Predicting Stellar Masses of the First Galaxies Using Graph Neural Networks
Research Notes of the AAS(2024)
摘要
Abstract Theoretical models of galaxy formation and evolution are primarily investigated through cosmological simulations and semi-analytical models. The former method consumes O ( 10 6 ) core-hours explicitly modeling the dynamics of the galaxies, whereas the latter method only requires O ( 10 3 ) core-hours foregoing directly simulating internal structure for computational efficiency. In this work, we present a proof-of-concept machine learning regression model, using a graph neural network architecture, to predict the stellar mass of high-redshift galaxies solely from their dark matter merger trees, trained from a radiation hydrodynamics cosmological simulation of the first galaxies.
更多查看译文
AI 理解论文
溯源树
样例
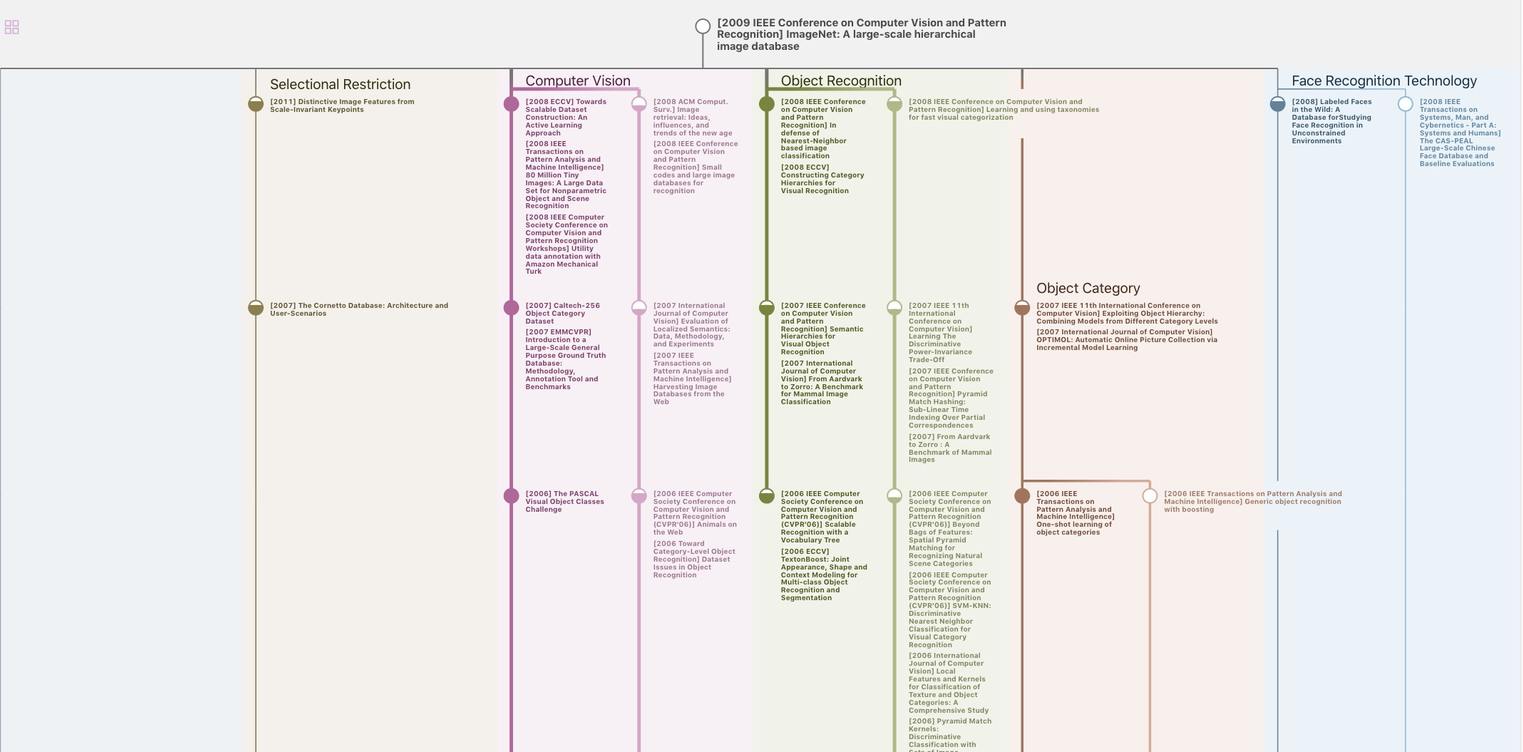
生成溯源树,研究论文发展脉络
Chat Paper
正在生成论文摘要