Use smoking contingent surveys and context to Characterize and Model Smoking Habits Tracked by Mobile Sensors in Naturalistic Environments (Preprint)
semanticscholar(2021)
摘要
BACKGROUND
There are 1.1 billion smokers worldwide and each year more than 8 million die prematurely because of cigarette smoking. Encouragingly, more than half of current smokers make a serious quit every year. Nonetheless, 90% of unaided quitters relapse within the first 4 weeks due to the lack of limited access to cost-effective and efficient smoking cessation tools in their daily life.
OBJECTIVE
This research work aims to 1)enable quantified monitoring of ambulatory smoking habits 24/7 in real life by exploiting continuous and automatic measurement techniques. 2) to identify and characterize smoking patterns with contextual signals collected in a longitudinal manner. This work also intends to provide guidance and insights on the design and deployment of technology-enabled smoking cessation applications in naturalistic environments.
METHODS
Specifically, a 4-week observational study consisting of 46 smokers was conducted in both working and personal life environments. In the study, an electric lighter, a smartphone, and an experimental application were used for tracking smoking events and acquiring concurrent contextual signals. Besides, the mobile technology was exploited to prompt smoking-continent ecological momentary assessment (EMA) surveys, sampling ambient social context. In the data analysis part, the smoking rate was assessed based on the timestamps of smoking and linked statistically to demographics, time, EMA surveys, and physiology. A multivariate model (Poisson mixed-effects model) to predict smoking rate in one-hour windows was developed to assess the contribution of each of the predictors.
RESULTS
In total, 8639 cigarettes and 1839 EMA surveys were tracked over 902 subject-days. Most smokers were found to have an inaccurate and often biased estimate of their daily smoking rate, compared to measured smoking rate. Specifically, 74%(34/46 )smokers made a more than one (on average 4.7 per day) cigarette wrong estimate, and 70%(32/46) had overestimated it. Based on the timestamp of tracked smoking events, smoking rates were visualized at different hours and were found to gradually increase and peak at 6 pm in the day. Additionally, a 1 to 2 hours shift in the smoking patterns was observed between weekdays and weekends. When moderate-and-heavy smokers were compared with light smokers, their ages (P<.05), FTND(P<.05), craving level(P<.001), enjoyment of cigarettes(P<.001), difficulty to resist smoking(P<.001), emotional valence(P<.001) and arousal(P<.001) were all found to be significantly different. In the Poisson mixed-effects model, the number of cigarettes smoked in a one-hour time window is highly dependent on smokers(P <0.001) and is explained by the hour(P <0.05) and age(P <0.01) factor.
CONCLUSIONS
The results confirm that smokers lack a good awareness of their smoking habits which are diverse and highly dependent on the context. Quantified smoking patterns upgrade our understanding of smoking behaviors in the context. These results also validate the techniques for smoking habits monitoring, pave the way for the design and deployment of technology-enabled smoking cessation applications.
INTERNATIONAL REGISTERED REPORT
RR2-http://dx.doi.org/10.1136/bmjopen-2018-028284
更多查看译文
AI 理解论文
溯源树
样例
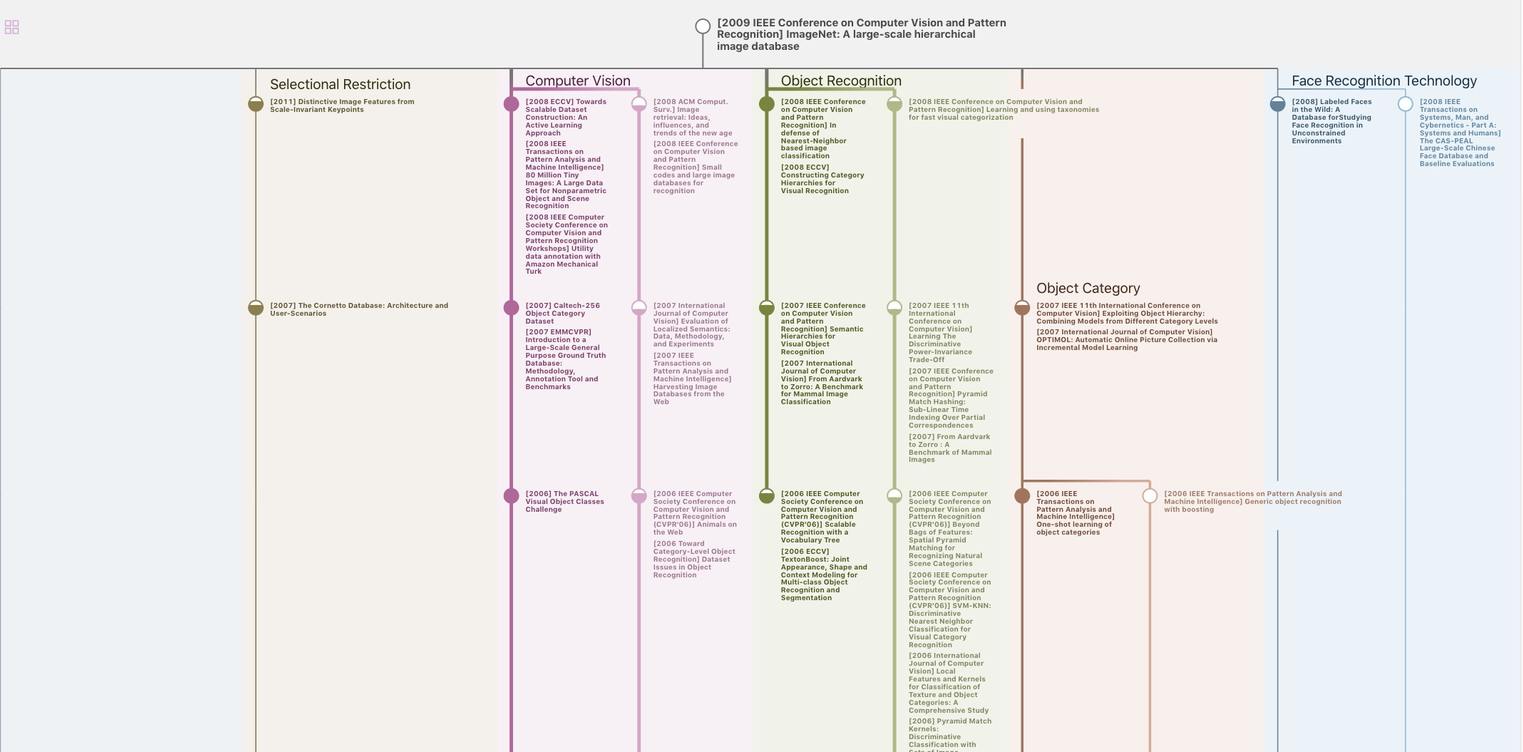
生成溯源树,研究论文发展脉络
Chat Paper
正在生成论文摘要