Deep Learning-Based Magnetic Resonance Imaging Lung Segmentation and Volumetric Marker Extraction in Preterm Infants
medRxiv(2021)
摘要
Objective and Impact Statement: We apply a deep learning (DL) segmentation method and automate the extraction of imaging markers for neonatal lung structure using magnetic resonance imaging (MRI) in order to inform clinical care with robust and quantifiable information about the neonatal lung. Introduction: Quantification of lung structural information in a standardized fashion is crucial to inform diagnostic processes that enable personalized treatment and monitoring strategies. Increased efficiency and accuracy in image quantification is especially needed in prematurely born infants, for whom long-term survival is critically determined by acute and chronic pulmonary complications, currently diagnosed based on clinical criteria due to the lack of routinely applicable diagnostic tools. Methods: We prospectively enrolled 107 premature infants in two clinical centers with and without chronic lung disease, i.e., Bronchopulmonary Dysplasia (BPD) to perform quiet-breathing lung MRI. An ensemble of deep convolutional neural networks was developed to perform lung segmentation, with a subsequent reconstruction of the 3-dimensional lung and computation of MRI volumetric measurements and compared to the standard manual segmentation. Results: The DL model successfully annotates lung segments with a volumetric dice score of 0.908 (Site 1) and 0.880 (Site 2), thereby reaching expert-level performance while demonstrating high transferability between study sites and robustness towards technical (low spatial resolution, movement artifacts) and disease conditions. Estimated lung volumes correlated with infant lung function testing measures and enabled the separation of neonates with and without BPD. Conclusion: Our work demonstrates the potential of AI-supported MRI measures to perform monitoring of neonatal lung development and characterization of respiratory diseases in this high-risk patient cohort.
更多查看译文
AI 理解论文
溯源树
样例
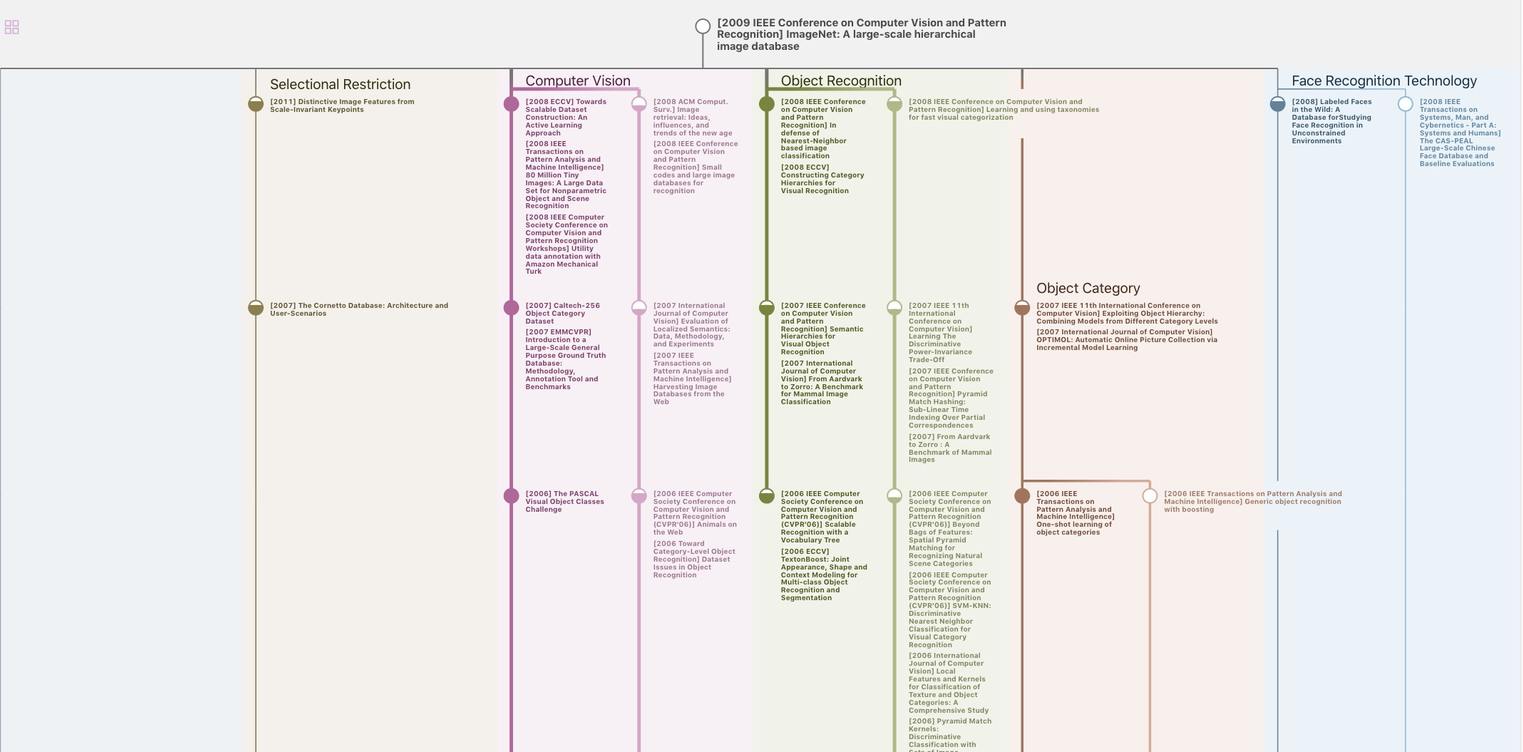
生成溯源树,研究论文发展脉络
Chat Paper
正在生成论文摘要