A Transformer-Based Approach to Multilingual Fake News Detection in Low-Resource Languages
ACM TRANSACTIONS ON ASIAN AND LOW-RESOURCE LANGUAGE INFORMATION PROCESSING(2022)
摘要
Fake news classification is one of the most interesting problems that has attracted huge attention to the researchers of artificial intelligence, natural language processing, and machine learning (ML). Most of the current works on fake news detection are in the English language, and hence this has limited its widespread usability, especially outside the English literate population. Although there has been a growth in multilingual web content, fake news classification in low-resource languages is still a challenge due to the non-availability of an annotated corpus and tools. This article proposes an effective neural model based on the multilingual Bidirectional Encoder Representations from Transformer (BERT) for domain-agnostic multilingual fake news classification. Large varieties of experiments, including language-specific and domain-specific settings, are conducted. The proposed model achieves high accuracy in domain-specific and domain-agnostic experiments, and it also outperforms the current state-of-the-art models. We perform experiments on zero-shot settings to assess the effectiveness of language-agnostic feature transfer across different languages, showing encouraging results. Cross-domain transfer experiments are also performed to assess language-independent feature transfer of the model. We also offer a multilingual multidomain fake news detection dataset of five languages and seven different domains that could be useful for the research and development in resource-scarce scenarios.
更多查看译文
关键词
Fake news detection,low-resource languages,multilingual,Hindi,Swahili,Indonesian,Vietnamese
AI 理解论文
溯源树
样例
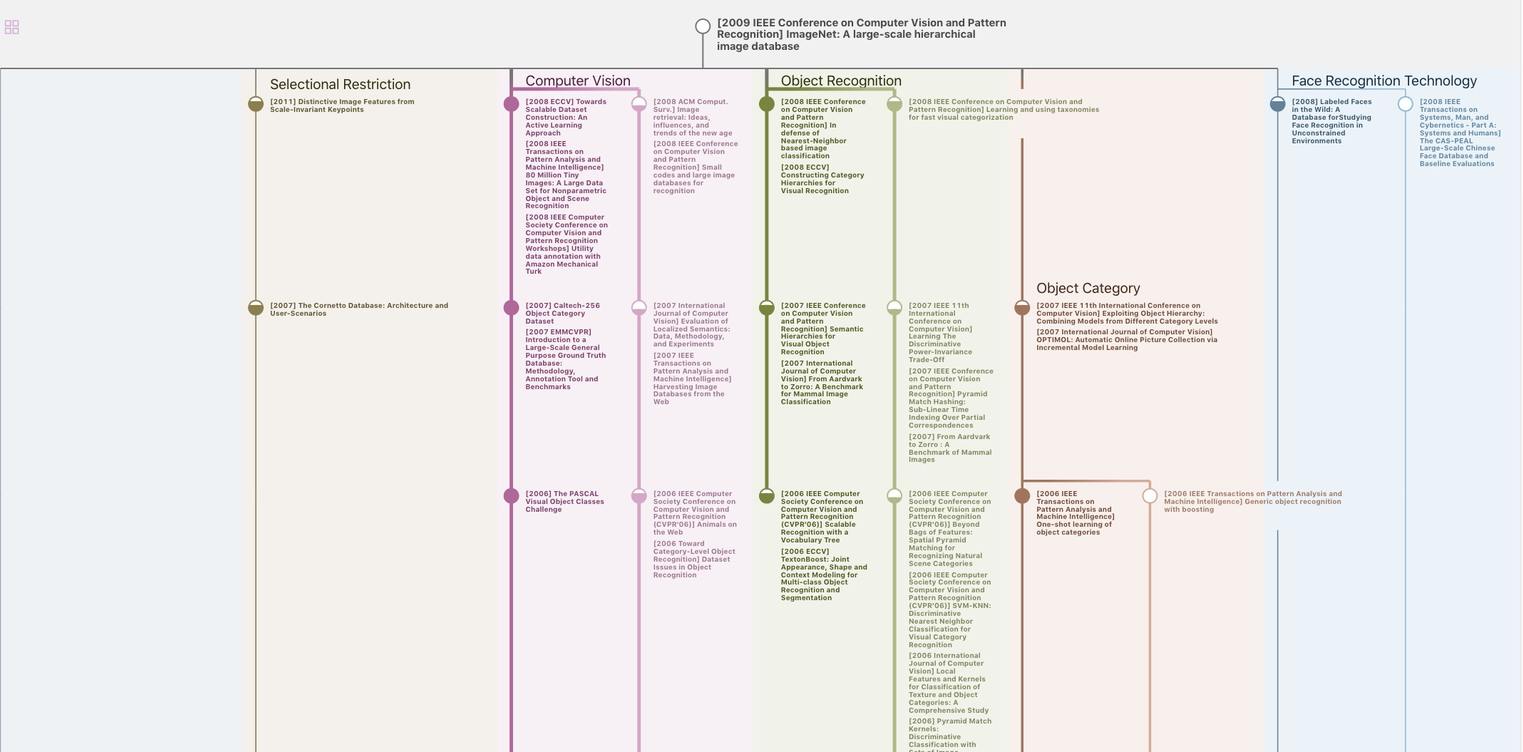
生成溯源树,研究论文发展脉络
Chat Paper
正在生成论文摘要