Semi-Explicit MMoE via Heterogeneous Multi-Task Learning for Ranking Relevance
semanticscholar(2021)
摘要
Relevance and attractiveness are two important attributes of search ranking results. While these two attributes are well-aligned for simple (query, result) pairs, they start to diverge for more nuanced, sophisticated pairs, especially when click-bait elements enter the result presentation. In this work, we explore how to jointly optimize both objectives in a state of the art ensemble framework, the multi-gate mixture of experts (MMoE) model, with explicit expert choice for different objectives. We also compare with less explicit expert gating mechanism. In order to adequately quantify the model improvements, we introduce the notion of bi-metric linear AUC that takes into account both relevance and user preference metrics under a one-parameter family of model scores, which generalizes the usual ROC or Precision/Recall AUC. We argue that these fine-grained metrics are better aligned with typical search engine business requirements. Due to the scarcity of relevance labels, we take a distillation approach, relying on state of the art NLP models such as BERT to produce high quality relevance predictions as labels. To differentiate among multiple degrees of relevance, we experiment with several extensions of cross entropy losses in order to capture the linear ordering of the relevance labels as well as their multi-categorical nature. The experimental results shows the new semi-explicit MMOE model via heterogeneous task learning often achieve the best performance. Finally, we successfully push the newly proposed model into a real-world online e-commerce ∗Both authors contributed equally to this research. †Corresponding author. Permission to make digital or hard copies of all or part of this work for personal or classroom use is granted without fee provided that copies are not made or distributed for profit or commercial advantage and that copies bear this notice and the full citation on the first page. Copyrights for components of this work owned by others than ACM must be honored. Abstracting with credit is permitted. To copy otherwise, or republish, to post on servers or to redistribute to lists, requires prior specific permission and/or a fee. Request permissions from permissions@acm.org. SIGKDD ’21, August 14–18, 2021, Virtual © 2021 Association for Computing Machinery. ACM ISBN 978-x-xxxx-xxxx-x/YY/MM. . . $15.00 https://doi.org/10.1145/nnnnnnn.nnnnnnn search system. We find this model create more business value for the company by helping the user find the item he or she wants to buy more quickly. The source code of our work is publicly available at https://github.com/user8831222/HMMoE.
更多查看译文
AI 理解论文
溯源树
样例
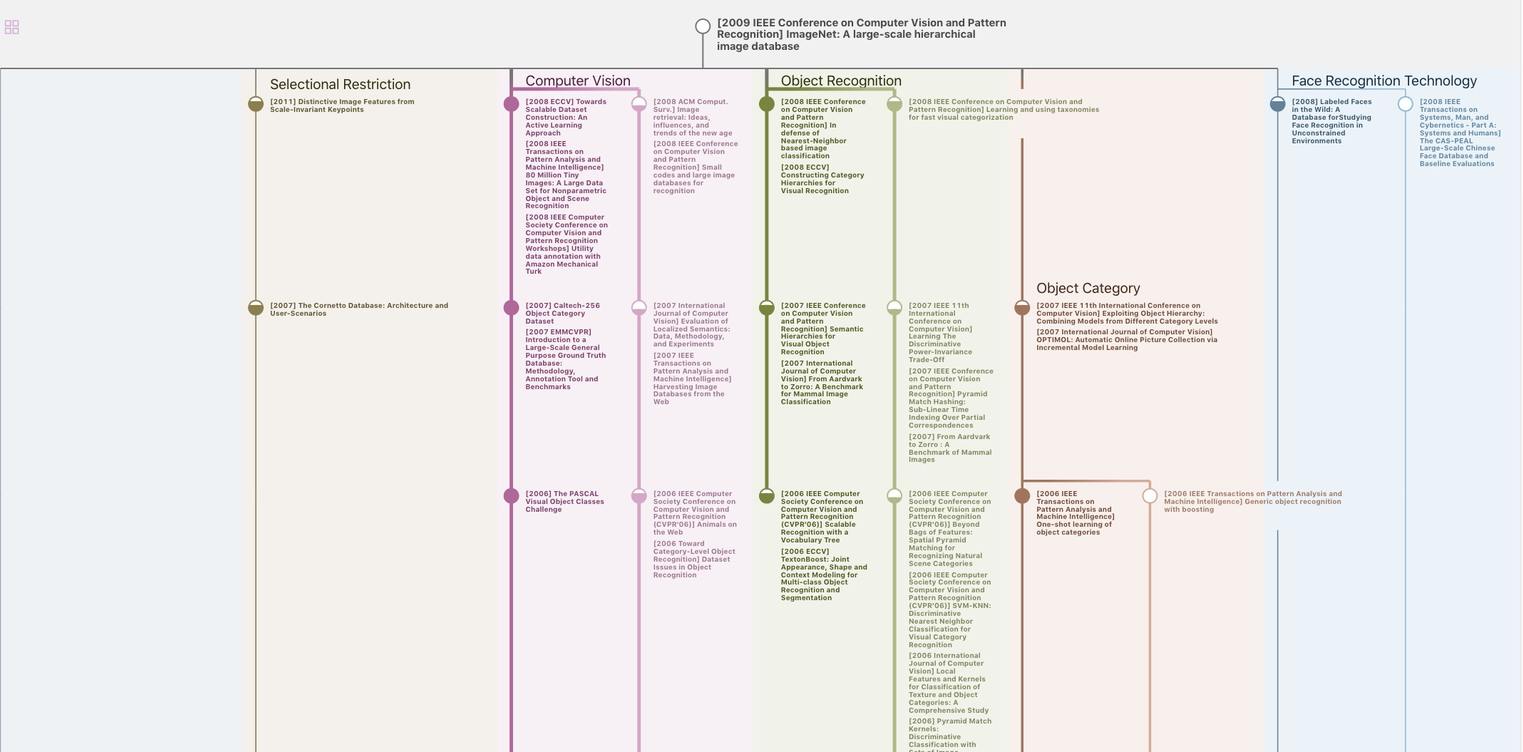
生成溯源树,研究论文发展脉络
Chat Paper
正在生成论文摘要