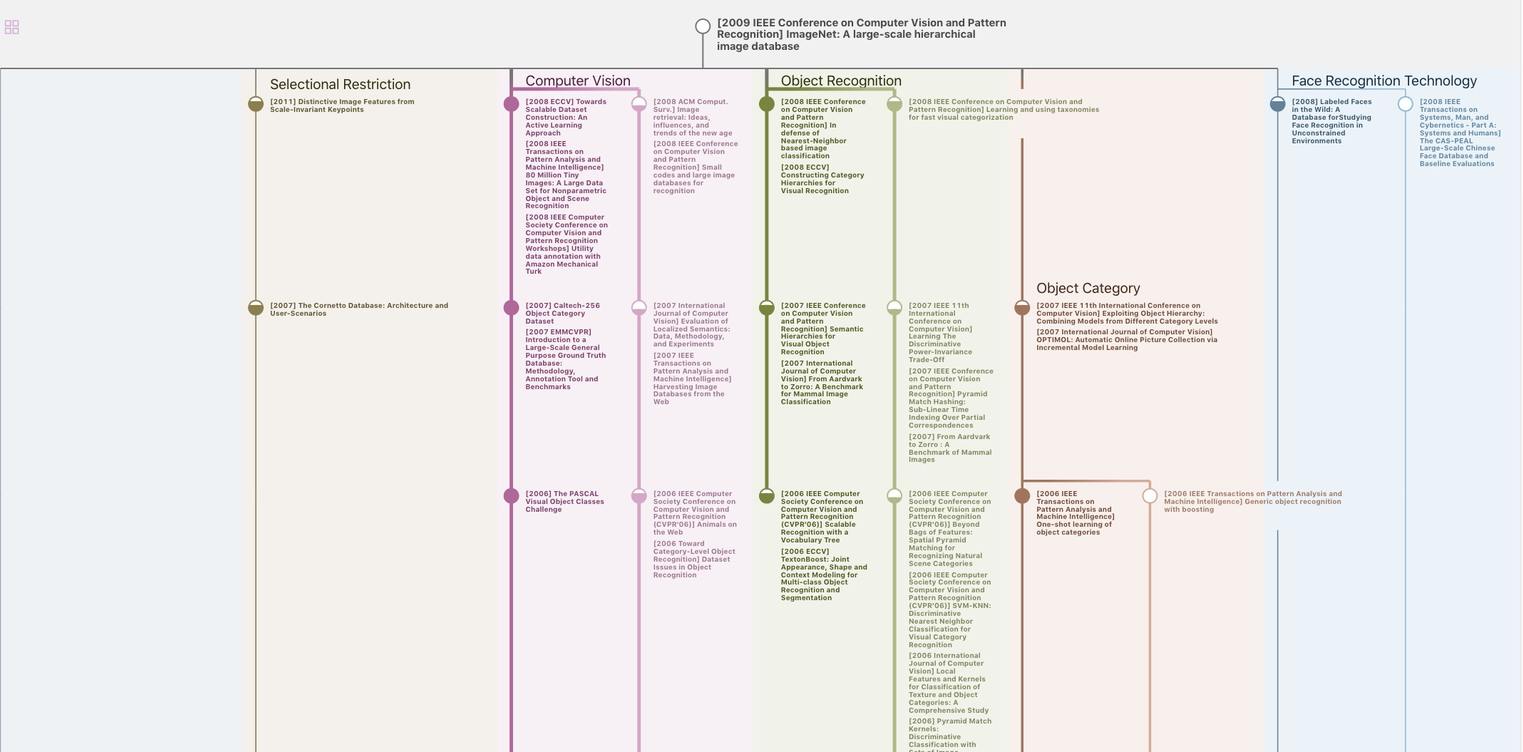
生成溯源树,研究论文发展脉络
Chat Paper
正在生成论文摘要