Mean squared error, deconstructed
Journal of Advances in Modeling Earth Systems(2021)
摘要
As science becomes increasingly cross-disciplinary and scientific models become increasingly cross-coupled, standardized practices of model evaluation are more important than ever. For normally distributed data, mean squared error (MSE) is ideal as an objective measure of model performance, but it gives little insight into what aspects of model performance are "good" or "bad." This apparent weakness has led to a myriad of specialized error metrics, which are sometimes aggregated to form a composite score. Such scores are inherently subjective, however, and while their components may be interpretable, the composite itself is not. We contend that, a better approach to model benchmarking and interpretation is to decompose MSE into interpretable components. To demonstrate the versatility of this approach, we outline some fundamental types of decomposition and apply them to predictions at 1,021 streamgages across the conterminous United States from three streamflow models. Through this demonstration, we hope to show that each component in a decomposition represents a distinct concept, like "season" or "variability," and that simple decompositions can be combined to represent more complex concepts, like "seasonal variability," creating an expressive language through which to interrogate models and data.
更多查看译文
关键词
mean squared error, decomposition, bias, variance, model benchmarking
AI 理解论文
溯源树
样例
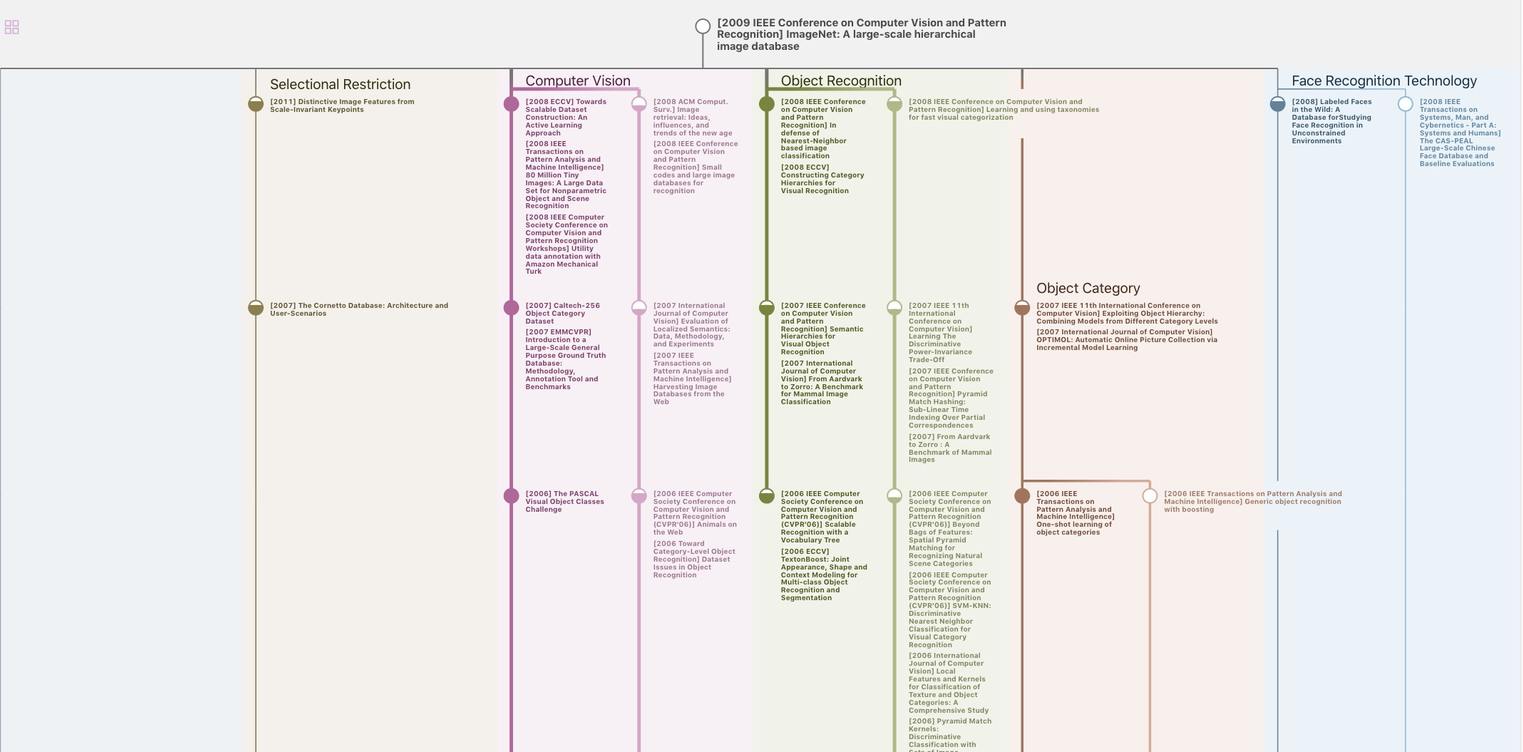
生成溯源树,研究论文发展脉络
Chat Paper
正在生成论文摘要