Deep Learning-Based Assistive Technology in Chest Radiograph Interpretation by Emergency Department Physicians: Prospective Study (Preprint)
semanticscholar(2021)
摘要
BACKGROUND
Interpretation of chest radiographs (CRs) performed by emergency department (ED) physicians is inferior to that by radiologists. Recent studies have investigated the impact of deep learning-based assistive technology on CR interpretation (DLCR), but its relevance to ED physicians remains unclear.
OBJECTIVE
This study aimed to investigate whether DLCR supports CR interpretation and clinical decision-making of ED physicians
METHODS
Seven ED physicians were used in a prospective study. CR interpretation and clinical decision-making were assessed based on 388 clinical cases, including detecting the target lesion with DLCR. Participant performance was evaluated by area under the receiver operating characteristics curve, sensitivity, specificity, and accuracy analyses; decision-making consistency was measured by kappa statistics.
RESULTS
There was a difference in performance between ED physicians working with and without DLCR (area under the receiver operating characteristics curve: 0.801, P<.001). Diagnostic sensitivity and accuracy of CR were higher for all ED physicians working with DLCR than for those working without it. The overall kappa value for decision-making consistency was 0.902 (95% CI: 0.884–0.920); concurrently, that for the experienced group was 0.956 (95% CI: 0.934–0.979) and that for the inexperienced group was 0.862 (95% CI: 0.835–0.889).
CONCLUSIONS
This study presents preliminary evidence that ED physicians using DLCR in a clinical setting perform better at CR interpretation than their counterparts who do not use this technology. DLCR use influenced clinical decision-making of inexperienced physicians more strongly than it did that of experienced physicians. These findings require prospective validation before DLCR can be recommended for use in routine clinical practice.
CLINICALTRIAL
none declared
更多查看译文
AI 理解论文
溯源树
样例
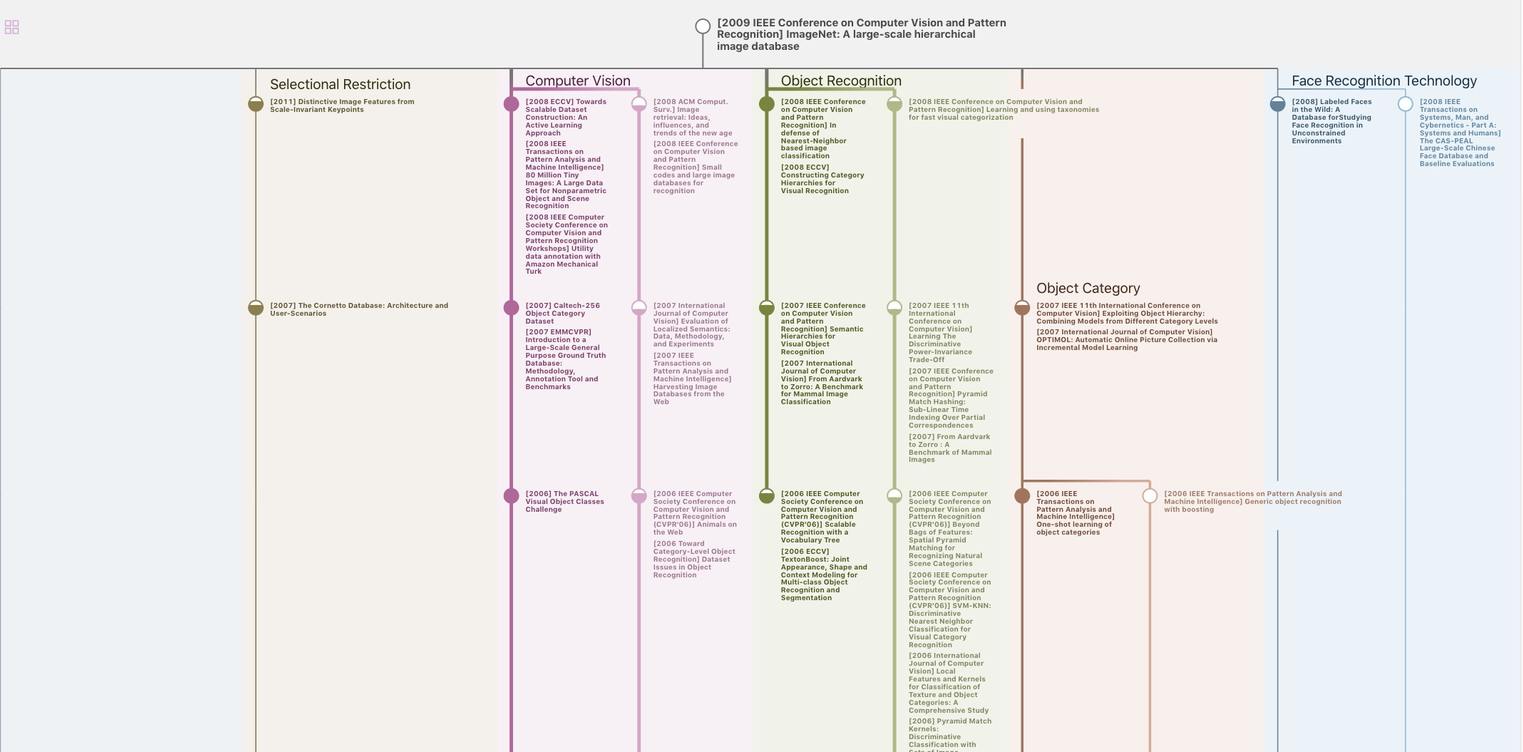
生成溯源树,研究论文发展脉络
Chat Paper
正在生成论文摘要