Adaptive Multi-Core Real- Time Scheduling Based on Reinforcement Learning.
International Conference on Control and Automation(2024)
摘要
With the transformation towards industrial intel-ligence, multi-core processors are increasingly being applied in real-time networked control systems to ensure secure exe-cution of sensing, computing and actuating tasks under time constraints. However, existing scheduling methods result in either low CPU utilization or many missed task deadlines in dynamic systems. In this paper, we propose a two-layer scheduling architecture to address this issue by fully exploring the complex dependency between real-time tasks. To be specific, the local layer determines task execution priorities considering both dependency between tasks and deadline constraints by utilizing a reinforcement learning approach. Moreover, to better utilize the parallel capabilities of multi-core processors and reduce temporal collisions, this paper minimizes the requested core count for the task set based on a greedy strategy. The global layer designs a scheduling algorithm based on the pre-empt method and provides schedulability analysis of multiple task sets. Experimental results validate the correctness of the proposed scheduling approach, and efficiency is demonstrated through comparisons with baseline method.
更多查看译文
关键词
System Dynamics,Actuator,Scheduling Algorithm,Networked Control Systems,Scheduling Method,Real-time Tasks,Service Quality,Less Than Or Equal,Search Space,Feasible Solution,Robotic Arm,Executive Order,Scheduling Scheme,Maximum Count,Task Scheduling,Admission Control,Independent Tasks,Average Reward,Core Resources,Real-time Scheduling,Visual Servoing,Online Scheduling
AI 理解论文
溯源树
样例
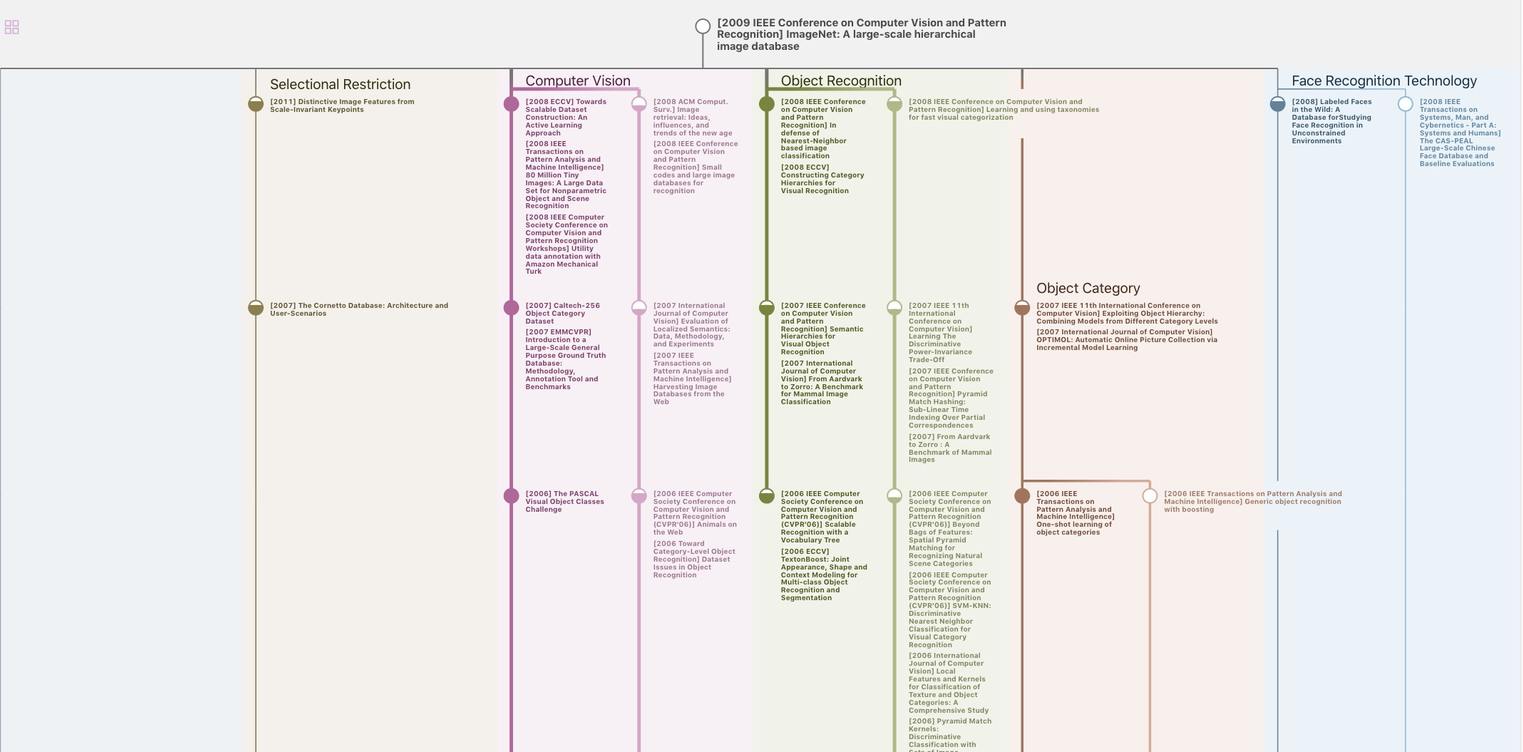
生成溯源树,研究论文发展脉络
Chat Paper
正在生成论文摘要