DiagnosisQA: A semi-automated pipeline for developing clinician validated diagnosis specific QA datasets.
medRxiv(2021)
摘要
Question answering (QA) is one of the oldest research areas of AI and Compu- national Linguistics. QA has seen significant progress with the development of state-of-the-art models and benchmark datasets over the last few years. However, pre-trained QA models perform poorly for clinical QA tasks, presumably due to the complexity of electronic healthcare data. With the digitization of healthcare data and the increasing volume of unstructured data, it is extremely important for healthcare providers to have a mechanism to query the data to find appropriate answers. Since diagnosis is central to any decision-making for the clinicians and patients, we have created a pipeline to develop diagnosis-specific QA datasets and curated a QA database for the Cerebrovascular Accident (CVA). CVA, also commonly known as Stroke, is an important and commonly occurring diagnosis amongst critically ill patients. Our method when compared to clinician validation achieved an accuracy of 0.90(with 90% CI [0.82,0.99]). Using our method, we hope to overcome the key challenges of building and validating a highly accurate QA dataset in a semiautomated manner which can help improve performance of QA models.
更多查看译文
关键词
specific diagnosisqa datasets,clinician,semi-automated
AI 理解论文
溯源树
样例
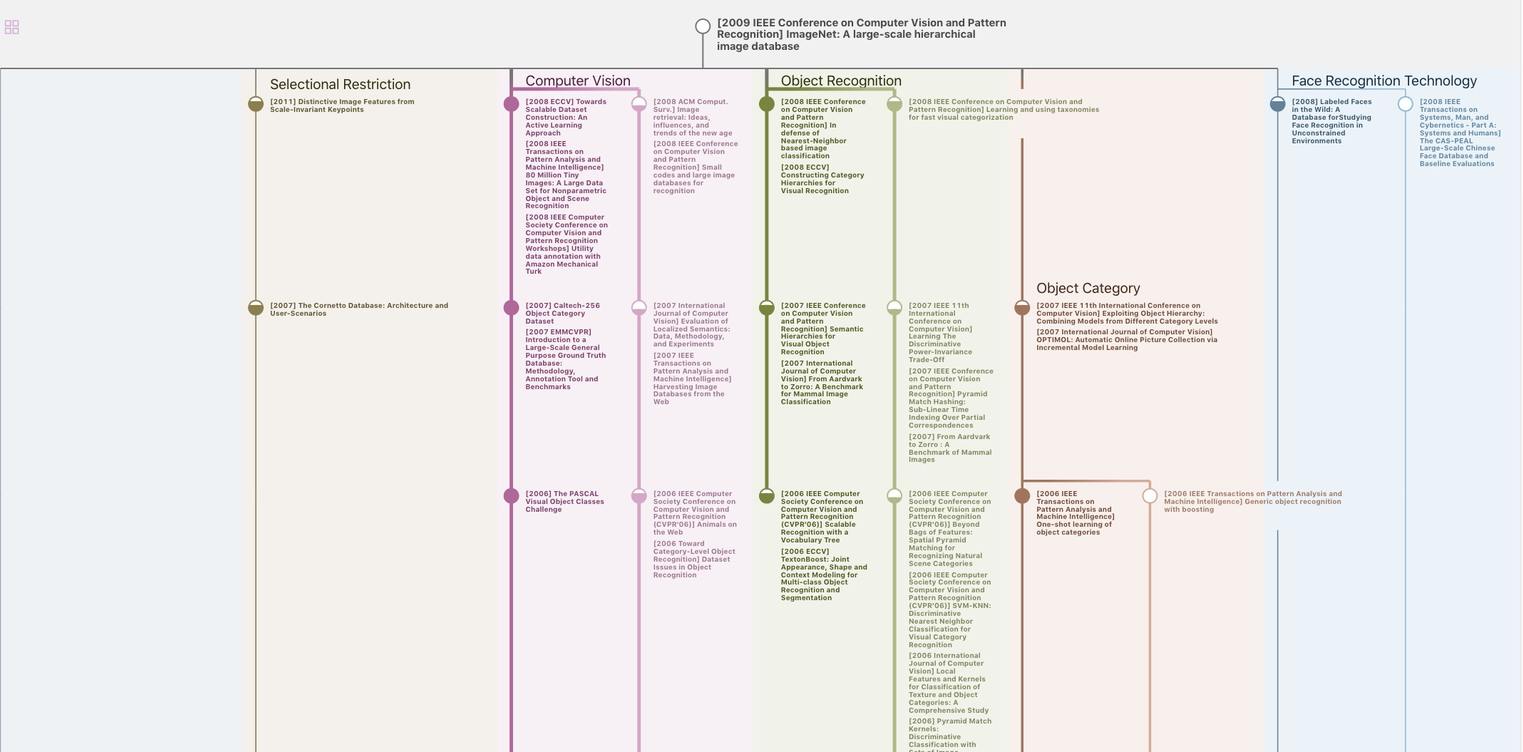
生成溯源树,研究论文发展脉络
Chat Paper
正在生成论文摘要