A comparison of Bayesian inference and gradient-based approaches for friction parameter estimation
semanticscholar(2020)
摘要
Numerical tidal models are essential to the study of a variety of coastal ocean processes, but typically rely on uncertain inputs, including a bottom friction parameter which can in principle be spatially varying. Here we employ an adjoint-capable numerical ocean model, Thetis, and apply it to the Bristol Channel and Severn Estuary, using a spatially varying Manning coefficient within the bottom friction parameterisation. The spatial variation in the coefficient is a priori constrained by a categorisation of the sediment type found on the sea bed into three groups: rock, sediment containing gravel, and sediment containing only sand. We compare two calibration methods to estimate the three corresponding Manning coefficients using tide gauge observation data. The first method consists of Bayesian inversion via a Markov Chain Monte Carlo algorithm, using a Gaussian process emulator as a surrogate for the full numerical model, while the second uses a gradient-based approach via the adjoint mode of the numerical model. We first apply these methods to a ‘synthetic’ experiment, then to the assimilation of real data; in each experiment we compare the results from each method and their respective computational cost. We further find that the use of the estimated Manning coefficients also reduces the model-observation misfit when tested within an independent numerical model, TELEMAC-2D, indicating that the calibration procedure has identified non model-specific and physically meaningful parameters.
更多查看译文
关键词
friction parameter estimation,bayesian inference,gradient-based
AI 理解论文
溯源树
样例
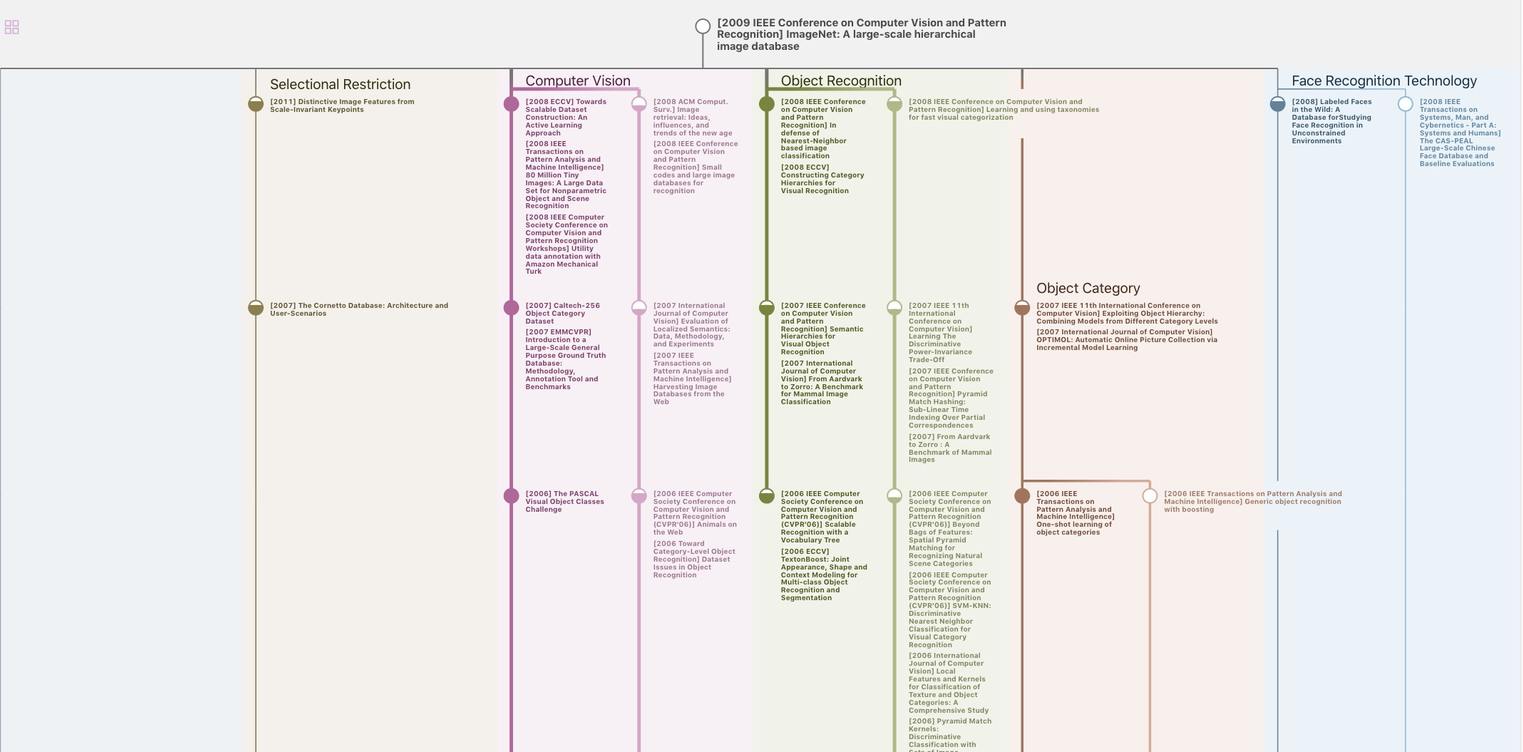
生成溯源树,研究论文发展脉络
Chat Paper
正在生成论文摘要