Risk Prediction Model of Early-Onset Preeclampsia Based on Risk Factors and Routine Laboratory Indicators.
International journal of sexual and reproductive health care(2023)
摘要
Background: Globally, 10-15% of maternal deaths are statistically attributable to preeclampsia. Compared with late-onset PE, the severity of early-onset PE remains more harmful with higher morbidity and mortality. Objective: To establish an early-onset preeclampsia prediction model by clinical characteristics, risk factors and routine laboratory indicators were investigated from pregnant women at 6 to 10 gestational weeks. Methods: The clinical characteristics, risk factors, and 38 routine laboratory indicators (6-10 weeks of gestation) including blood lipids, liver and kidney function, coagulation, blood count, and other indicators of 91 early-onset preeclampsia patients and 709 normal controls without early-onset preeclampsia from January 2010 to May 2021 in Peking University Third Hospital (PUTH) were retrospectively analyzed. A logistic regression, decision tree model, and support vector machine (SVM) model were applied for establishing prediction models, respectively. ROC curves were drawn; area under curve (AUCROC), sensitivity, and specificity were calculated and compared. Results: There were statistically significant differences in the rates of diabetes, antiphospholipid syndrome (APS), kidney disease, obstructive sleep apnea (OSAHS), primipara, history of preeclampsia, and assisted reproductive technology (ART) (p < 0.05). Among the 38 routine laboratory indicators, there were no significant differences in the levels of PLT/LYM, NEU/LYM, TT, D-Dimer, FDP, TBA, ALP, TP, ALB, GLB, UREA, Cr, P, Cystatin C, HDL-C, Apo-A1, and Lp(a) between the two groups (p > 0.05). The levels of the rest indicators were all statistically different between the two groups (p < 0.05). If only 12 risk factors of PE were analyzed with the logistic regression, decision tree model, and support vector machine (SVM), and the AUCROC were 0.78, 0.74, and 0.66, respectively, while 12 risk factors of PE and 38 routine laboratory indicators were analyzed with the logistic regression, decision tree model, and support vector machine (SVM), and the AUCROC were 0.86, 0.77, and 0.93, respectively. Conclusions: The efficacy of clinical risk factors alone in predicting early-onset preeclampsia is not high while the efficacy increased significantly when PE risk factors combined with routine laboratory indicators. The SVM model was better than logistic regression model and decision tree model in early prediction of early-onset preeclampsia incidence.
更多查看译文
关键词
early-onset preeclampsia,risk factors,routine laboratory indicators,risk prediction model,machine learning
AI 理解论文
溯源树
样例
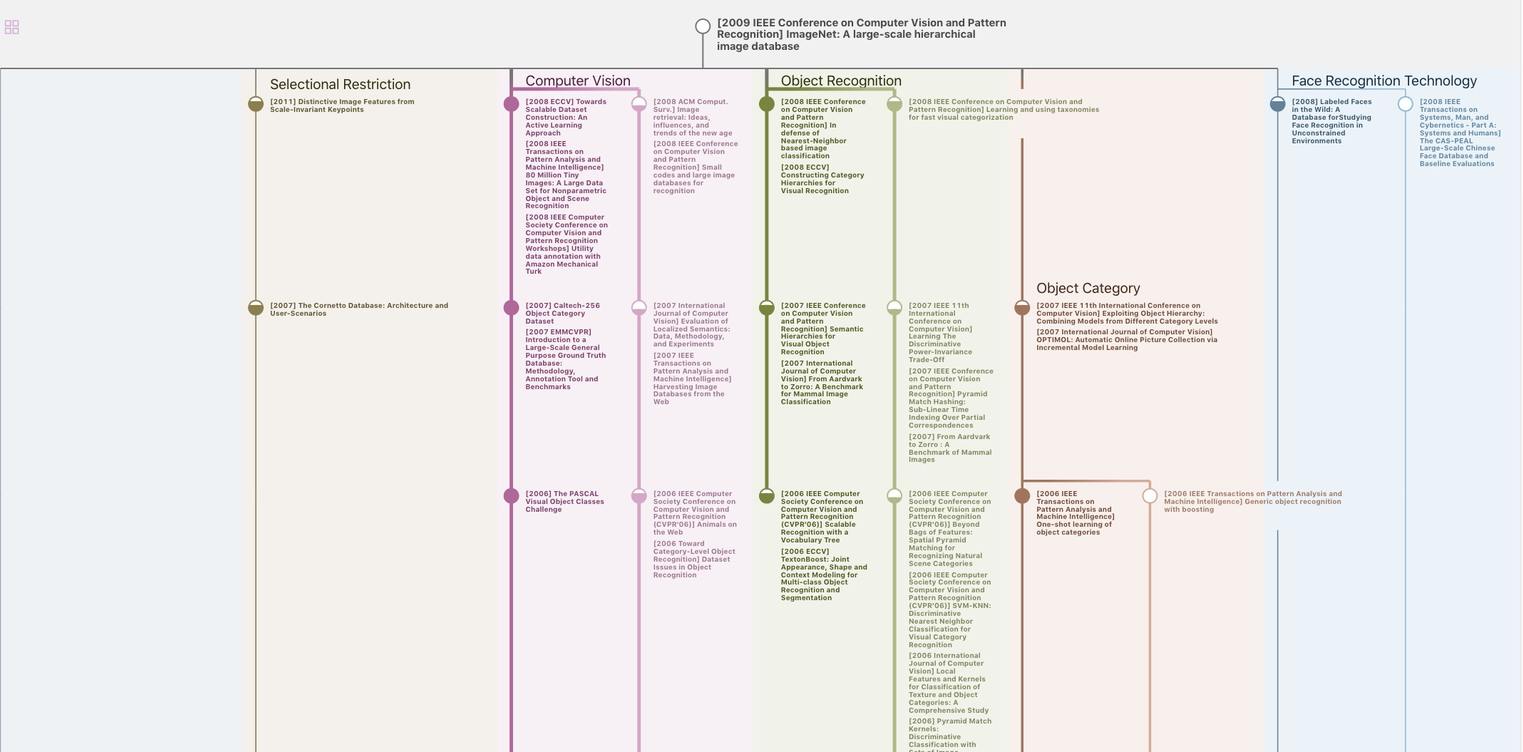
生成溯源树,研究论文发展脉络
Chat Paper
正在生成论文摘要