Toward Automated Machine Learning in Vibrational Spectroscopy: Use and Settings of Genetic Algorithms for Pre-Processing and Regression Optimization
Chemometrics and intelligent laboratory systems(2021)
摘要
Vibrational spectroscopy has become a valuable tool in many fields as it provides a molecular signature with a non-destructive measurement. Identification and prediction performance of the technique greatly depend on pre-processing steps used to remove unwanted sources of variability, especially for biological matter. However, finding the right combination of pre-processing methods (smoothing, baseline correction and/or normalization) is not a trivial task and usually depends on the operator habits. As testing all possible pre-processing sequences is time consuming, genetic algorithms (GAs) were put forward as a way to quickly find a relatively good sequence. We present here a GA that additionally optimizes the regression model, making the whole data analysis process automated, paving the way to automated machine learning. To make the best of GAs, we determined the optimal GA parameters, based on three datasets of different vibrational spectroscopy modalities (Raman or IR spectra from food industry or biological samples). They depended on the desired quality of the solution, but hardly on the dataset itself, meaning they could be used on new data without further tuning. Our method compares positively with random search, ant colony optimization and tree-structured Parzen Estimator, commonly used in machine learning for tuning hyperparameters. In conclusion, we provide a GA adapted to the simultaneous selection of pre-processing and regression of vibrational spectra.
更多查看译文
关键词
Raman spectroscopy,Near infrared spectroscopy,Chemometrics,Genetic algorithm,Pre-processing,Automated machine learning
AI 理解论文
溯源树
样例
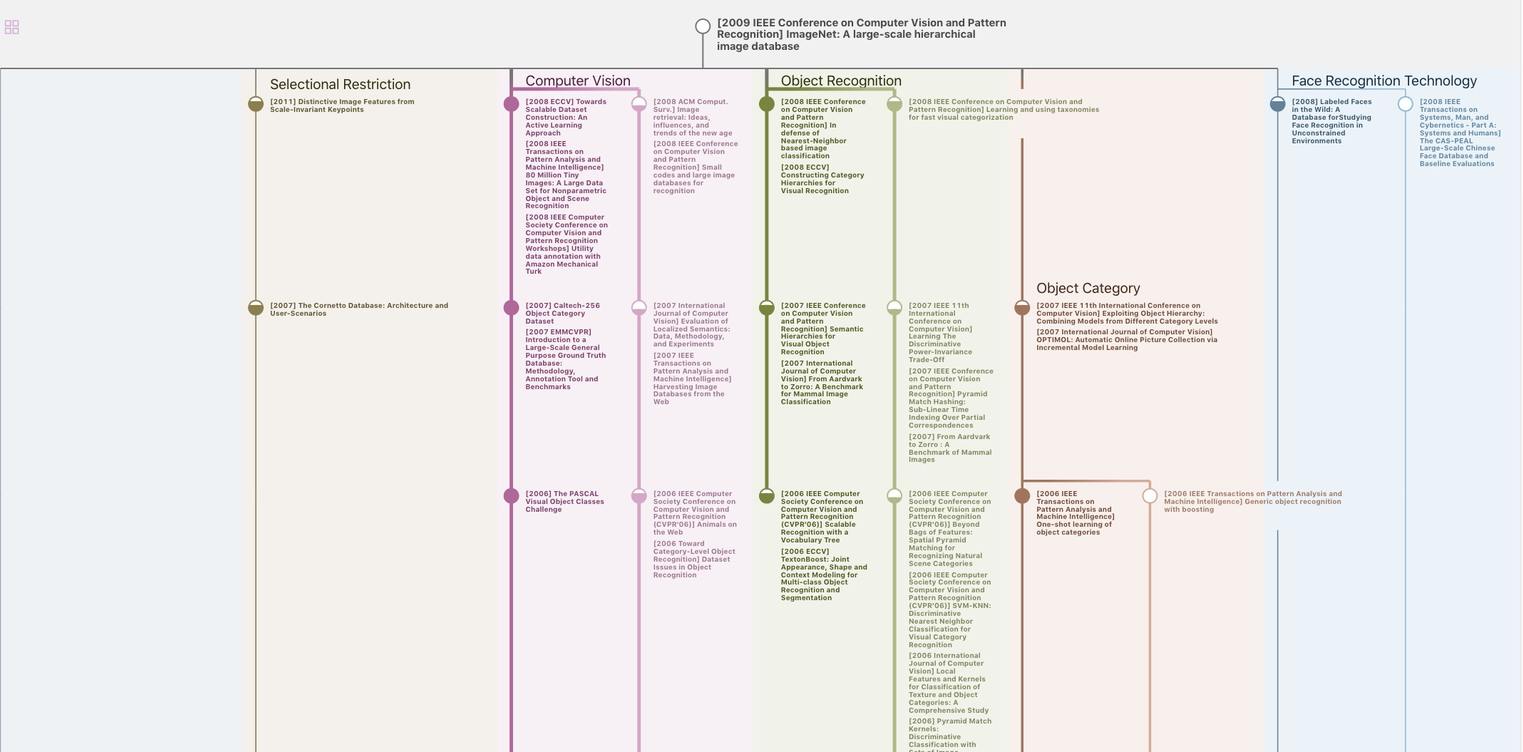
生成溯源树,研究论文发展脉络
Chat Paper
正在生成论文摘要