An overview of penalised regression methods for informing the selection of predictive markers
semanticscholar(2020)
摘要
Background: Penalised regression methods are a useful atheoretical approach for identifying key predictive indicators when one’s initial list of indicators is substantial, a process which may aid in informing population health surveillance. The purpose of this study was to examine the predictive performance and feature (i.e., variable) selection capability of common penalised regression methods (LASSO, adaptive LASSO, and elastic-net), compared with traditional logistic regression and forward selection methods. Design: Data were drawn from the Australian Temperament Project, a longitudinal cohort study beginning in 1983. The analytic sample consisted of 1,292 (707 women) participants. A total of 102 adolescent psychosocial and contextual indicators were available to predict young adult daily smoking. Findings: Penalised regression methods showed small improvements in predictive performance over logistic regression and forward selection. However, no single penalised regression model outperformed the others. Elastic-net models selected more indicators than either LASSO or adaptive LASSO. Additionally, more regularised models included fewer indicators, yet had comparable predictive performance. Forward selection methods dismissed many indicators identified as important in the penalised regression models. Conclusions: Although overall predictive accuracy was only marginally better with penalised regression method, benefits were most clear in their capacity to select a manageable subset of indicators. Preference to competing penalised regression methods may therefore be guided by feature selection capability, and thus interpretative considerations, rather than predictive performance alone.
更多查看译文
AI 理解论文
溯源树
样例
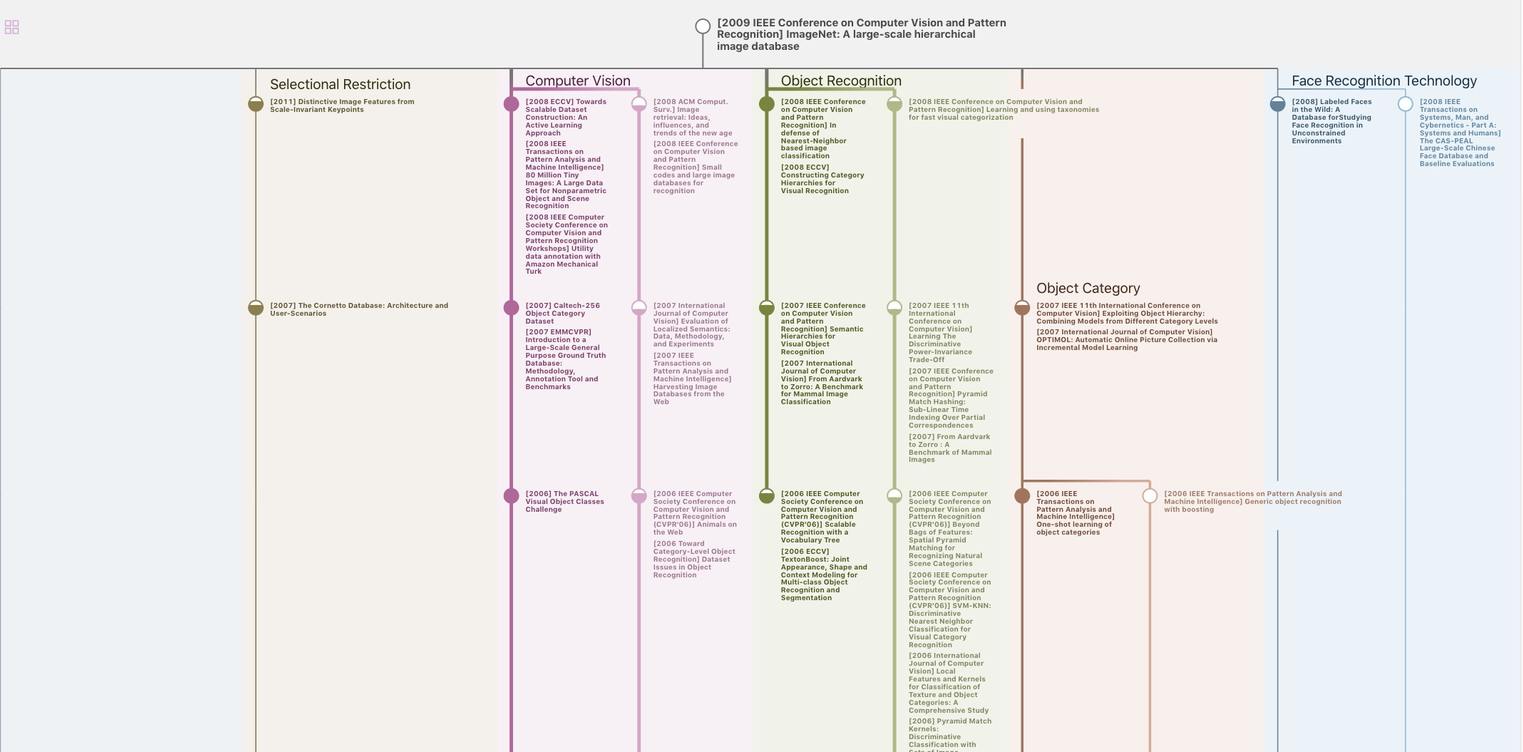
生成溯源树,研究论文发展脉络
Chat Paper
正在生成论文摘要