High-resolution snow depth prediction using Random Forest algorithm with topographic parameters: A case study in the Greiner watershed, Nunavut
HYDROLOGICAL PROCESSES(2022)
摘要
Increased surface temperatures (0.7 degrees C per decade) in the Arctic affects polar ecosystems by reducing the extent and duration of annual snow cover. Monitoring of these important ecosystems needs detailed information on snow cover properties at resolutions (<100 m) that influence ecological habitats and permafrost thaw. A machine learning method using topographic parameters with the Random Forest (RF) algorithm previously developed in alpine environments was applied over an arctic landscape for the first time. The topographic parameters used in the RF algorithm were Topographic Position Index (TPI) and up-wind slope index (S-x), which were estimated from the freely available Arctic DEM at 2 m resolution. Addition of an ecotype parameter (proxy for vegetation height) showed minimal predictive improvement. Using RF, snow depth distributions were predicted from topographic parameters with a root mean square error = 8 cm (23%) (R-2 = 0.79) at 10 m resolution for an arctic watershed (1500 km(2)) in western Nunavut, Canada.
更多查看译文
关键词
Arctic snow, Random Forest, Snow depth
AI 理解论文
溯源树
样例
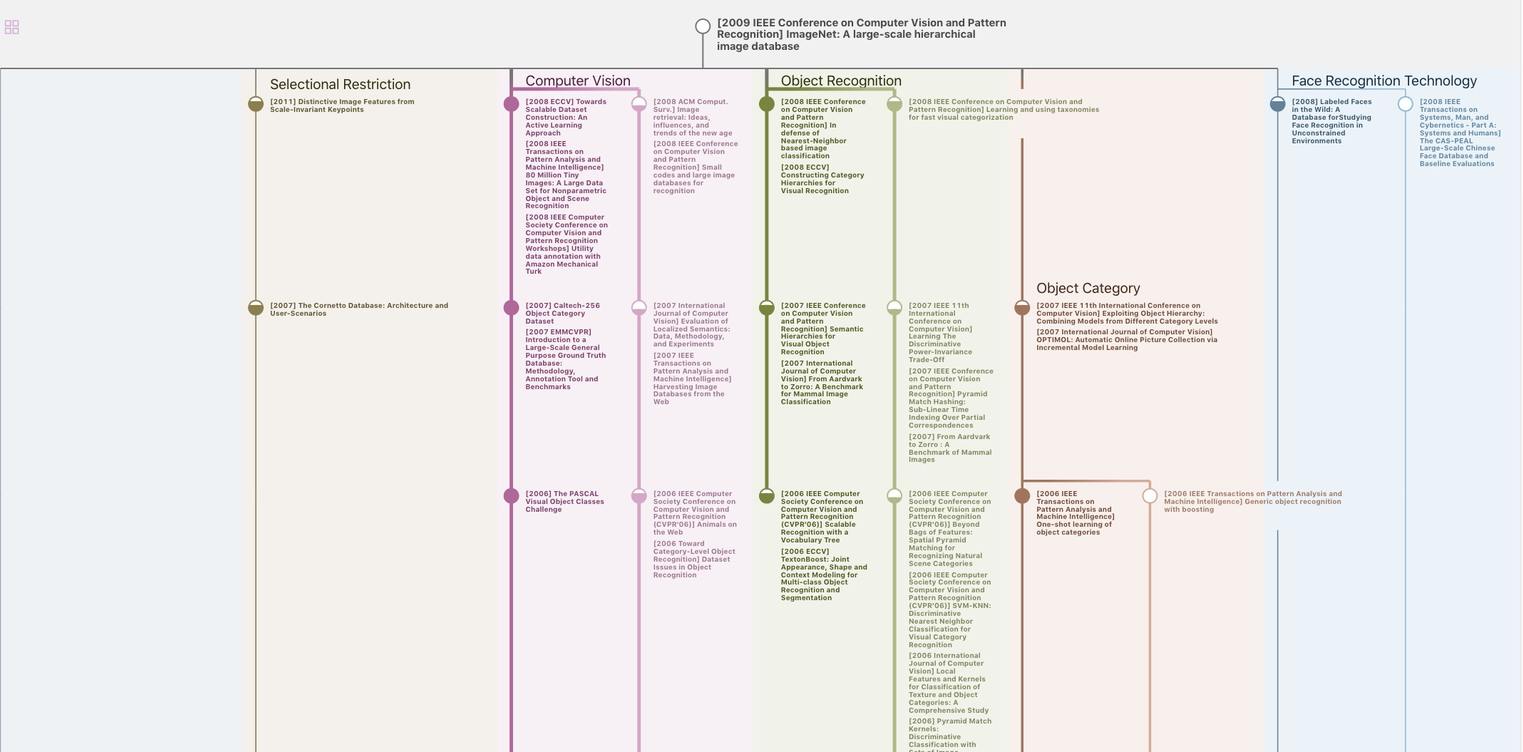
生成溯源树,研究论文发展脉络
Chat Paper
正在生成论文摘要