Machine learning enables new insights into genetic contributions to liver fat accumulation.
Cell genomics(2021)
摘要
Excess liver fat, called hepatic steatosis, is a leading risk factor for end-stage liver disease and cardiometabolic diseases but often remains undiagnosed in clinical practice because of the need for direct imaging assessments. We developed an abdominal MRI-based machine-learning algorithm to accurately estimate liver fat (correlation coefficients, 0.97-0.99) from a truth dataset of 4,511 middle-aged UK Biobank participants, enabling quantification in 32,192 additional individuals. 17% of participants had predicted liver fat levels indicative of steatosis, and liver fat could not have been reliably estimated based on clinical factors such as BMI. A genome-wide association study of common genetic variants and liver fat replicated three known associations and identified five newly associated variants in or near the , , , , and genes (p < 3 × 10). A polygenic score integrating these eight genetic variants was strongly associated with future risk of chronic liver disease (hazard ratio > 1.32 per SD score, p < 9 × 10). Rare inactivating variants in the or genes were identified in 0.8% of individuals with steatosis and conferred more than 6-fold risk (p < 2 × 10), highlighting a molecular subtype of hepatic steatosis characterized by defective secretion of apolipoprotein B-containing lipoproteins. We demonstrate that our imaging-based machine-learning model accurately estimates liver fat and may be useful in epidemiological and genetic studies of hepatic steatosis.
更多查看译文
关键词
liver fat,hepatic steatosis,machine learning,MRI,GWAS,whole-exome sequencing
AI 理解论文
溯源树
样例
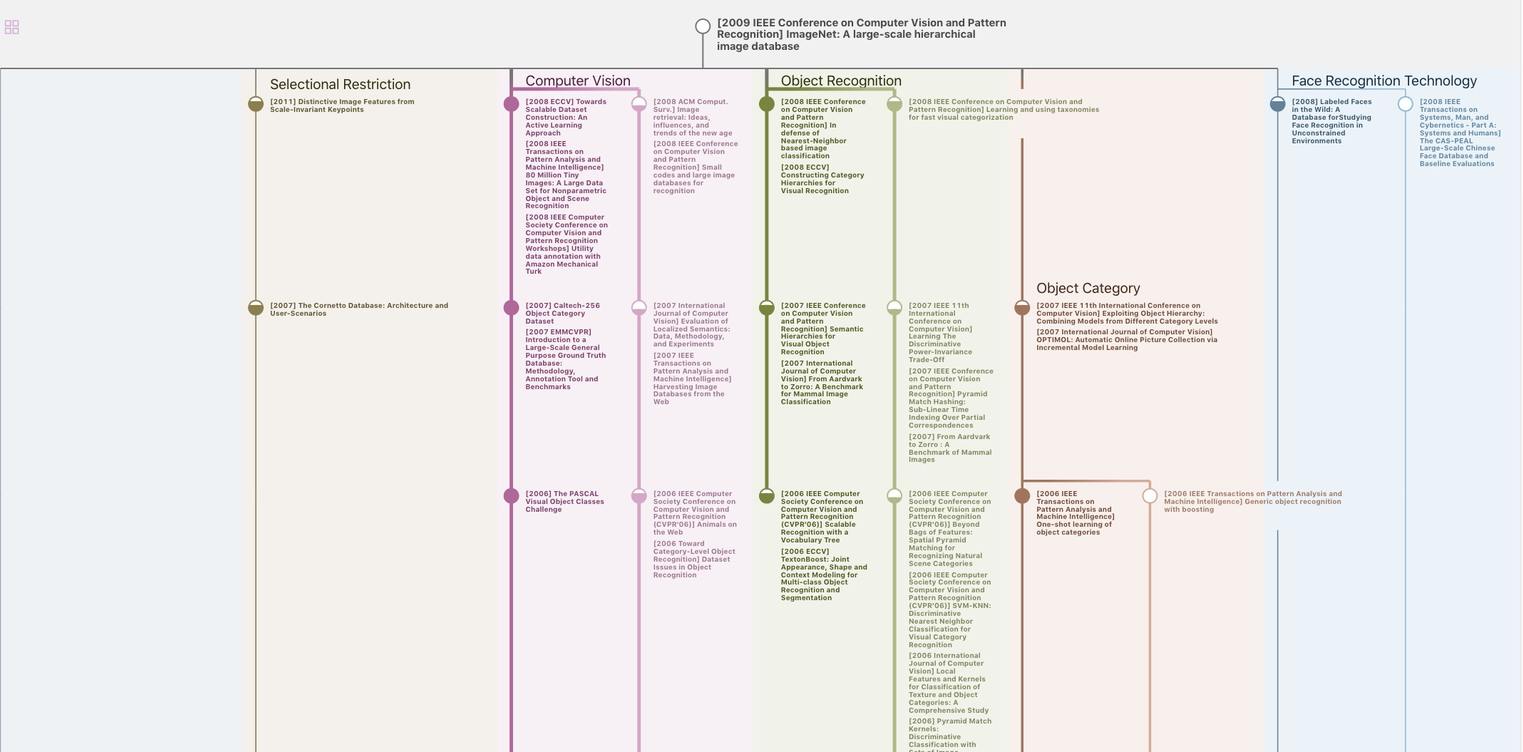
生成溯源树,研究论文发展脉络
Chat Paper
正在生成论文摘要