Dual-Path Morph-UNet for Road and Building Segmentation From Satellite Images
IEEE GEOSCIENCE AND REMOTE SENSING LETTERS(2022)
摘要
Building footprints and road network detection have gained significant attention for map preparation, humanitarian aid dissemination, disaster management, to name a few. Traditionally, morphological filters excel at extracting shape features from remotely sensed images and have been widely used in the literature. However, the structural element (SE) dimension selection impedes these classical and learning-based methods utilizing any morphological operators. To overcome this aspect, we propose a novel framework to extract road and building from remote sensing (RS) images by exploiting morphological networks. The method predominantly aims at learning an optimized SE to capture variably-sized building and road footprints. We substitute convolutions with 2-D morphological operations in the basic building blocks of the network architecture (Dual-path Morph-UNet) to manage the intricate task of optimizing the SE in addition to the actual segmentation task. The dual-path framework incorporates parallel residual and dense paths in an encoder-decoder architecture, which permits learning of higher-level feature representations with fewer parameters. Finally, we implement the proposed framework on the benchmarked Massachusetts roads and buildings dataset and demonstrate superior results than the state-of-the-art (SOTA). In addition, the proposed network consists of 10x less learnable parameters than the SOTA methods.
更多查看译文
关键词
Mathematical morphology, morphological networks, object segmentation, remote sensing (RS)
AI 理解论文
溯源树
样例
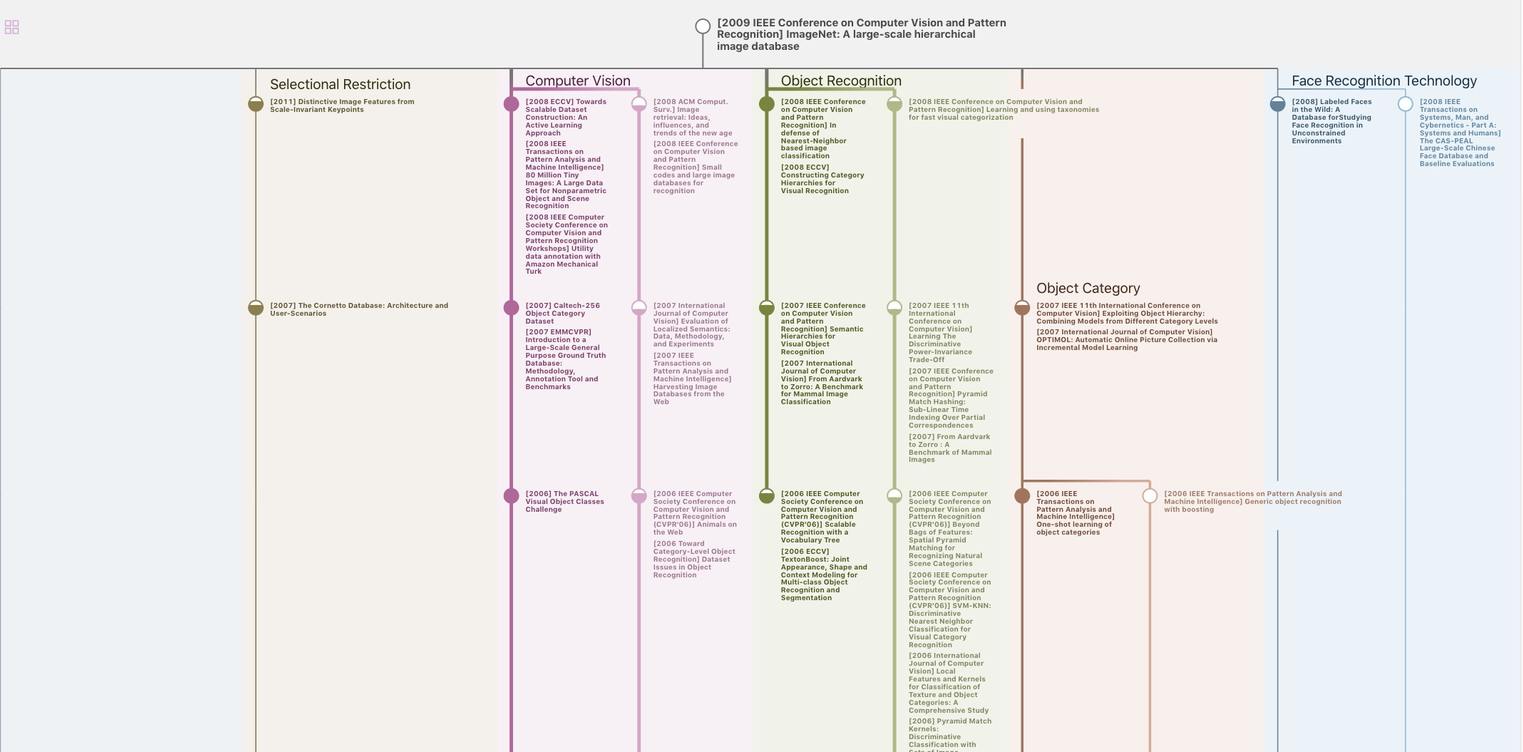
生成溯源树,研究论文发展脉络
Chat Paper
正在生成论文摘要