Multiblock-Networks: A Neural Network Analog to Component Based Methods for Multi-Source Data
ArXiv(2021)
摘要
Training predictive models on datasets from multiple sources is a common, yet challenging setup in applied machine learning. Even though model interpretation has attracted more attention in recent years, many modeling approaches still focus mainly on performance. To further improve the interpretability of machine learning models, we suggest the adoption of concepts and tools from the well-established framework of component based multiblock analysis, also known as chemometrics. Nevertheless, artificial neural networks provide greater flexibility in model architecture and thus, often deliver superior predictive performance. In this study, we propose a setup to transfer the concepts of component based statistical models, including multiblock variants of principal component regression and partial least squares regression, to neural network architectures. Thereby, we combine the flexibility of neural networks with the concepts for interpreting block relevance in multiblock methods. In two use cases we demonstrate how the concept can be implemented in practice, and compare it to both common feed-forward neural networks without blocks, as well as statistical component based multiblock methods. Our results underline that multiblock networks allow for basic model interpretation while matching the performance of ordinary feed-forward neural networks.
更多查看译文
关键词
neural multiblock-networks analog,component based methods,neural multiblock-networks,multi-source
AI 理解论文
溯源树
样例
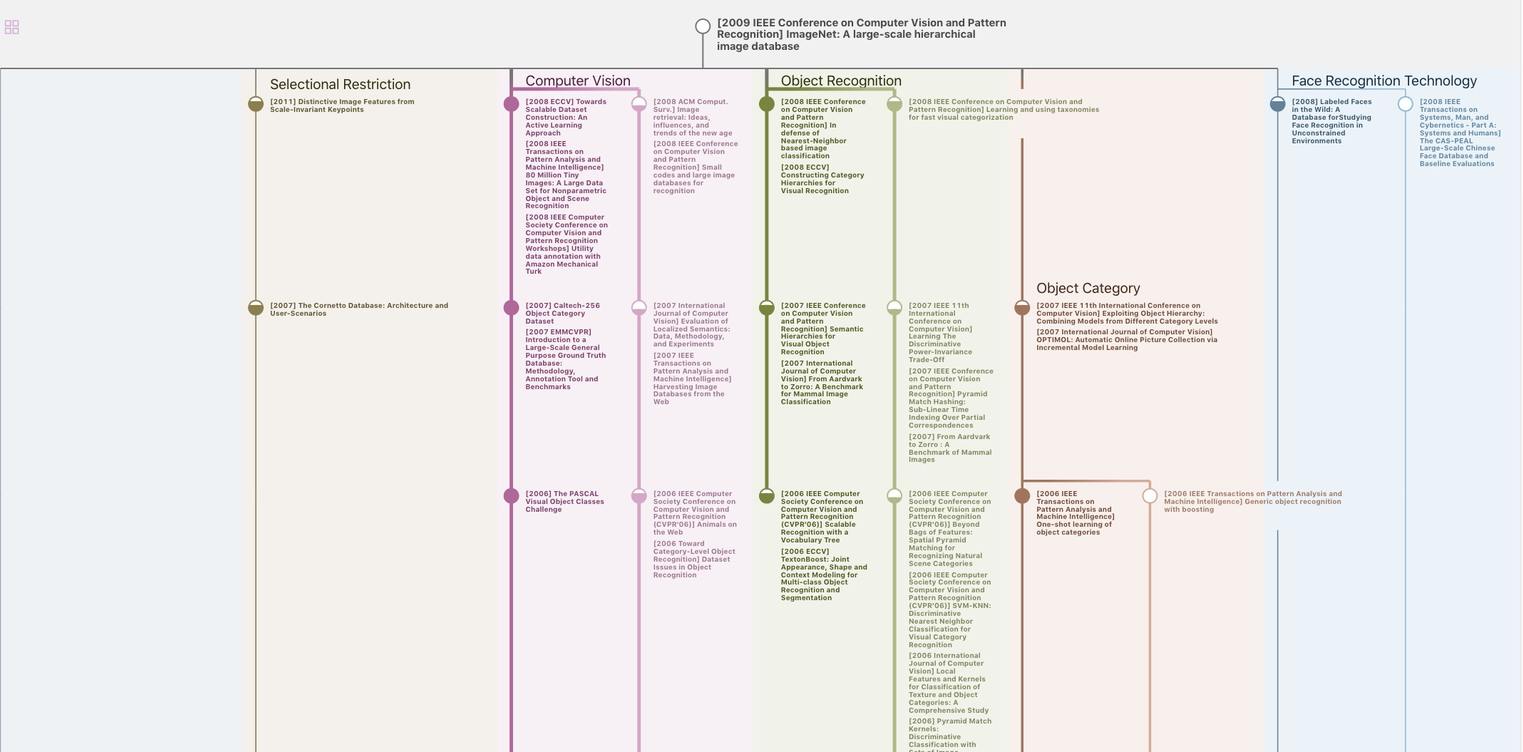
生成溯源树,研究论文发展脉络
Chat Paper
正在生成论文摘要