Knowledge Tracing Models’ Predictive Performance when a Student Starts a Skill
semanticscholar
摘要
Previous studies on the accuracy of knowledge tracing models have typically considered the performance of all student actions. However, this practice ignores the difference between students’ initial and later attempts on the same skill. To be effective for uses such as mastery learning, a knowledge tracing model should be able to infer student knowledge and performance on a skill after the student has practiced that skill a few times. However, a model’s initial performance prediction – on the first attempt at a new skill – has a different meaning. It indicates how successful a model is at inferring student performance on a skill from both their performance on other skills and from the difficulty and other properties of the first item the student encounters. As such, it may be relevant to differentiate prediction in these two contexts when evaluating a knowledge tracing model. In this paper, we describe model performance at a more granular level and examine the consistency of model performance across the number of student instances on a given skill. Results from our research show that much of the difference in performance between classic algorithms such as BKT (Bayesian Knowledge Tracing) and PFA (Performance Factors Analysis), as compared to a modern algorithm such as DKVMN (Dynamic Key-Value Memory Networks), comes down to the first attempts of a skill. Model performance is much more comparable by the time the student reaches their third attempt at a skill. Thus, while there are many benefits to using contemporary knowledge tracing algorithms, they may not be as different as previously thought in terms of mastery learning.
更多查看译文
AI 理解论文
溯源树
样例
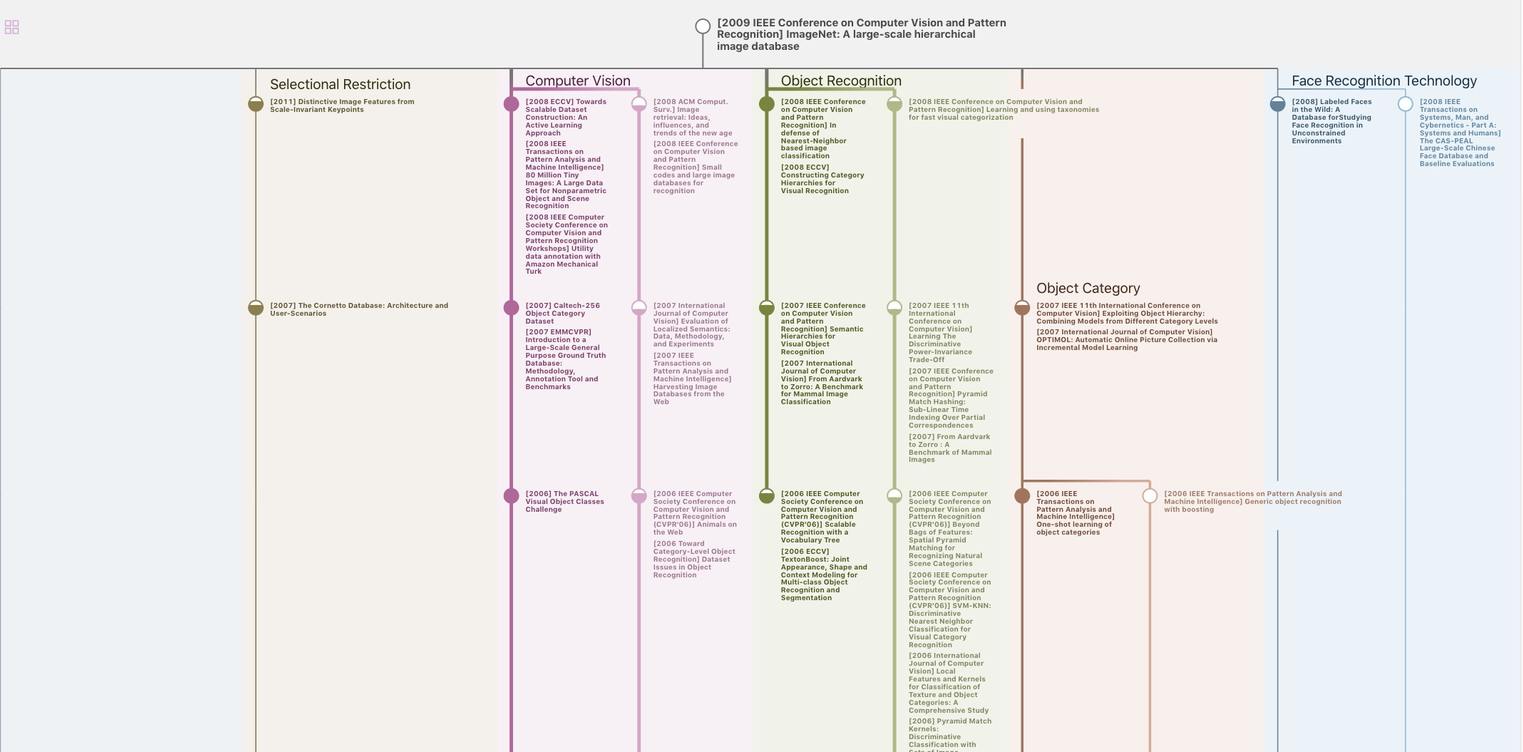
生成溯源树,研究论文发展脉络
Chat Paper
正在生成论文摘要