DPCCN: Densely-Connected Pyramid Complex Convolutional Network for Robust Speech Separation and Extraction
IEEE International Conference on Acoustics, Speech, and Signal Processing (ICASSP)(2022)
摘要
In recent years, a number of time-domain speech separation methods have been proposed. However, most of them are very sensitive to the environments and wide domain coverage tasks. In this paper, from the time-frequency domain perspective, we propose a densely-connected pyramid complex convolutional network, termed DPCCN, to improve the robustness of speech separation under complicated conditions. Furthermore, we generalize the DPCCN to target speech extraction (TSE) by integrating a new specially designed speaker encoder. Moreover, we also investigate the robustness of DPCCN to unsupervised cross-domain TSE tasks. A Mixture-Remix approach is proposed to adapt the target domain acoustic characteristics for fine-tuning the source model. We evaluate the proposed methods not only under noisy and reverberant in-domain condition, but also in clean but cross-domain conditions. Results show that for both speech separation and extraction, the DPCCN-based systems achieve significantly better performance and robustness than the currently dominating time-domain methods, especially for the cross-domain tasks. Particularly, we find that the Mixture-Remix fine-tuning with DPCCN significantly outperforms the TD-SpeakerBeam for unsupervised cross-domain TSE, with around 3.5 dB performance improvement on target domain test set, without any source domain performance degradation.
更多查看译文
关键词
DPCCN,Mixture-Remix,cross-domain,speech separation,unsupervised target speech extraction
AI 理解论文
溯源树
样例
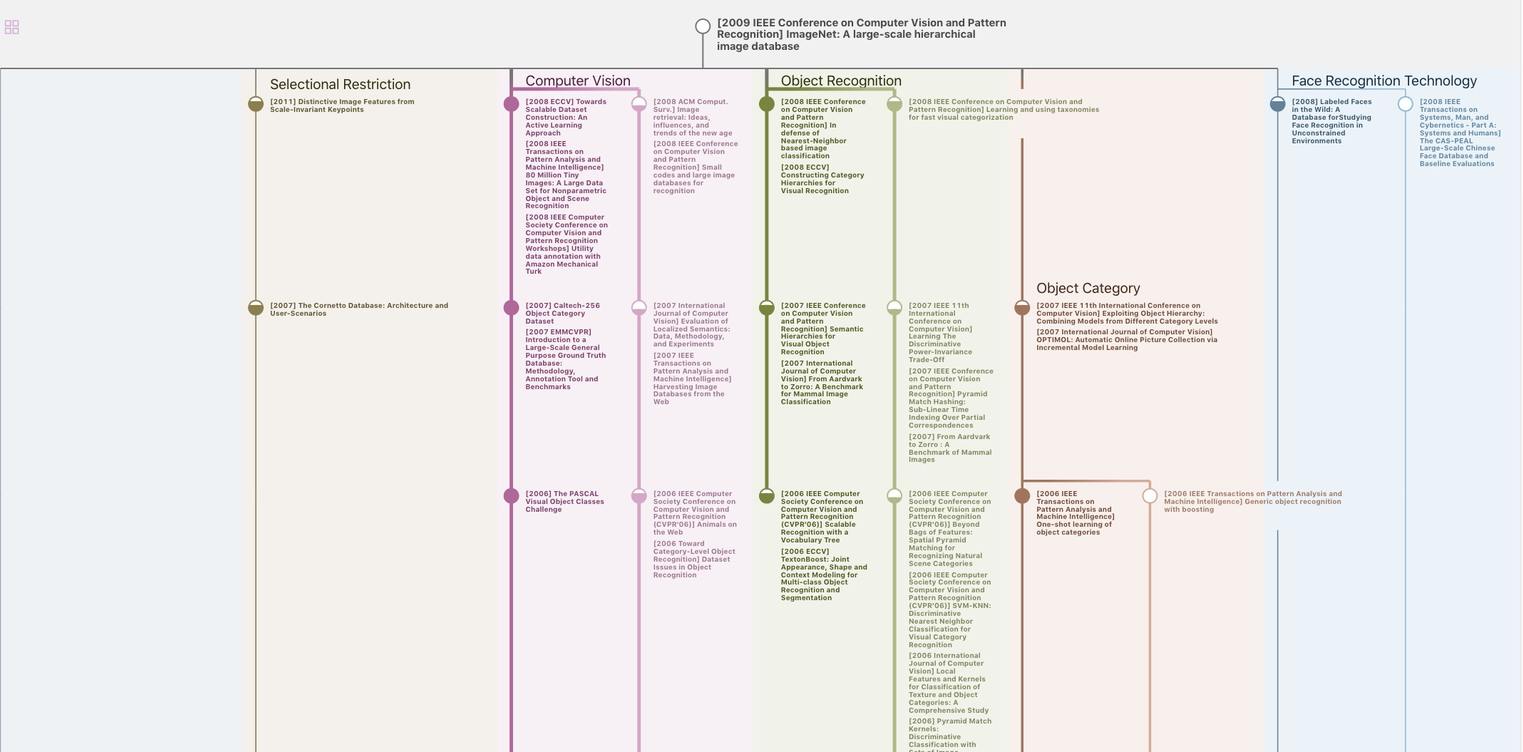
生成溯源树,研究论文发展脉络
Chat Paper
正在生成论文摘要